AIVR-Net: Attribute-based invariant visual representation learning for vehicle re-identification
KNOWLEDGE-BASED SYSTEMS(2024)
Abstract
Vehicle re -identification (ReID) aims to match and track vehicles in a surveillance system across nonoverlapping camera views. Despite great advances have been achieved in intra-domain and cross -domain vehicle ReID, most existing methods still suffer the problem caused by diverse environment changes and rarely exploit fine attribute properties which contain high-level intrinsic semantic information. Inspired by the transferable knowledge of attributes (e.g., color and model -type) in ZSL (Zero -Shot Learning), we propose a novel end -to -end attribute -guided network for vehicle re -identification, namely, Attribute Invariant Visual Representation Network (AIVR-Net), which targets to obtain attribute invariant features and facilitate discriminative visual representation learning for vehicle ReID. Specifically, we leverage the concept of composition pairs in compositional zero -shot learning to disentangle the attribute representations and design two novel modules. (i) Identity -guided Attention Module (IAM) is employed to filter out identity -irrelevant features. (ii) A Domain Alignment Module (DAM) is further proposed to align high-level semantic information at representation and gradient levels, respectively. AIVR-Net learns identity representations and visual -attribute invariant representations by multi -task training strategy. The experiment results demonstrate that AIVR-Net outperforms the state-of-the-art vehicle ReID methods and achieves excellent generalization performance on the vehicle ReID benchmarks.
MoreTranslated text
Key words
Vehicle ReID,Attribute-based,Representation disentanglement
AI Read Science
Must-Reading Tree
Example
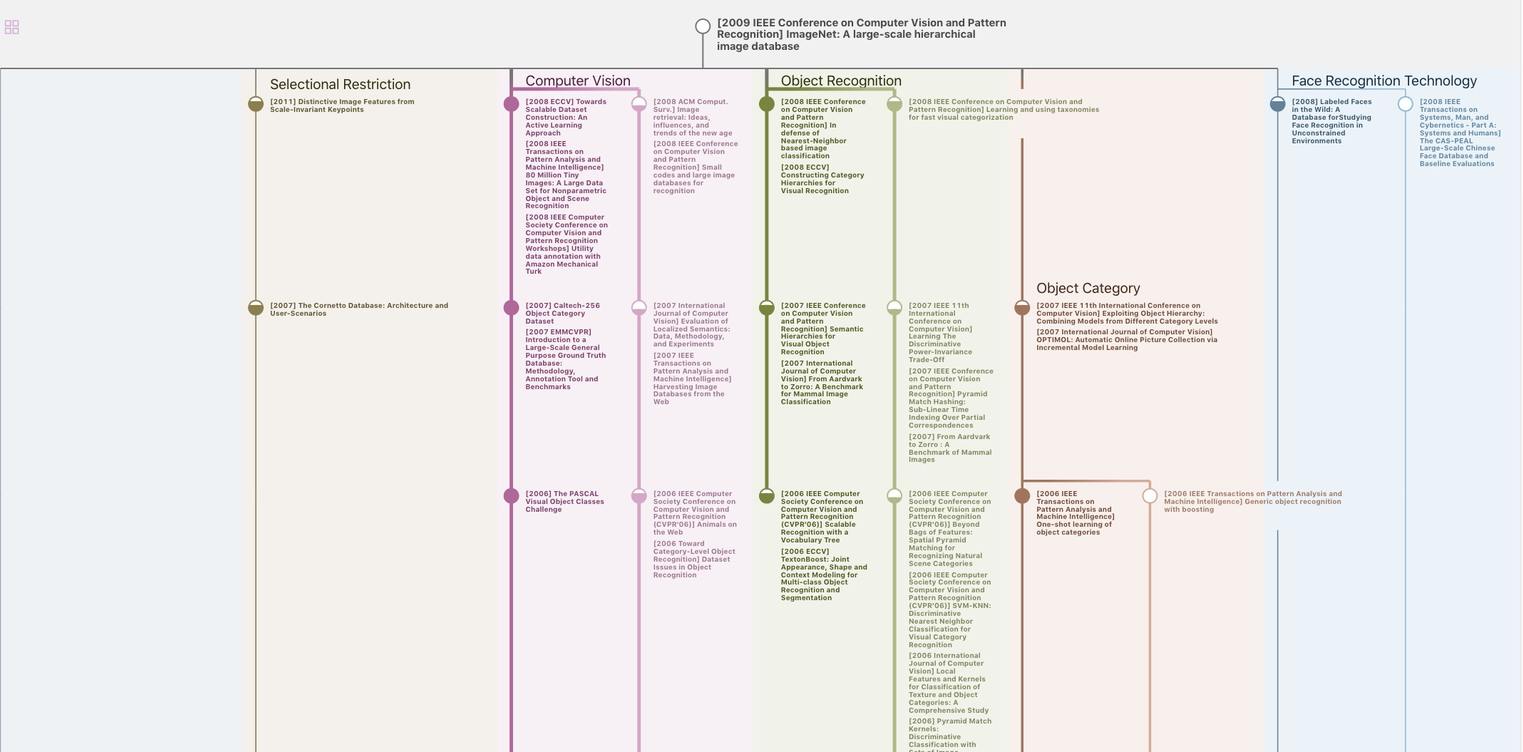
Generate MRT to find the research sequence of this paper
Chat Paper
Summary is being generated by the instructions you defined