Bridging large language model disparities: Skill tagging of multilingual educational content
BRITISH JOURNAL OF EDUCATIONAL TECHNOLOGY(2024)
摘要
The adoption of large language models (LLMs) in education holds much promise. However, like many technological innovations before them, adoption and access can often be inequitable from the outset, creating more divides than they bridge. In this paper, we explore the magnitude of the country and language divide in the leading open-source and proprietary LLMs with respect to knowledge of K-12 taxonomies in a variety of countries and their performance on tagging problem content with the appropriate skill from a taxonomy, an important task for aligning open educational resources and tutoring content with state curricula. We also experiment with approaches to narrowing the performance divide by enhancing LLM skill tagging performance across four countries (the USA, Ireland, South Korea and India-Maharashtra) for more equitable outcomes. We observe considerable performance disparities not only with non-English languages but with English and non-US taxonomies. Our findings demonstrate that fine-tuning GPT-3.5 with a few labelled examples can improve its proficiency in tagging problems with relevant skills or standards, even for countries and languages that are underrepresented during training. Furthermore, the fine-tuning results show the potential viability of GPT as a multilingual skill classifier. Using both an open-source model, Llama2-13B, and a closed-source model, GPT-3.5, we also observe large disparities in tagging performance between the two and find that fine-tuning and skill information in the prompt improve both, but the closed-source model improves to a much greater extent. Our study contributes to the first empirical results on mitigating disparities across countries and languages with LLMs in an educational context.Practitioner notes What is already known about this topic Recent advances in generative AI have led to increased applications of LLMs in education, offering diverse opportunities. LLMs excel predominantly in English and exhibit a bias towards the US context. Automated content tagging has been studied using English-language content and taxonomies. What this paper adds Investigates the country and language disparities in LLMs concerning knowledge of educational taxonomies and their performance in tagging content. Presents the first empirical findings on addressing disparities in LLM performance across countries and languages within an educational context. Improves GPT-3.5's tagging accuracy through fine-tuning, even for non-US countries, starting from zero accuracy. Extends automated content tagging to non-English languages using both open-source and closed-source LLMs. Implications for practice and/or policy Underscores the importance of considering the performance generalizability of LLMs to languages other than English. Highlights the potential viability of ChatGPT as a skill tagging classifier across countries.
更多查看译文
关键词
ChatGPT,Llama2,LLM,multilingual,skill tagging
AI 理解论文
溯源树
样例
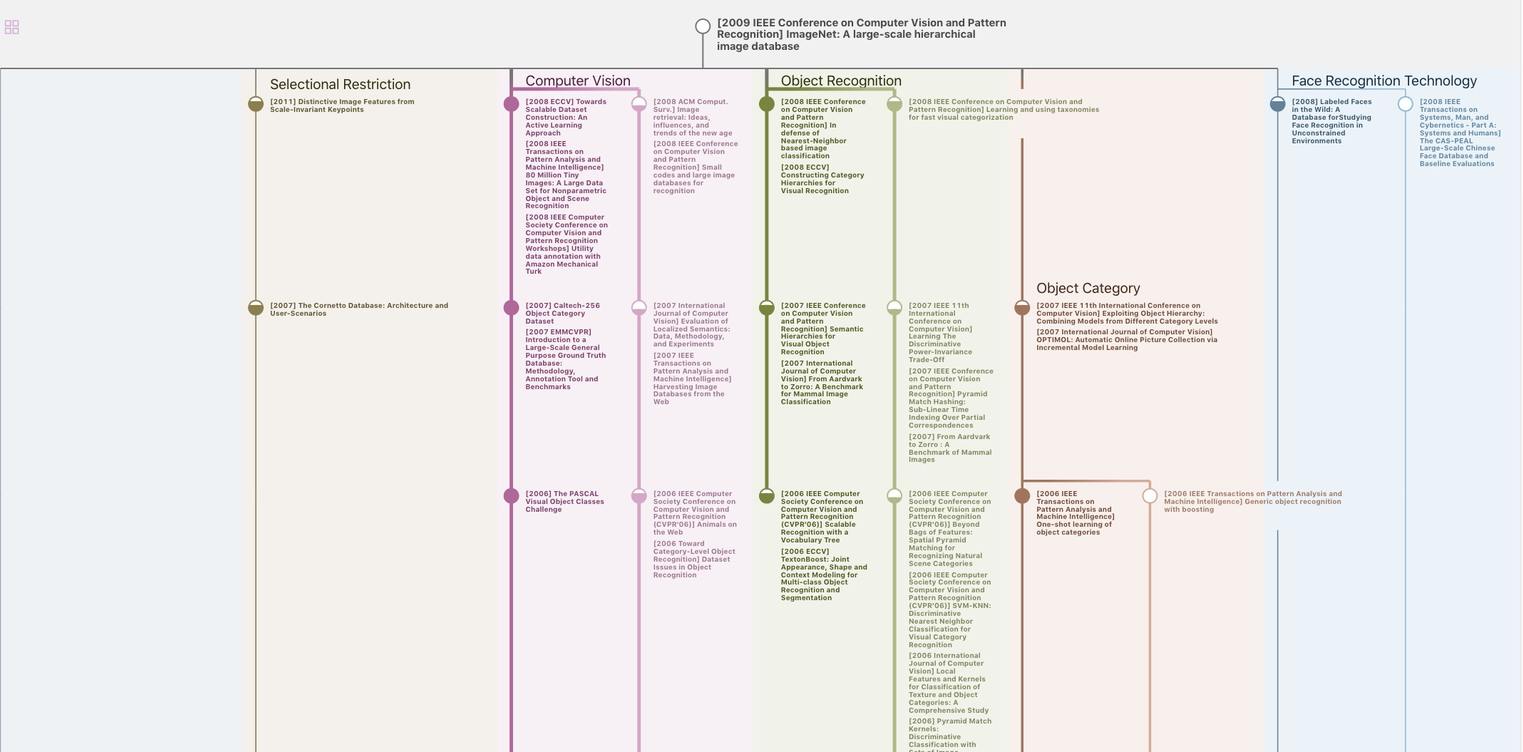
生成溯源树,研究论文发展脉络
Chat Paper
正在生成论文摘要