Accelerated end-to-end chemical synthesis development with large language models
crossref(2024)
摘要
The rapid emergence of large language model (LLM) technology presents significant opportunities to facilitate the development of synthetic reactions. In this work, we leveraged the power of GPT-4 to build a multi-agent system to handle fundamental tasks involved throughout the chemical synthesis development process. The multi-agent system comprises six specialized LLM-based agents, including Literature Scouter, Experiment Designer, Hardware Executor, Spectrum Analyzer, Separation Instructor, and Result Interpreter, which are pre-prompted to accomplish the designated tasks. A web application was built with the multi-agent system as the backend to allow chemist users to interact with experimental platforms and analyze results via natural language, thus, requiring zero-coding skills to allow easy access for all chemists. We demonstrated this multi-agent system on the development of a recently developed copper/TEMPO catalyzed aerobic alcohol oxidation to aldehyde reaction, and this LLM multi-agent copiloted end-to-end reaction development process includes: literature search and information extraction, substrate scope and condition screening, reaction kinetics study, reaction condition optimization, reaction scale-up and product purification. This work showcases the trilogy among chemist users, LLM-based agents, and automated experimental platforms to reform the traditional expert-centric and labor-intensive reaction development workflow.
更多查看译文
AI 理解论文
溯源树
样例
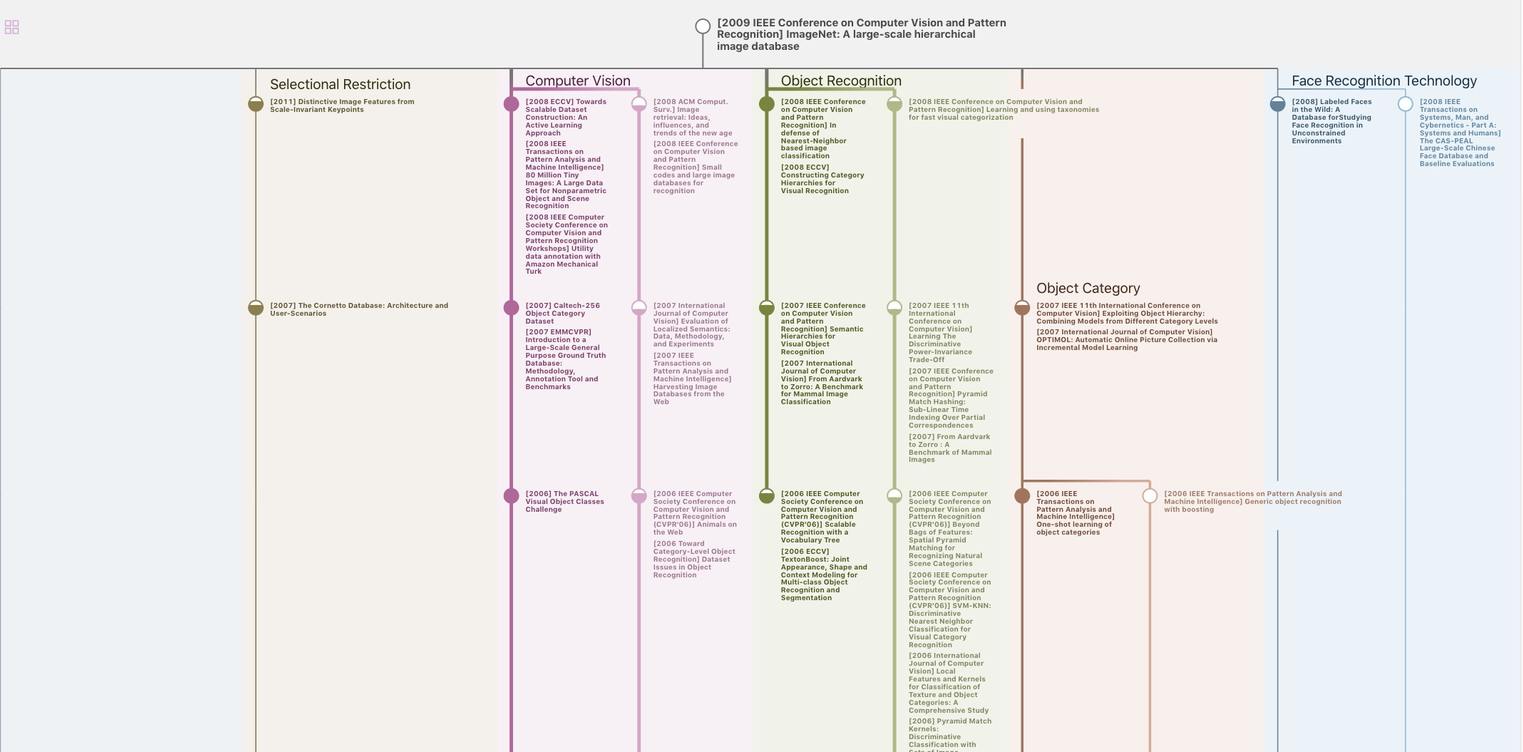
生成溯源树,研究论文发展脉络
Chat Paper
正在生成论文摘要