DeepCraftFuse: visual and deeply-learnable features work better together for esophageal cancer detection in patients with Barrett's esophagus
NEURAL COMPUTING & APPLICATIONS(2024)
摘要
Limitations in computer-assisted diagnosis include lack of labeled data and inability to model the relation between what experts see and what computers learn. Even though artificial intelligence and machine learning have demonstrated remarkable performances in medical image computing, their accountability and transparency level must be improved to transfer this success into clinical practice. The reliability of machine learning decisions must be explained and interpreted, especially for supporting the medical diagnosis. While deep learning techniques are broad so that unseen information might help learn patterns of interest, human insights to describe objects of interest help in decision-making. This paper proposes a novel approach, DeepCraftFuse, to address the challenge of combining information provided by deep networks with visual-based features to significantly enhance the correct identification of cancerous tissues in patients affected with Barrett's esophagus (BE). We demonstrate that DeepCraftFuse outperforms state-of-the-art techniques on private and public datasets, reaching results of around 95% when distinguishing patients affected by BE that is either positive or negative to esophageal cancer.
更多查看译文
关键词
Barrett's esophagus,Machine learning,Adenocarcinoma,Deep learning,Object detector
AI 理解论文
溯源树
样例
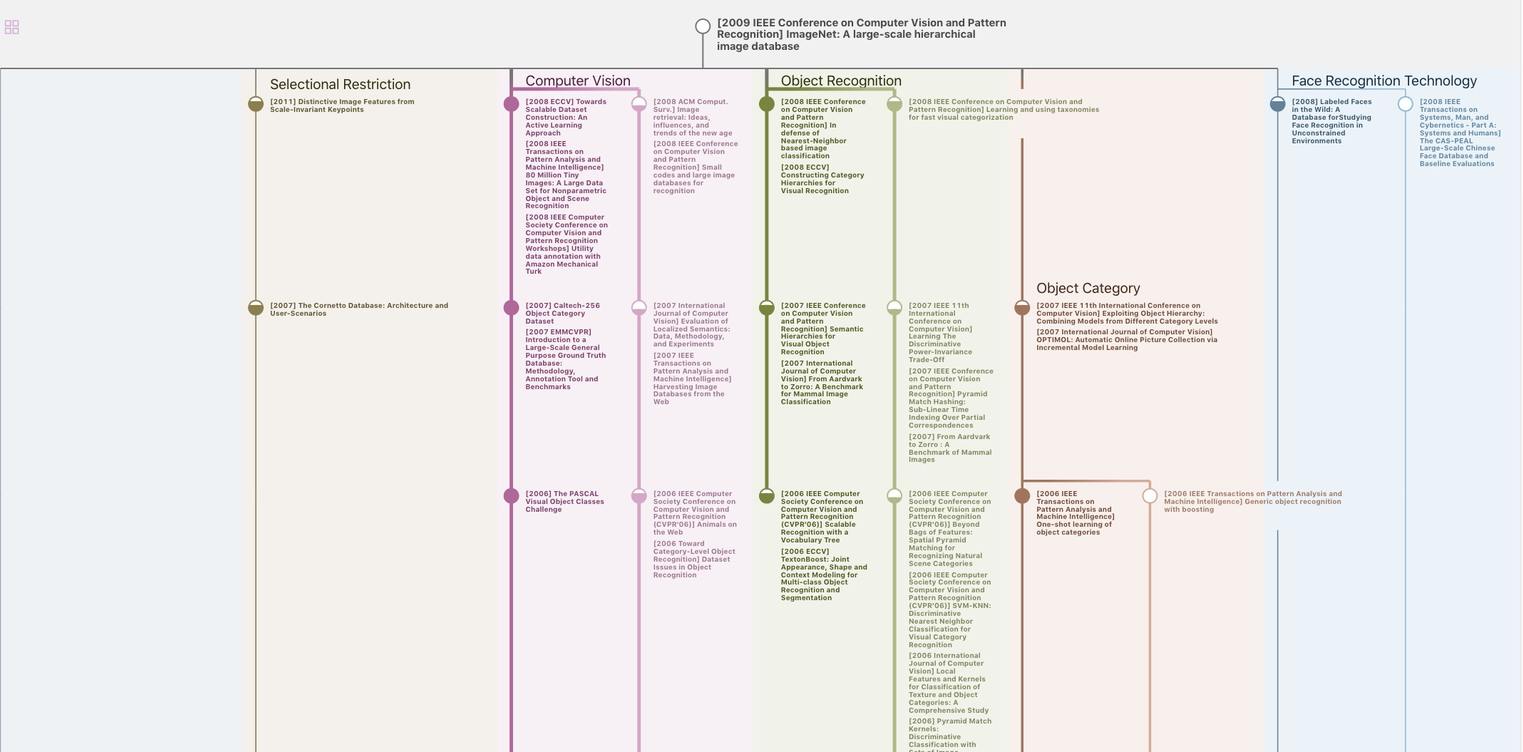
生成溯源树,研究论文发展脉络
Chat Paper
正在生成论文摘要