Tailoring Evolutionary Algorithms to Solve the Multiobjective Location-Routing Problem for Biomass Waste Collection
IEEE TRANSACTIONS ON EVOLUTIONARY COMPUTATION(2024)
Abstract
Location-routing problems (LRPs) widely exist in logistics activities. For the biomass waste collection, there is a recognized need for novel models to locate the collection facilities and plan the vehicle routes. So far most location-routing models fall into the cost-driven-only category. However, comprehensive objectives are required in the specific context, such as time-dependent pollution and speed- and load-related emission. Furthermore, LRPs are hierarchical by nature, containing the facility location problems (strategic level) and the vehicle routing problems (VRPs) (tactical level). Existing studies in this field usually adopt computational intelligence methods directly without decomposing the problem. This can be inefficient especially when multiple objectives are applied. Motivated by these, we develop a novel multiobjective optimization model for the LRP for biomass waste collection. To solve this model, we explore the way to tailor evolutionary algorithms to the hierarchical structure. We develop adapted versions of two commonly used evolutionary algorithms: 1) the genetic algorithm and 2) the ant colony optimization algorithm. For the genetic algorithm, we divide the population by the strategic level decisions, so that each subpopulation has a fixed location plan, breaking the LRP down into many multidepot VRPs. For the ant colony optimization, we use an additional pheromone vector to track the good decisions on the location level, and segregate the pheromones related to different satellite depots to avoid misleading information. Thus, the problem degenerates into VRP. Experimental results show that our proposed methods have better performances on the LRP for biomass waste collection.
MoreTranslated text
Key words
Ant colony optimization,genetic algorithm,location-routing,multiobjective optimization,multipopulation technique
AI Read Science
Must-Reading Tree
Example
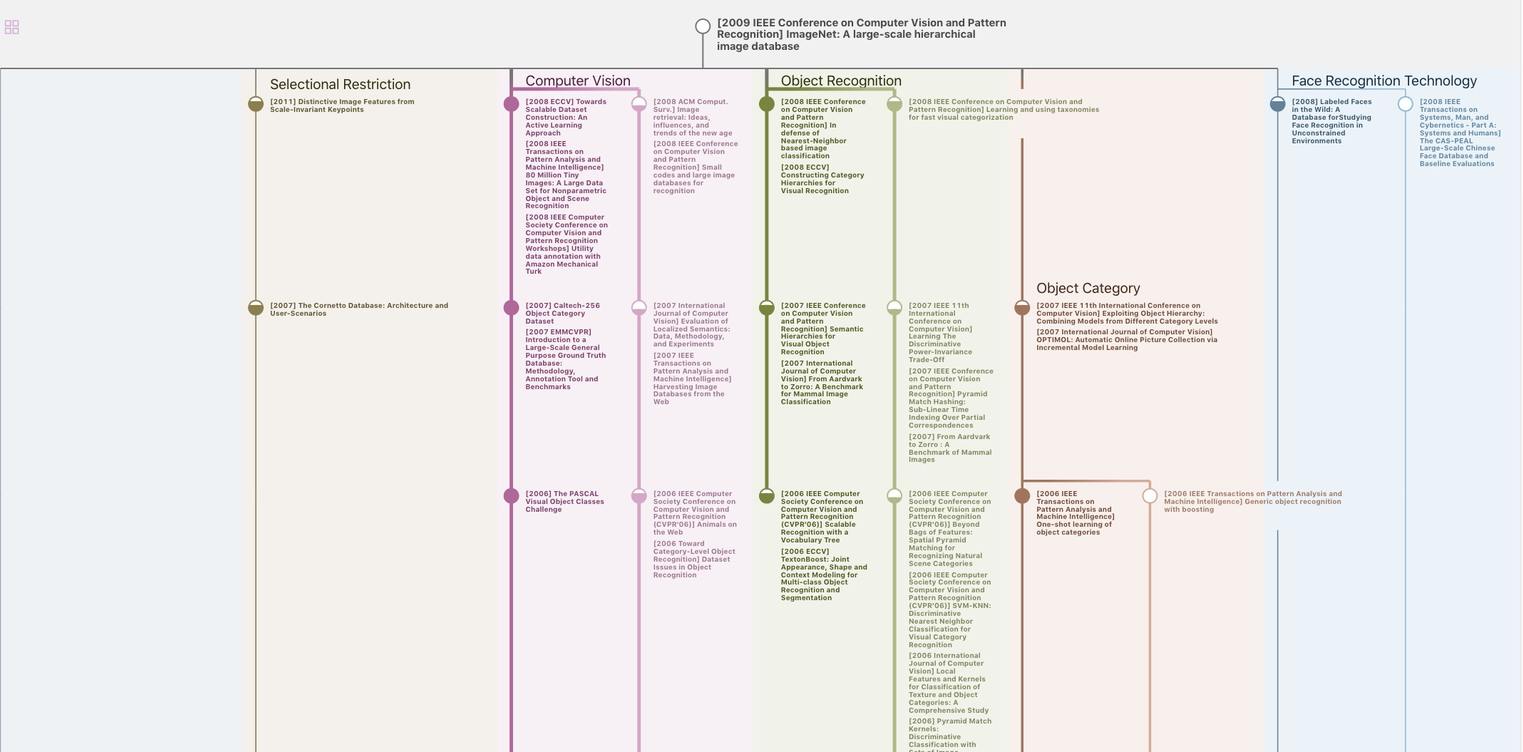
Generate MRT to find the research sequence of this paper
Chat Paper
Summary is being generated by the instructions you defined