Nonparametric Two-Sample Testing by Betting
IEEE TRANSACTIONS ON INFORMATION THEORY(2024)
摘要
We study the problem of designing consistent sequential two-sample tests in a nonparametric setting. Guided by the principle of testing by betting, we reframe this task into that of selecting a sequence of payoff functions that maximize the wealth of a fictitious bettor, betting against the null in a repeated game. In this setting, the relative increase in the bettor's wealth has a precise interpretation as the measure of evidence against the null, and thus our sequential test rejects the null when the wealth crosses an appropriate threshold. We develop a general framework for setting up the betting game for two-sample testing, in which the payoffs are selected by a prediction strategy as data-driven predictable estimates of the witness function associated with the variational representation of some statistical distance measures, such as integral probability metrics (IPMs). We then formally relate the statistical properties of the test (such as consistency, type-II error exponent and expected sample size) to the regret of the corresponding prediction strategy. We construct a practical sequential two-sample test by instantiating our general strategy with the kernel-MMD metric, and demonstrate its ability to adapt to the difficulty of the unknown alternative through theoretical and empirical results. Our framework is versatile, and easily extends to other problems; we illustrate this by applying our approach to construct consistent tests for the following problems: (i) time-varying two-sample testing with non-exchangeable observations, and (ii) an abstract class of "invariant" testing problems, including symmetry and independence testing.
更多查看译文
关键词
Testing,Games,Measurement,Task analysis,Probability,Computational efficiency,Machine learning,Sequential analysis,kernel-MMD,hypothesis testing,Integral probability metrics
AI 理解论文
溯源树
样例
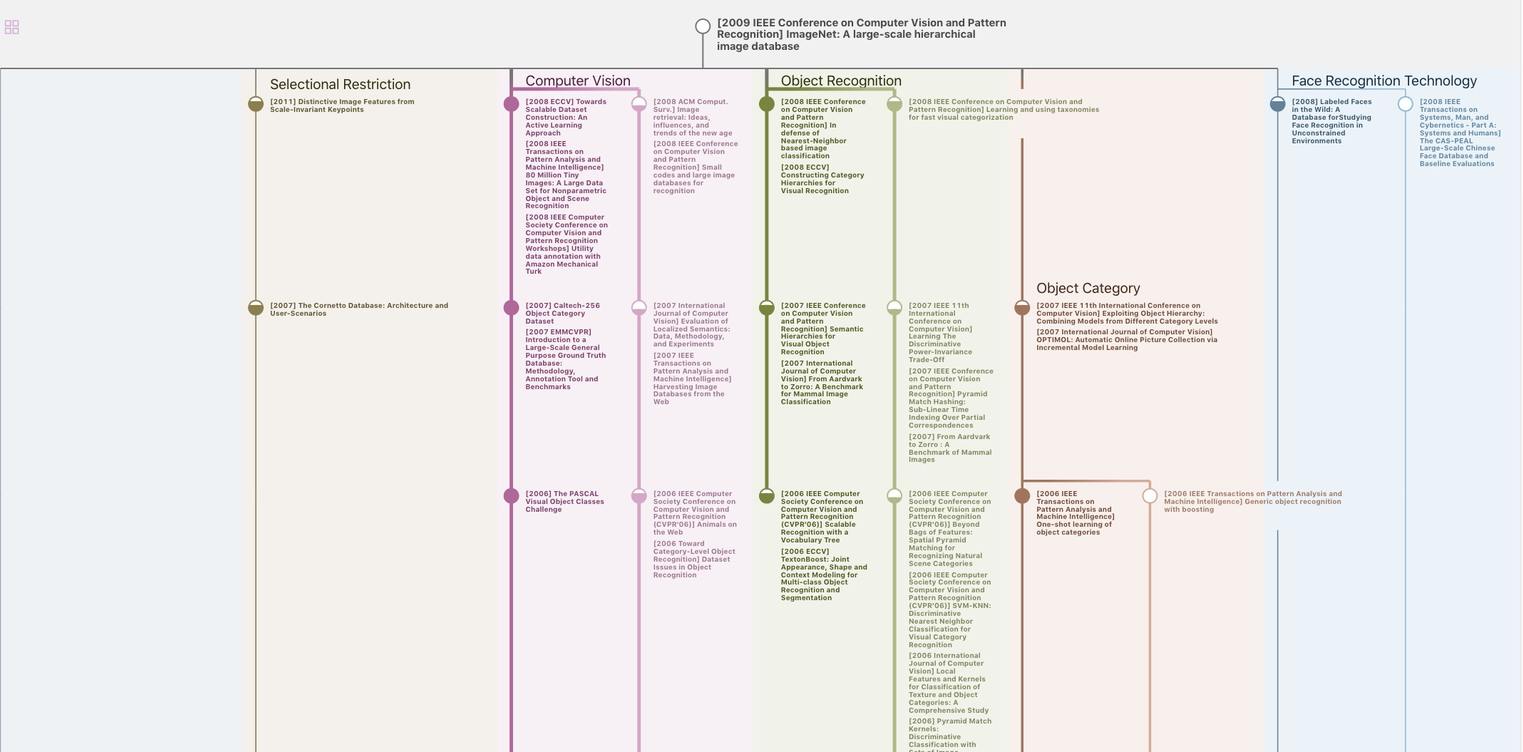
生成溯源树,研究论文发展脉络
Chat Paper
正在生成论文摘要