Unsupervised Representation Learning for 3-Dimensional Magnetic Resonance Imaging Super-Resolution with Degradation Adaptation
IEEE Transactions on Artificial Intelligence(2024)
摘要
High-resolution (HR) magnetic resonance imaging is essential in aiding doctors in their diagnoses and image-guided treatments. However, acquiring HR images can be time-consuming and costly. Consequently, deep learning-based super-resolution reconstruction (SRR) has emerged as a promising solution for generating super-resolution (SR) images from low-resolution (LR) images. Unfortunately, training such neural networks requires aligned authentic HR and LR image pairs, which are challenging to obtain due to patient movements during and between image acquisitions. While rigid movements of hard tissues can be corrected with image registration, aligning deformed soft tissues is complex, making it impractical to train neural networks with authentic HR and LR image pairs. Previous studies have focused on SRR using authentic HR images and downsampled synthetic LR images. However, the difference in degradation representations between synthetic and authentic LR images suppresses the quality of SR images reconstructed from authentic LR images. To address this issue, we propose a novel Unsupervised Degradation Adaptation Network (UDEAN). Our network consists of a degradation learning network and an SRR network. The degradation learning network downsamples HR images using the degradation representation learned from misaligned or unpaired LR images. The SRR network then learns to map the downsampled HR images to the original ones. Experimental results show that our method outperforms state-of-the-art networks with an improvement of up to 0.051/3.52 dB in SSIM/PSNR on two public datasets, thus is a promising solution to the challenges in clinical settings.
更多查看译文
关键词
3D Super-Resolution,Degradation Adaptation,Geometric Deformation,Magnetic Resonance Imaging,Unsupervised Learning
AI 理解论文
溯源树
样例
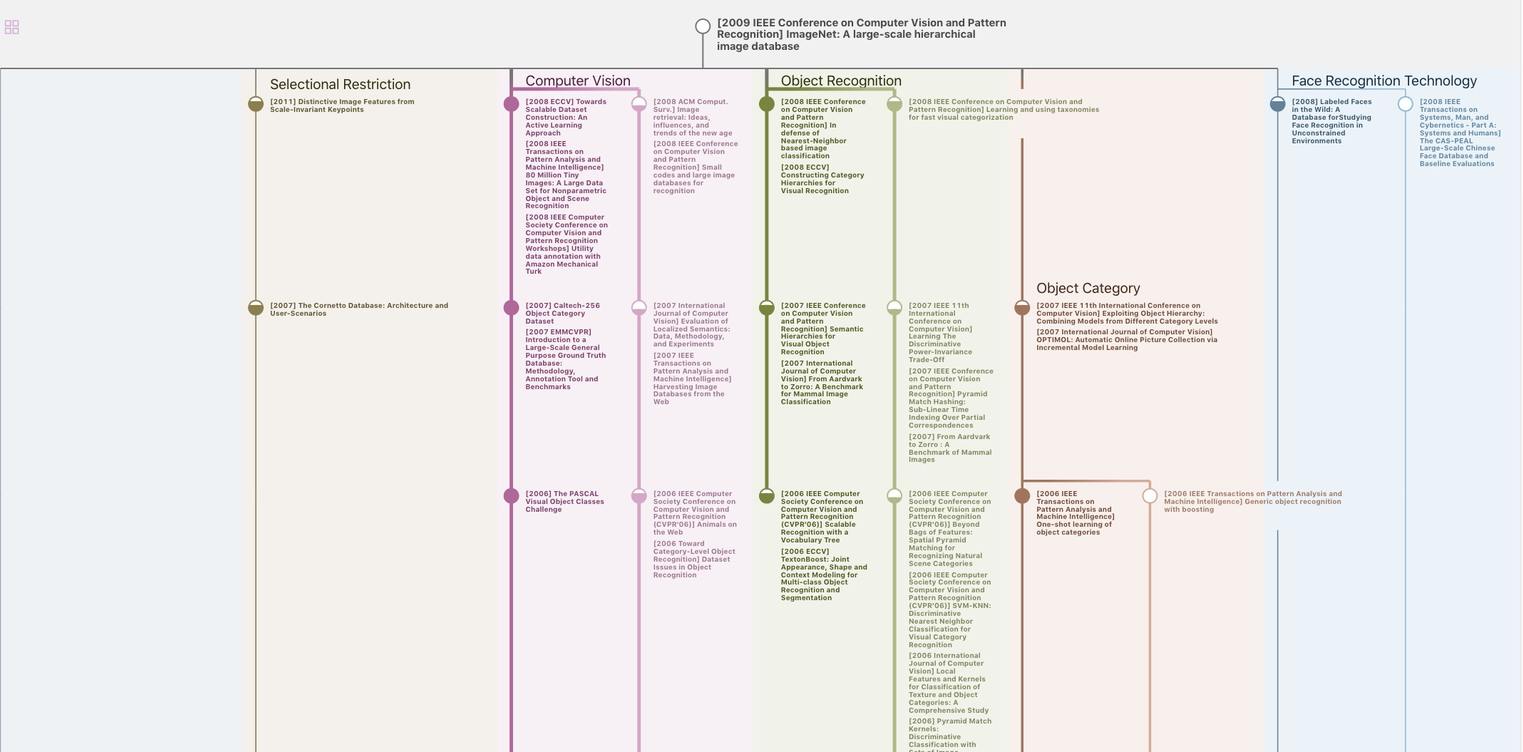
生成溯源树,研究论文发展脉络
Chat Paper
正在生成论文摘要