Multi-class Probabilistic Bounds for Majority VoteClassifiers with Partially Labeled Data
JOURNAL OF MACHINE LEARNING RESEARCH(2024)
摘要
In this paper, we propose a probabilistic framework for analyzing a multi-class majorityvote classifier in the case where training data is partially labeled. First, we derive a multi-class transductive bound over the risk of the majority vote classifier, which is based on theclassifier's vote distribution over each class. Then, we introduce a mislabeling error modelto analyze the error of the majority vote classifier in the case of the pseudo-labeled trainingdata. We derive a generalization bound over the majority vote error when imperfect labelsare given, taking into account the mean and the variance of the prediction margin. Finally,we demonstrate an application of the derived transductive bound for self-training to findautomatically the confidence threshold used to determine unlabeled examples for pseudo-labeling. Empirical results on different data sets show the effectiveness of our frameworkcompared to several state-of-the-art semi-supervised approaches.
更多查看译文
关键词
semi-supervised learning,learning theory,multi-class classification,transductive inference,self-training
AI 理解论文
溯源树
样例
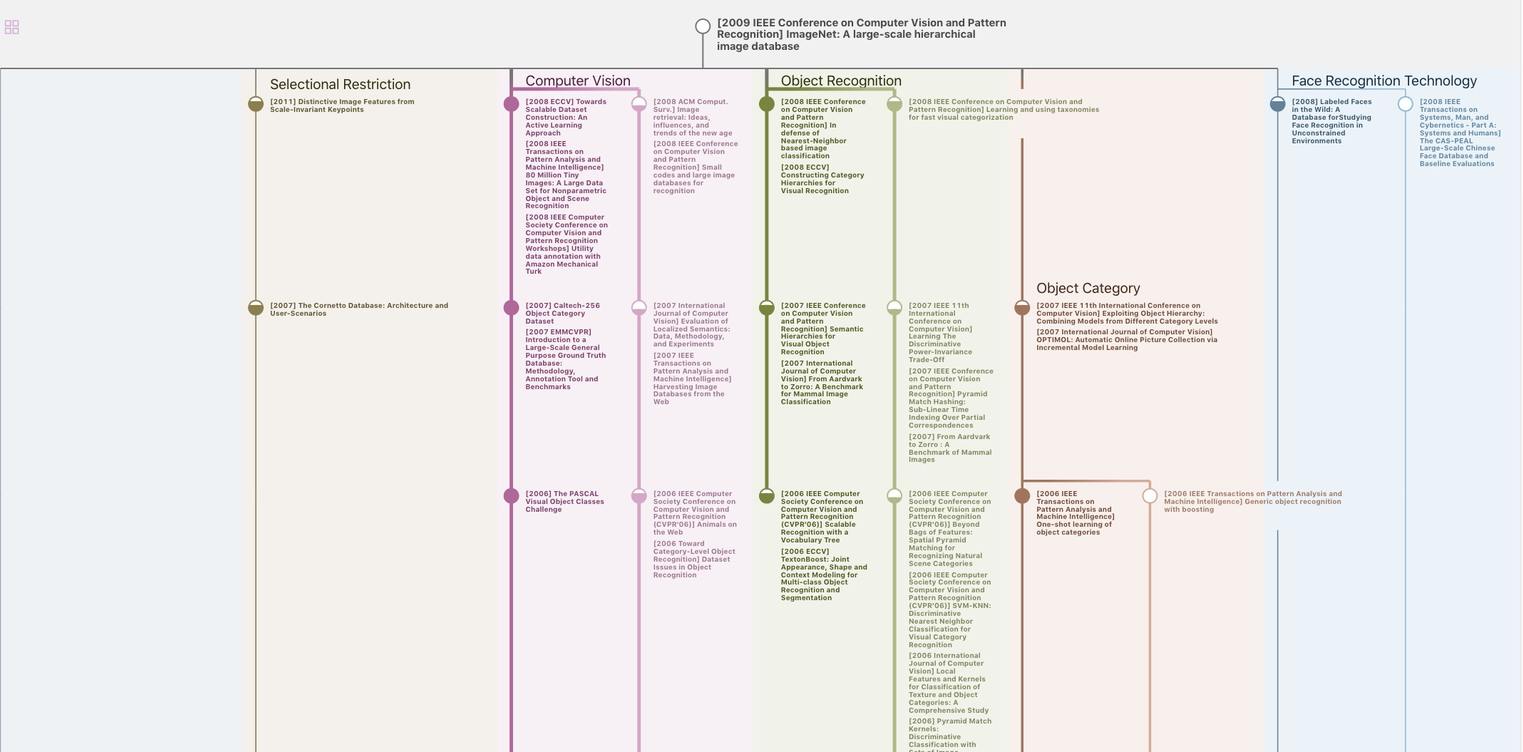
生成溯源树,研究论文发展脉络
Chat Paper
正在生成论文摘要