DeepDamageNet: A two-step deep-learning model for multi-disaster building damage segmentation and classification using satellite imagery
CoRR(2024)
Abstract
Satellite imagery has played an increasingly important role in post-disaster
building damage assessment. Unfortunately, current methods still rely on manual
visual interpretation, which is often time-consuming and can cause very low
accuracy. To address the limitations of manual interpretation, there has been a
significant increase in efforts to automate the process. We present a solution
that performs the two most important tasks in building damage assessment,
segmentation and classification, through deep-learning models. We show our
results submitted as part of the xView2 Challenge, a competition to design
better models for identifying buildings and their damage level after exposure
to multiple kinds of natural disasters. Our best model couples a building
identification semantic segmentation convolutional neural network (CNN) to a
building damage classification CNN, with a combined F1 score of 0.66,
surpassing the xView2 challenge baseline F1 score of 0.28. We find that though
our model was able to identify buildings with relatively high accuracy,
building damage classification across various disaster types is a difficult
task due to the visual similarity between different damage levels and different
damage distribution between disaster types, highlighting the fact that it may
be important to have a probabilistic prior estimate regarding disaster damage
in order to obtain accurate predictions.
MoreTranslated text
AI Read Science
Must-Reading Tree
Example
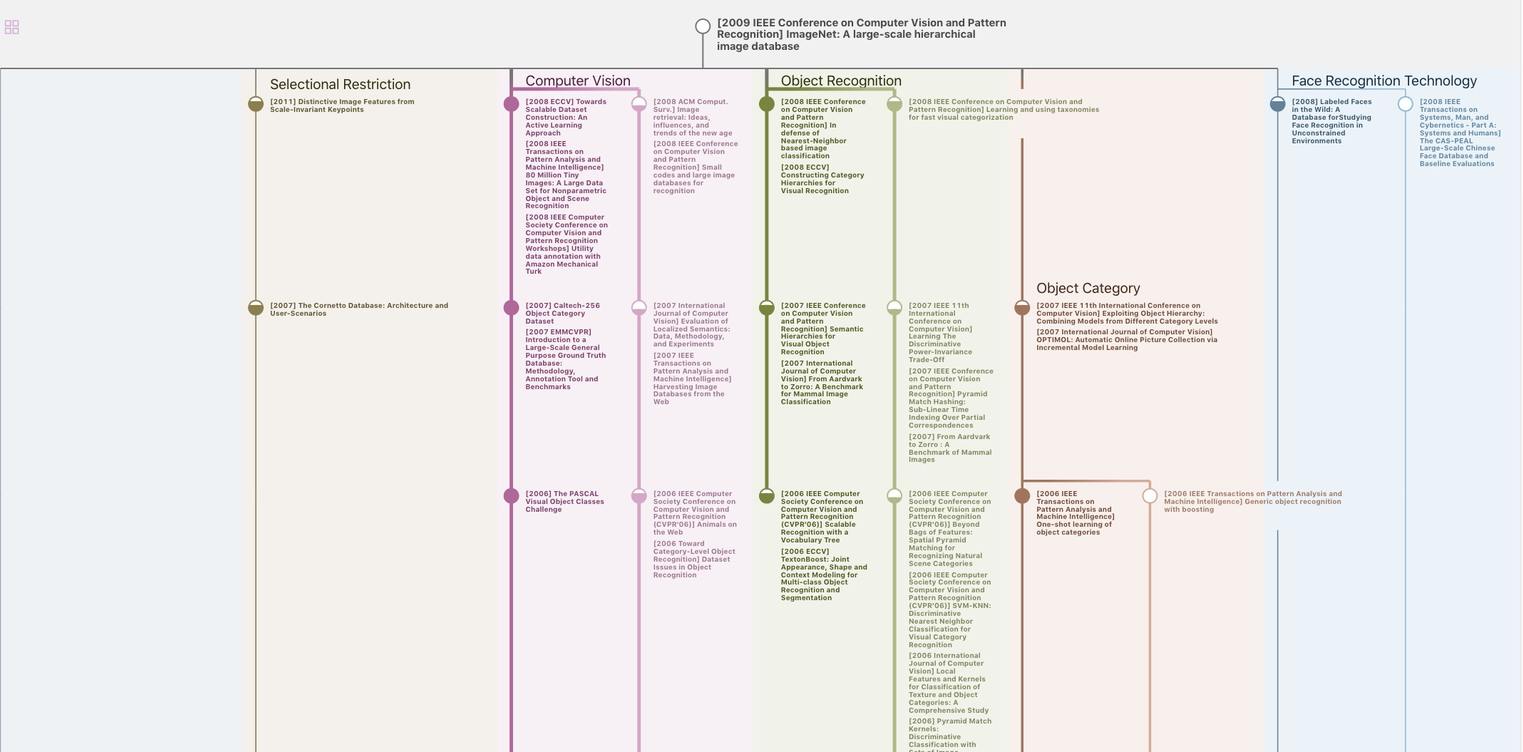
Generate MRT to find the research sequence of this paper
Chat Paper
Summary is being generated by the instructions you defined