Advanced Imaging Technologies With Ensemble Learning for Consumer Products Identification and Classification
IEEE Transactions on Consumer Electronics(2024)
摘要
Consumer product recognition involves utilizing computer vision (CV) and machine learning (ML) systems for identifying and recognizing numerous consumer goods in video or image frames. This technology determines applications in retail, e-commerce, security systems, and inventory management. The purpose is to automate the detection of certain products or objects in visual data, streamlining several procedures and improving efficacy. Deep Learning (DL), implementing structures such as convolutional neural networks (CNNs), automates the detection and classification of consumer products in images or video frames. Trained on diverse datasets, these techniques learn intricate features, benefiting retail and e-commerce by enhancing inventory management and improving the shopping experience through automated product detection. This study develops a honey badger algorithm with ensemble learning for consumer product identification and classification (HBAEL-CPIC) technique. The goal of the HBAEL-CPIC technique is to detect and classify various kinds of consumer products accurately. To accomplish this, the HBAEL-CPIC technique employs a non-local means (NLM) filter to eradicate the occurrence of the noise. Next, the HBAEL-CPIC technique follows the capsule network (CapsNet) model for the feature extractor process. Meanwhile, the hyperparameter tuning of the CapsNet approach has been carried out using the HBA. At last, the ensemble of three models namely extreme learning machine (ELM), descriptive back propagated: radial basis function (DBRF), and extreme gradient boosting (XGBoost) are used for classification. To highlight the improved detection solution of the HBAEL-CPIC technique, a series of experiments were performed. The comprehensive comparison study of the HBAEL-CPIC technique exhibited a superior accuracy value of 96.56% over other DL approaches.
更多查看译文
关键词
Consumer Products Detection,CapsNet,Hyperparameter Tuning,Ensemble Learning,XGBoost
AI 理解论文
溯源树
样例
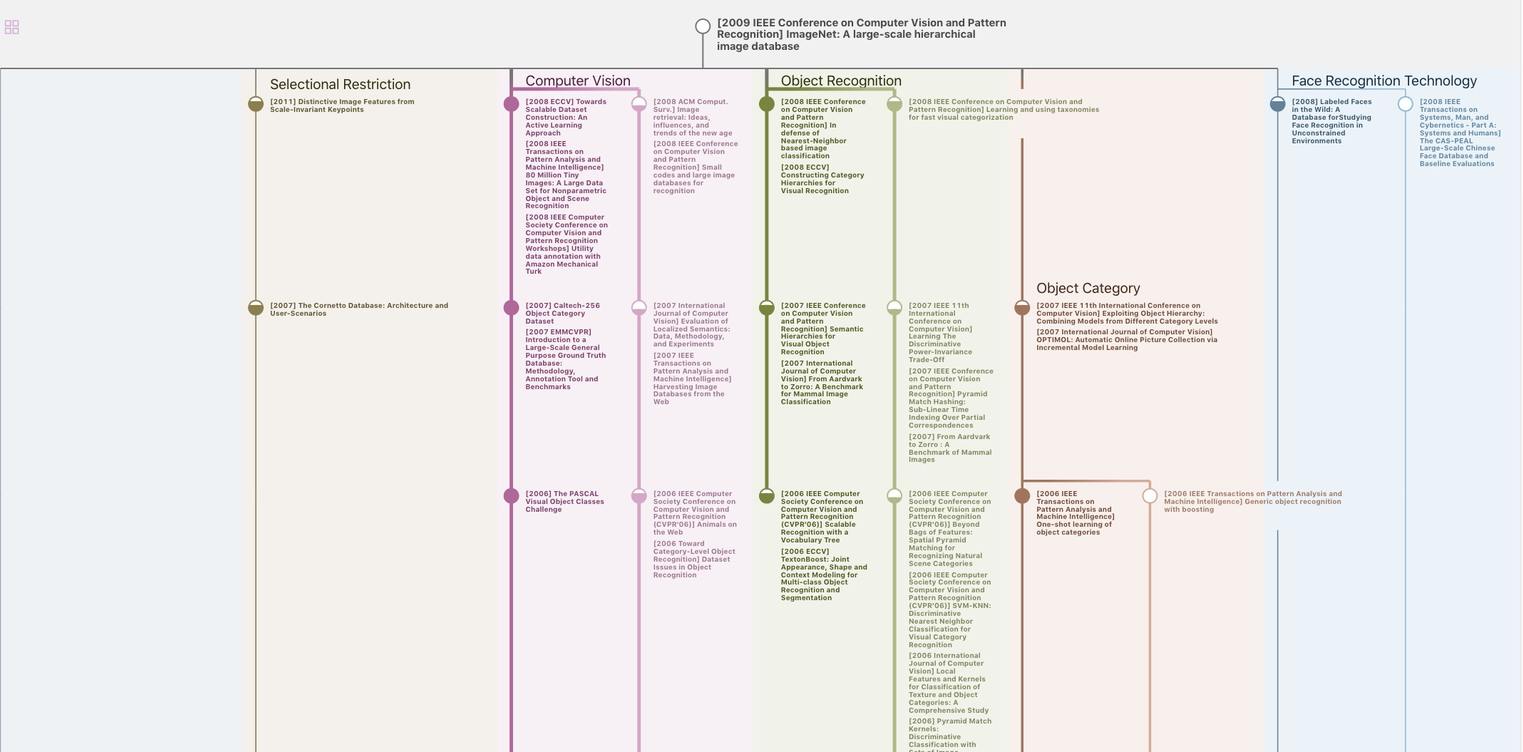
生成溯源树,研究论文发展脉络
Chat Paper
正在生成论文摘要