Intraday residual transfer learning in minimally observed power distribution networks dynamic state estimation
Data-Centric Engineering(2024)
摘要
Traditionally, electricity distribution networks were designed for unidirectional power flow without the need to accommodate generation installed at the point of use. However, with the increase in Distributed Energy Resources and other Low Carbon Technologies, the role of distribution networks is changing. This shift brings challenges, including the need for intensive metering and more frequent reconfiguration to identify threats from voltage and thermal violations. Mitigating action through reconfiguration is informed by State Estimation, which is especially challenging for low voltage distribution networks where the constraints of low observability, non-linear load relationships, and highly unbalanced systems all contribute to the difficulty of producing accurate state estimates. To counter low observability, this paper proposes the application of a novel transfer learning methodology, based upon the concept of conditional online Bayesian transfer, to make forward predictions of bus pseudo-measurements. Day ahead load forecasts at a fully observed point on the network are adjusted using the intraday residuals at other points in the network to provide them with load forecasts without the need for a complete set of forecast models at all substations. These form pseudo-measurements that then inform the state estimates at future time points. This methodology is demonstrated on both a representative IEEE Test network and on an actual GB 11 kV feeder network.
更多查看译文
关键词
power distribution,power system modeling,short term load forecasting,transfer learning
AI 理解论文
溯源树
样例
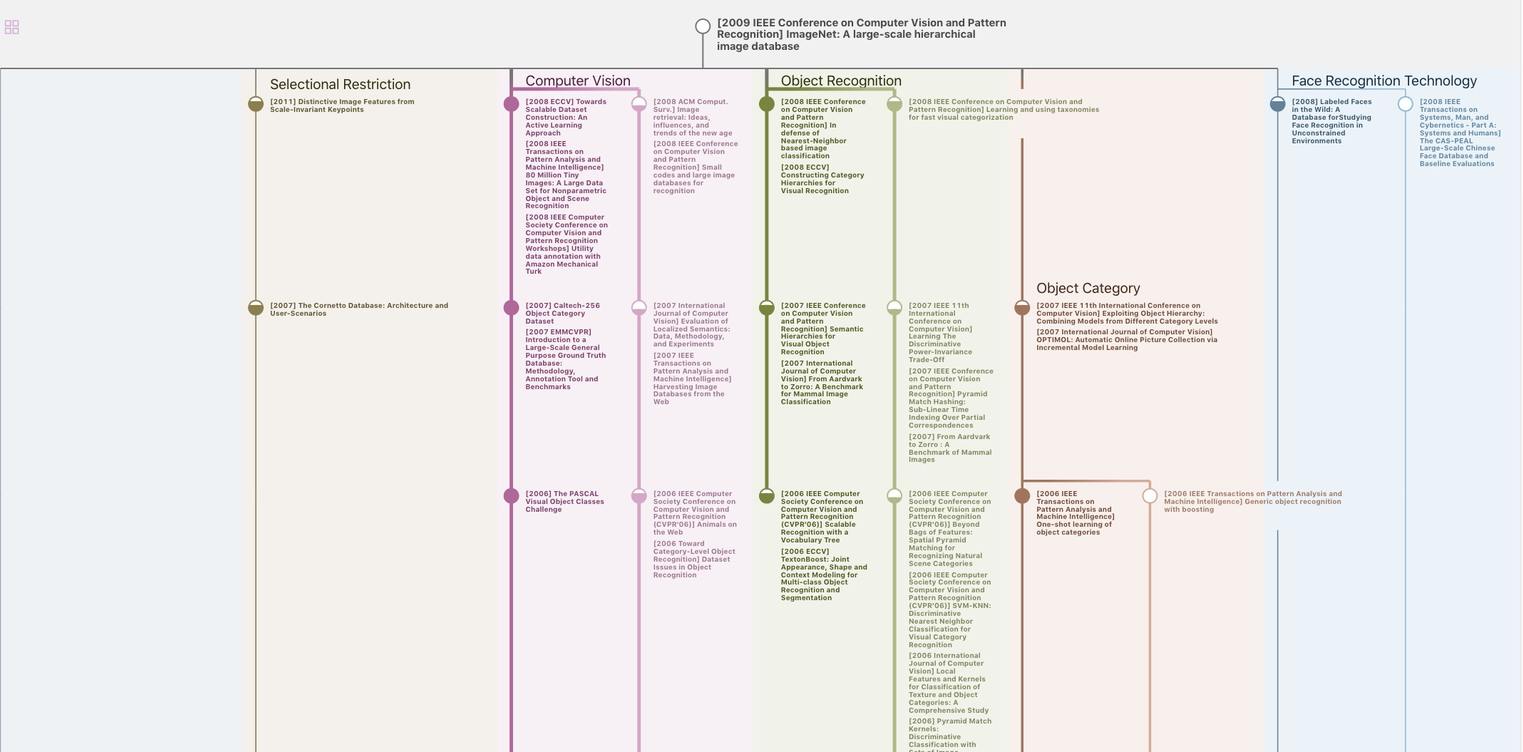
生成溯源树,研究论文发展脉络
Chat Paper
正在生成论文摘要