A Wavelet-Domain Consistency-Constrained Compressive Sensing Framework Based on Memory-Boosted Guidance Filtering.
IEEE Trans. Instrum. Meas.(2024)
摘要
In recent years, a large number of researchers have begun to embed convolutional neural networks into traditional Compressive Sensing (CS) reconstruction algorithms. They have proposed a series of Deep Unfolding Networks (DUNs) with the characteristics of having good interpretability and high-quality reconstruction. However, most DUNs only use the inherent CS model, which leads to the limited reconstruction performance. In addition, simple reconstruction networks cannot well remove noises from features. To address the above issues, this paper proposes a wavelet-domain consistency-constrained CS framework. We introduce discrete wavelet transform into the CS optimization model and unfold its optimization algorithm into a deep dual-domain hybrid reconstruction network. The overall network of the proposed method is named Memory-boosted Dual-domain Guidance Filtering Network (MDGF-Net). MDGF-Net utilizes frequency-domain information to supplement spatial-domain image reconstruction. Furthermore, guided filtering is leveraged to filter out noises in the reconstructed features. Here, we design two consecutive denoising modules, namely, dual-domain memory-boosted guided filtering module and self-guided memory-boosted filtering module. By combining the guided filtering and long short-term memory mechanism, these two modules can effectively reduce the information loss while removing the feature noise, and retain the valid information. Numerous experimental results on various datasets indicate that the proposed MDGF-Net achieves superior reconstruction performance compared to several state-of-the-art DUNs. Our codes are publicly available at https://github.com/mdcnn/MDGF-Net-Plus.
更多查看译文
关键词
Compressive sensing,interpretable reconstruction network,guided filtering,discrete wavelet transform,deep learning
AI 理解论文
溯源树
样例
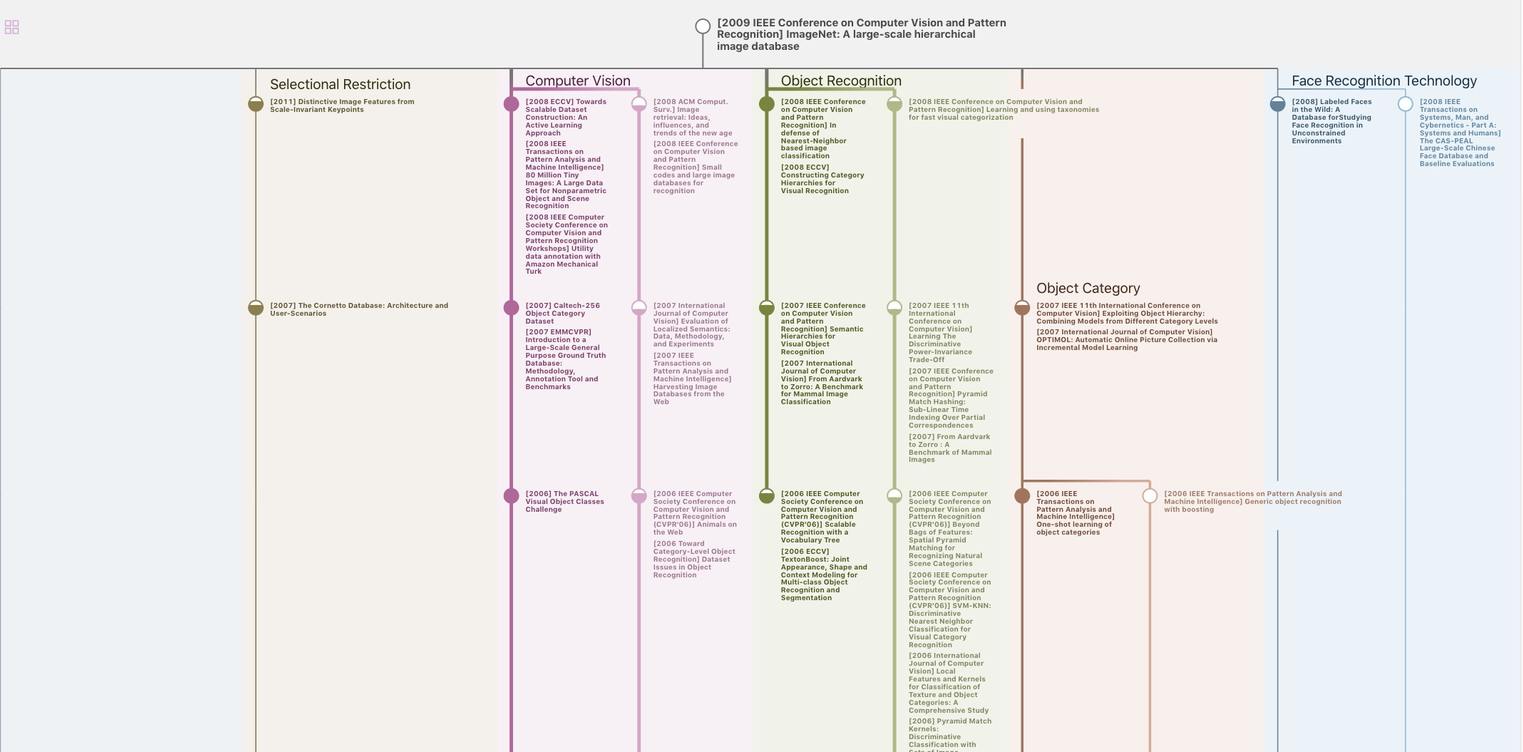
生成溯源树,研究论文发展脉络
Chat Paper
正在生成论文摘要