Fault detection using machine learning based dynamic ICA-distributed CCA: Application to industrial chemical process
DIGITAL CHEMICAL ENGINEERING(2024)
摘要
Unexpected accidents and events in industrial chemical processes have resulted in a considerable number of causalities and property damage. Safety process management in industrial chemical processes is critical to avoid and ensure casualties and property damage. However, due to the immense scope and high complexity of current industrial chemical processes, the traditional safety process management approaches cannot address these challenges to attain adequate fault detection accuracy. To address this issue, an innovative machine learningbased distributed canonical correlation analysis-dynamic independent component analysis (DICA-DCCA) approach is needed to improve the fault detection effectiveness of complicated systems. The (DICA-DCCA) model could potentially detect anomalies and faults in industrial chemical data by utilizing three essential statistics:Id2,Ie 2 and squared prediction error (SPE). The practical effectiveness of the proposed frameworks is evaluated and compared using a continuous stirred tank reactor (CSTR) framework as a standard benchmark study. The research findings present that the suggested (DICA-DCCA) approach is more resilient and effective in detecting abnormalities and faults than the ICA and DICA approaches with FDR 100 % and FAR 0 %. The implied research approach is robust, operational, and productive.
更多查看译文
关键词
Fault detection,Machine learning,Industrial chemical processes,Safety process management,DICA-DCCA approach,CSTR framework
AI 理解论文
溯源树
样例
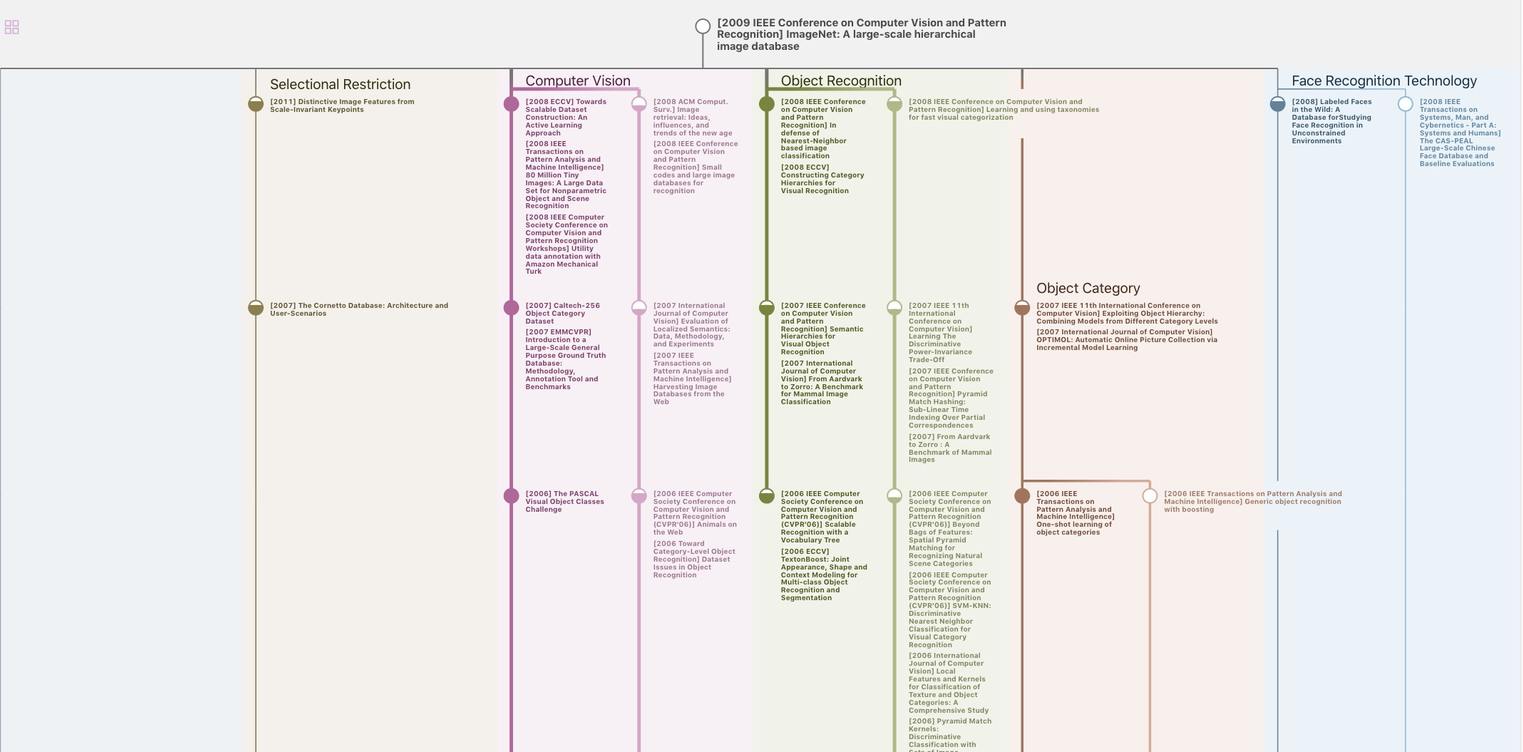
生成溯源树,研究论文发展脉络
Chat Paper
正在生成论文摘要