Load Identification Based on Attention Semisupervised Curriculum Label Learning With AVME-HT Feature
IEEE TRANSACTIONS ON INSTRUMENTATION AND MEASUREMENT(2024)
Abstract
Nonintrusive load monitoring (NILM) is a cost-effective technology for monitoring detailed electricity energy consumption. In recent years, machine learning has emerged as the predominant approach for achieving NILM tasks. However, machine learning models require an extensive volume of labeled data which is unachievable in the real world. Additionally, the collected power data is susceptible to noise interference arising from grid or measurement error. To this end, this article presents a new NILM method, adaptive variational mode extraction-Hilbert transform-excitation attention-semisupervised curriculum label learning (AVME-HT-EA-SCLL), which offers robust antinoise performance, comprehensive feature extraction, efficient utilization of unlabeled data, and exceptional classification accuracy. The main contributions of this work include the proposed adaptive variational mode extraction (AVME) method for efficient signal decomposition, a new feature extraction method, which combines AVME and Hilbert transform to extract unique features and filter out noise interference, a semisupervised learning (SSL) model based on curriculum learning strategy to identify appliances with limited labeled data, and an excitation attention (EA) network to amplify attention toward crucial information. Experimental evaluations conducted on public datasets such as the plug-load appliance identification dataset (PLAID) and the worldwide household and industry transient energy dataset (WHITED), as well as a private dataset collected in the laboratory, demonstrate that the proposed method surpasses state-of-the-art approaches.
MoreTranslated text
Key words
Feature extraction,Load modeling,Signal resolution,Computational modeling,Data models,Transient analysis,Trajectory,Curriculum learning strategy,excitation attention (EA),load identification,nonintrusive load monitoring (NILM),semisupervised learning (SSL),variational mode extraction
AI Read Science
Must-Reading Tree
Example
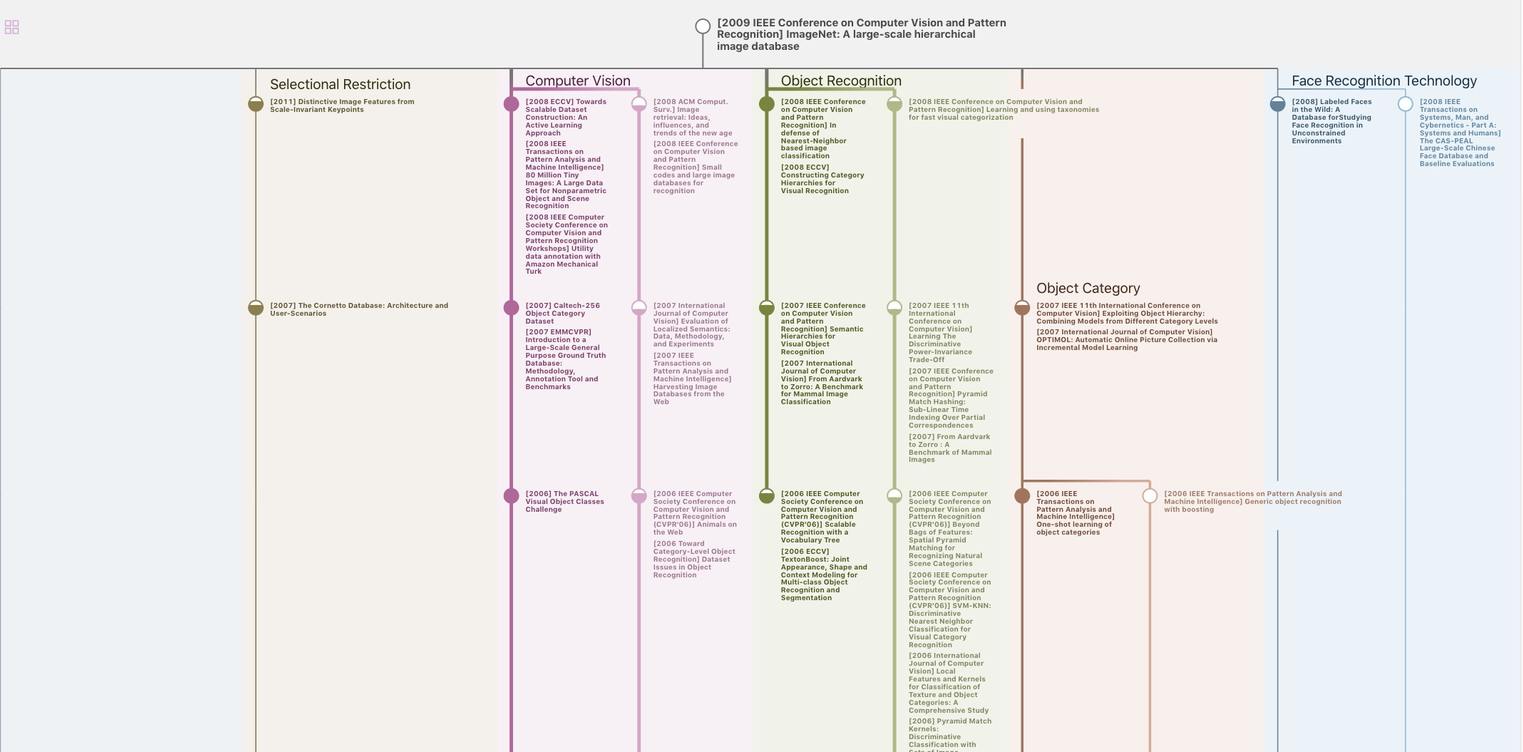
Generate MRT to find the research sequence of this paper
Chat Paper
Summary is being generated by the instructions you defined