Unity is Strength? Benchmarking the Robustness of Fusion-based 3D Object Detection against Physical Sensor Attack
WWW 2024(2024)
摘要
As a safety-critical application, Autonomous Driving (AD) has received growing attention from security researchers. AD heavily relies on sensors for perception. However, sensors themselves are susceptible to various threats since they are exposed to the environments and vulnerable to malicious or interfering signals. To cope with situations where a sensor might malfunction, Multi Sensor Fusion (MSF) was proposed as a general strategy to enhance the robustness of perception models. In this paper, we focus on investigating MSF security under various sensor attacks and wish to answer the following research questions: (1)Does fusion enhance robustness or not? (2)How does the architecture of the fusion model influence robustness? To this end, we establish a rigorous benchmark for fusion-based 3D object detection robustness. Our new benchmark features 5 types of LiDAR attacks and 6 types of camera attacks. Different from traditional benchmarks, we take the physical sensor attacks into consideration during the corruption construction. Then, we systematically investigate 7 MSF-based and 5 single-modality 3D object detection models with different fusion architectures. We release the benchmarks and codes to facilitate future studies: \textcolorblue \hrefhttps://github.com/Jinzizhisir/PSA-Fusion https://github.com/Jinzizhisir/PSA-Fusion .
更多查看译文
AI 理解论文
溯源树
样例
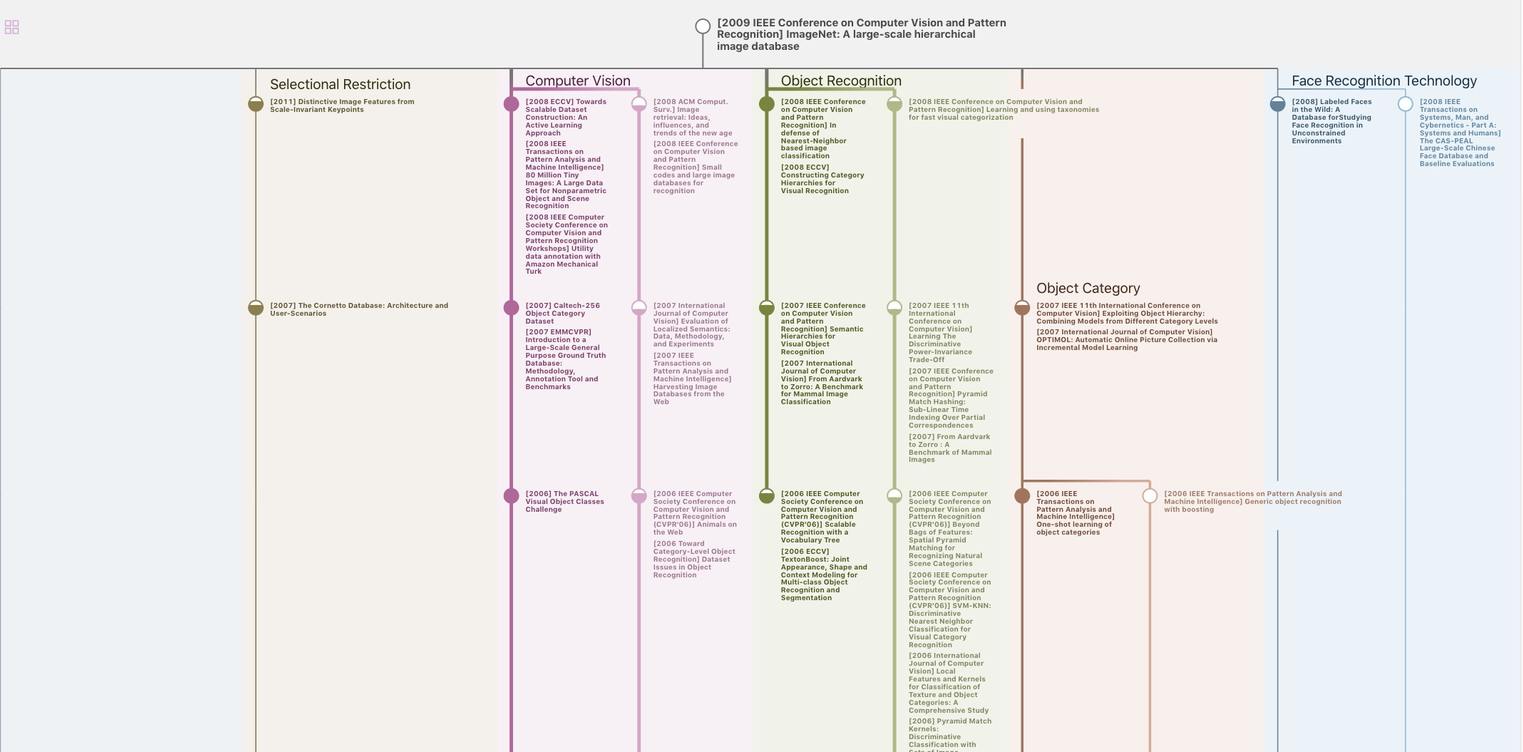
生成溯源树,研究论文发展脉络
Chat Paper
正在生成论文摘要