Ensemble Learning Using Multivariate Variational Mode Decomposition Based on the Transformer for Multistep-Ahead Streamflow Forecasting
JOURNAL OF HYDROLOGY(2024)
关键词
Streamflow forecasting,Ensemble learning,Multivariate variational mode decomposition,Transformer
AI 理解论文
溯源树
样例
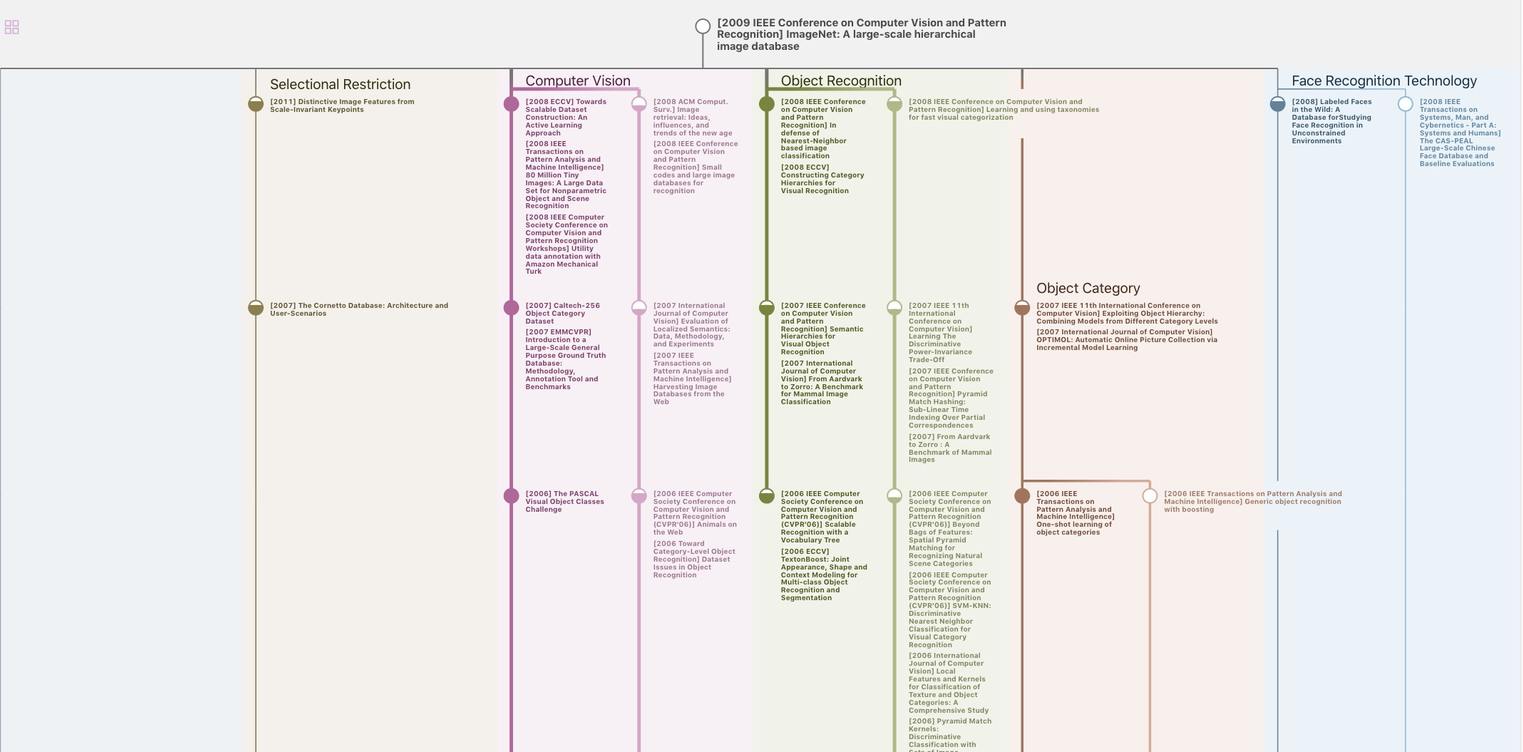
生成溯源树,研究论文发展脉络
Chat Paper
正在生成论文摘要