The Development of Thermodynamically Consistent and Physics-Informed Equation-of-state Model Through Machine Learning
APL Machine Learning(2024)
AI Read Science
Must-Reading Tree
Example
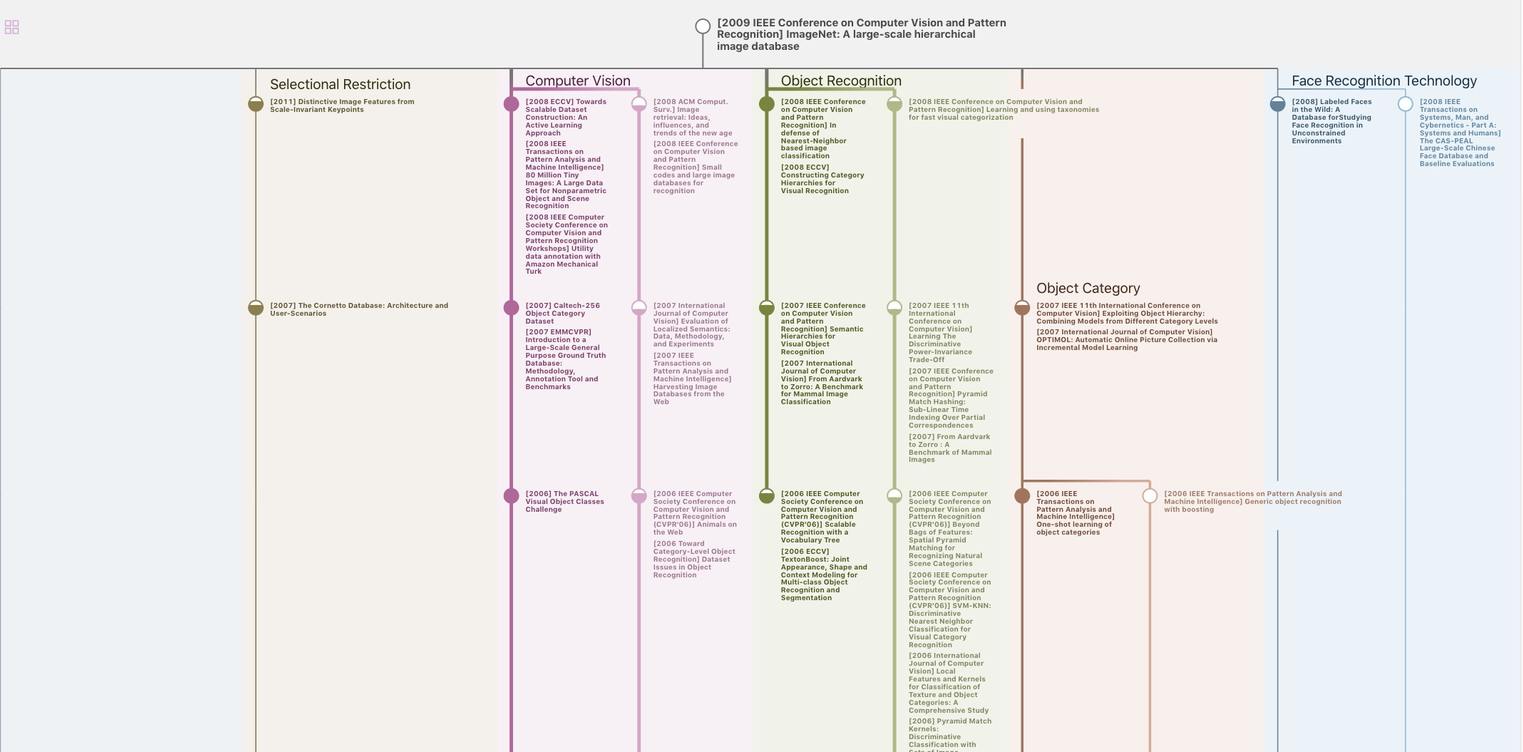
Generate MRT to find the research sequence of this paper
Chat Paper
Summary is being generated by the instructions you defined