MeSAM: Multiscale Enhanced Segment Anything Model for Optical Remote Sensing Images.
IEEE Trans. Geosci. Remote. Sens.(2024)
Abstract
Segment anything model (SAM) has been widely applied to various downstream tasks for its excellent performance and generalization capability. However, SAM exhibits three limitations related to remote sensing semantic segmentation task: 1) the image encoders excessively lose high-frequency information, such as object boundaries and textures, resulting in rough segmentation masks; 2) due to being trained on natural images, SAM faces difficulty in accurately recognizing objects with large-scale variations and uneven distribution in remote sensing images; 3) the output tokens used for mask prediction are trained on natural images and not applicable to remote sensing image segmentation. In this paper, we explore an efficient paradigm for applying SAM to the semantic segmentation of remote sensing images. Furthermore, we propose MeSAM, a new SAM fine-tuning method more suitable for remote sensing images to adapt it to semantic segmentation tasks. Our method first introduces an inception mixer into the image encoder to effectively preserve high-frequency features. Secondly, by designing a mask decoder with remote-sensing correction and incorporating multiscale connections, we make up the difference in SAM from natural images to remote sensing images. Experimental results demonstrated that our method significantly improves the segmentation accuracy of SAM for remote sensing images, outperforming some state-of-the-art methods. The code will be available at https://github.com/Magic-lem/MeSAM.
MoreTranslated text
Key words
Segment anything model,semantic segmentation,high-frequency,multiscale,remote sensing
AI Read Science
Must-Reading Tree
Example
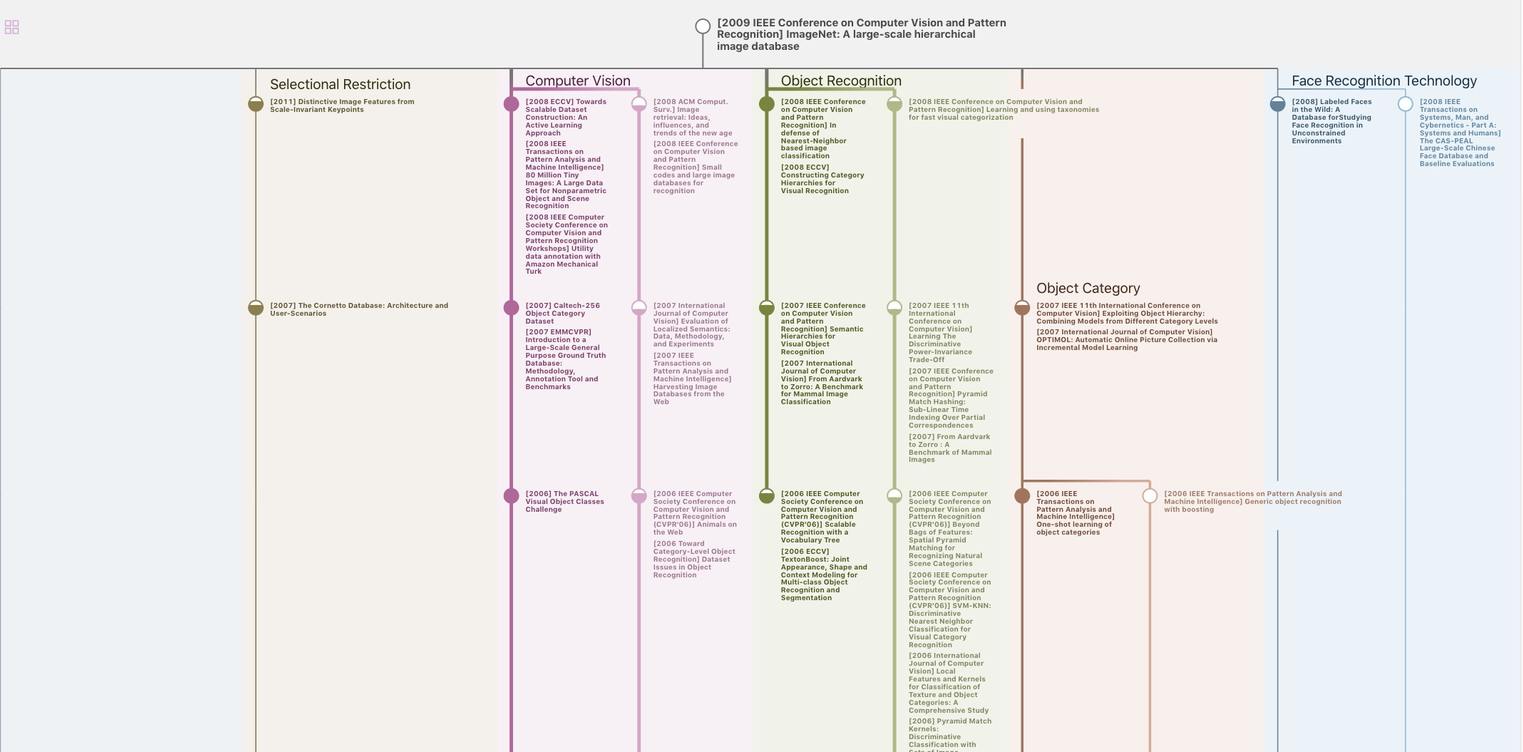
Generate MRT to find the research sequence of this paper
Chat Paper
Summary is being generated by the instructions you defined