NeuroDM: Decoding and visualizing human brain activity with EEG-guided diffusion model
Computer Methods and Programs in Biomedicine(2024)
摘要
Background and Objective:
Brain-Computer Interface (BCI) technology has recently been advancing rapidly, bringing significant hope for improving human health and quality of life. Decoding and visualizing visually evoked electroencephalography (EEG) signals into corresponding images plays a crucial role in the practical application of BCI technology. The recent emergence of diffusion models provides a good modeling basis for this work. However, the existing diffusion models still have great challenges in generating high-quality images from EEG, due to the low signal-to-noise ratio and strong randomness of EEG signals. The purpose of this study is to address the above-mentioned challenges by proposing a framework named NeuroDM that can decode human brain responses to visual stimuli from EEG-recorded brain activity.
Methods:
In NeuroDM, an EEG-Visual-Transformer (EV-Transformer) is used to extract the visual-related features with high classification accuracy from EEG signals, then an EEG-Guided Diffusion Model (EG-DM) is employed to synthesize high-quality images from the EEG visual-related features.
Results:
We conducted experiments on two EEG datasets (one is a forty-class dataset, and the other is a four-class dataset). In the task of EEG decoding, we achieved average accuracies of 99.80% and 92.07% on two datasets, respectively. In the task of EEG visualization, the Inception Score of the images generated by NeuroDM reached 15.04 and 8.67, respectively. All the above results outperform existing methods.
Conclusions:
The experimental results on two EEG datasets demonstrate the effectiveness of the NeuroDM framework, achieving state-of-the-art performance in terms of classification accuracy and image quality. Furthermore, our NeuroDM exhibits strong generalization capabilities and the ability to generate diverse images.
更多查看译文
关键词
Electroencephalography,Diffusion model,Feature extraction,Image generation
AI 理解论文
溯源树
样例
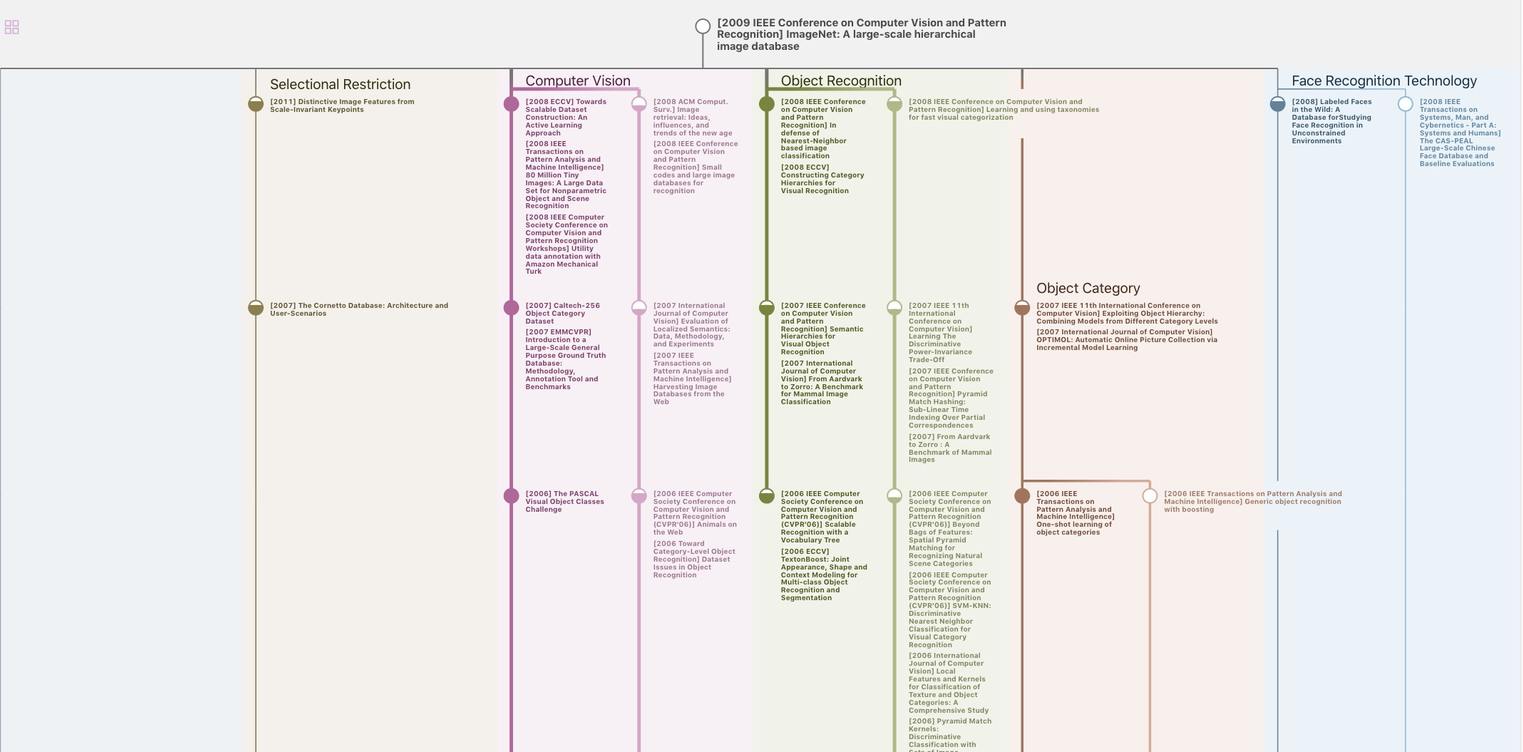
生成溯源树,研究论文发展脉络
Chat Paper
正在生成论文摘要