Harnessing deep learning for forecasting fire-burning locations and unveiling PM2.5 emissions
MODELING EARTH SYSTEMS AND ENVIRONMENT(2024)
摘要
Climate change and human activity have increased fires in India. Fine particulate matter (PM2.5) is released into the atmosphere by stubble burning in Punjab and Haryana and forest fires in the north-eastern and central areas of the country. Accurate short-term PM2.5 estimates are essential to protect human health and reduce acute air pollution. However, global air quality forecasting methods grapple with a persistent assumption of fire emissions. They use near-real-time fire emissions throughout the prediction cycle. Air quality forecasts are prone to inaccuracies and biases due to fire emissions' dynamic nature. We employ spatiotemporal deep learning techniques, specifically ConvLSTM and ConvGRU, to forecast fire emission locations up to three days in advance. Through our evaluation, we find that ConvLSTM outperforms ConvGRU in terms of prediction accuracy and performance. The chosen model provides a very good correlation coefficient (approximate to 0.8) for the 1st day forecast and a moderate value (0.5-0.55) for subsequent 2nd and 3rd days forecasts. The predictors NDVI, temperature, wind, surface pressure, and total cloud cover are included to our model training to improve these correlations. In Punjab-Haryana, wind input improves results. This fire burning location prediction method could improve air quality forecasting. Our deep learning model can improve forecasts by revealing the complex interactions of components and reflecting fire emissions' dynamic nature. This research may help improve air quality forecasts in the face of rising fire events, protecting communities across the Indian subcontinent.
更多查看译文
关键词
Fire emission,Fire forecasting,Deep learning,ConvLSTM,Air pollution forecasting
AI 理解论文
溯源树
样例
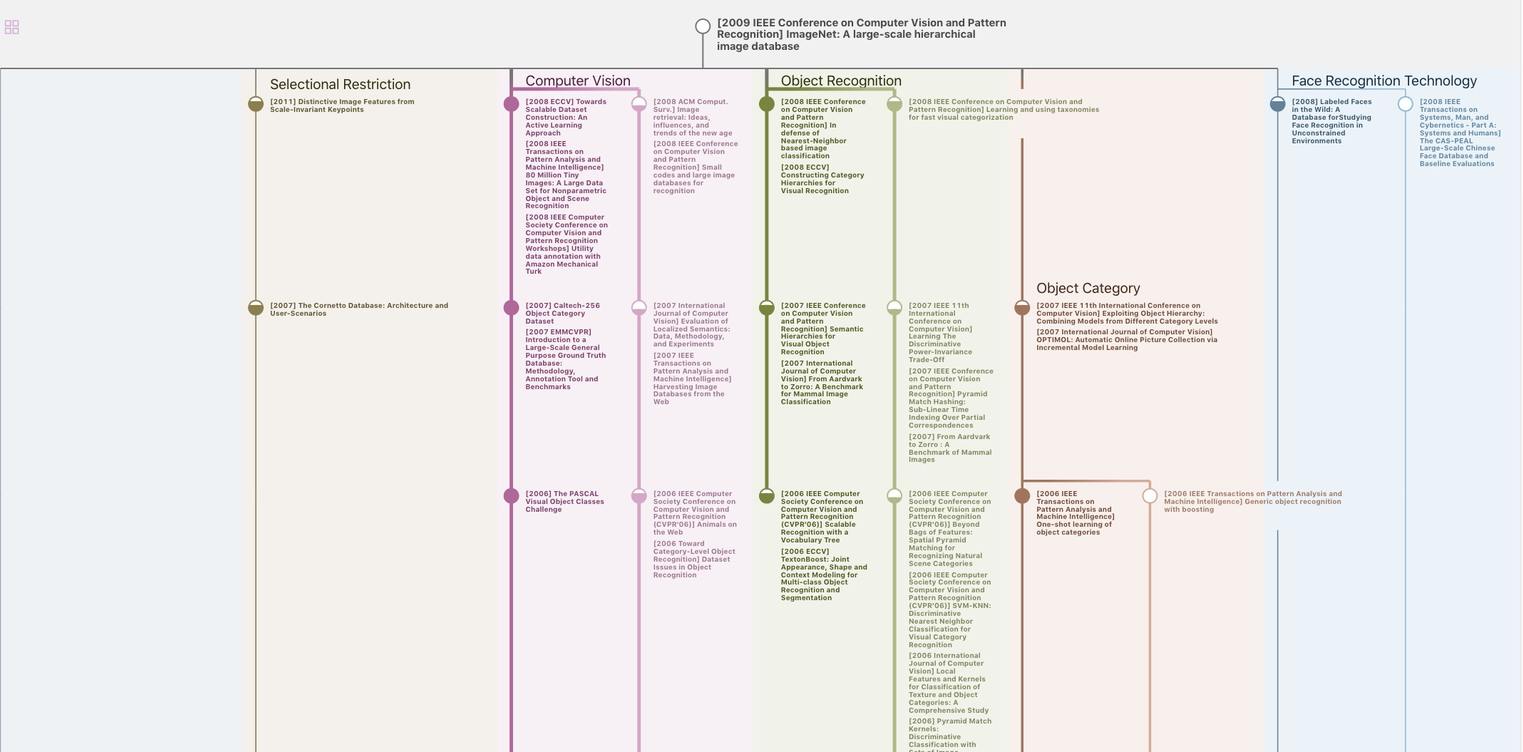
生成溯源树,研究论文发展脉络
Chat Paper
正在生成论文摘要