Tensor Low-rank Graph Embedding and Learning for One-Step Incomplete Multi-view Clustering
IEEE Transactions on Multimedia(2024)
Abstract
Traditional multi-view algorithms typically require data to be complete, and these algorithms may not be suitable or effective when dealing with incomplete data. As a result, the research field has witnessed the emergence of methods specifically designed for addressing incomplete multi-view clustering. In contrast, most of existing incomplete multi-view algorithms primarily emphasize capturing global information while neglecting the importance of local information. To overcome these challenges, we put forward a novel approach, named tensor low-rank graph embedding and learning for one-step incomplete multi-view clustering. We combine the beneficial aspects of graph embedding and tensor low-rank in this method, which not only focuses on local relationships but also provides insights into the global structure. Firstly, the initial similarity graph matrix is constructed using the inter-dependency between views. Secondly, the similarity graph matrix is structured as a third-order tensor and constrained by the tensor nuclear norm. This constraint enables the capturing of higher-order correlations across multiple views. Thirdly, graph embedding is added to obtain a common feature representation. Finally, the algorithm incorporates clustering to determine the optimal clustering labels. We conducted experiments comparing our algorithm with seven different incomplete multi-view methods using four different evaluation metrics. The experimental results indicate that our algorithm achieved the best clustering performance across five datasets under various missing rates. Especially when faced with a 50% missing rate in the Extended YaleB dataset, the clustering accuracy and normalized mutual information of our method are improved by 28.23% and 28.11%, respectively, compared to the second-best algorithm.
MoreTranslated text
Key words
Graph embedding,Incomplete multi-view,One-step,Tensor low-rank constraint,Multi-view clustering
AI Read Science
Must-Reading Tree
Example
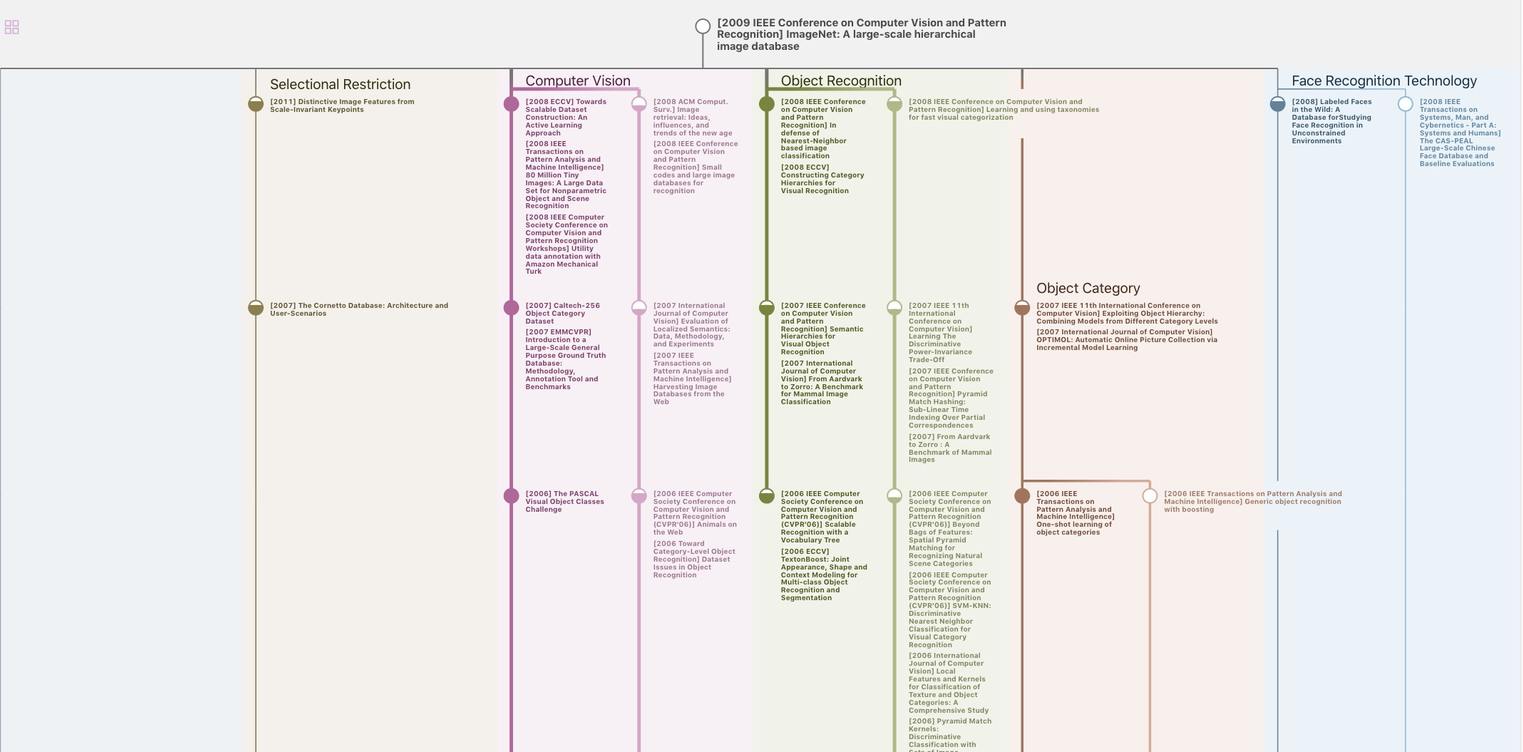
Generate MRT to find the research sequence of this paper
Chat Paper
Summary is being generated by the instructions you defined