Similar modality completion-based multimodal sentiment analysis under uncertain missing modalities
Information Fusion(2024)
摘要
Recently, uncertain missing modalities in multimodal sentiment analysis (MSA) brings a new challenge for sentiment analysis. However, existing research cannot accurately complete the missing modalities, and fail to explore the advantages of the text modality in MSA. For the above problems, this work develops a Similar Modality Completion based-MSA model under uncertain missing modalities (termed as SMCMSA). Firstly, we construct the full modalities samples database (FMSD) by screening out the full modality samples from the whole multimodal dataset, and then predicting and marking the sentiment labels of each modality of the samples with three pre-trained unimodal sentiment analysis model (PTUSA). Next, for completing the uncertain missing modalities, we propose a set of missing modalities completion strategies based on the similar modalities selected from FMSD. For the completed multimodal data, we first encode the text, video and audio modality using the encoder of transformer, then we fuse the representation of text into the representations of video and audio under the guidance of a pre-trained model, thereby improving the quality of video and audio. Finally, we conduct sentiment classification based on the representations of text, video and audio with the softmax function respectively, and get the final decision with the decision-level fusion method. Based on benchmark datasets CMU-MOSI and IEMOCAP, extensive experiments have been conducted to verify that our proposed model SMCMSA has better performance than that of the state-of-the-art baseline models.
更多查看译文
关键词
Multimodal sentiment analysis,Uncertain missing modalities,Similar modality completion,Transformer
AI 理解论文
溯源树
样例
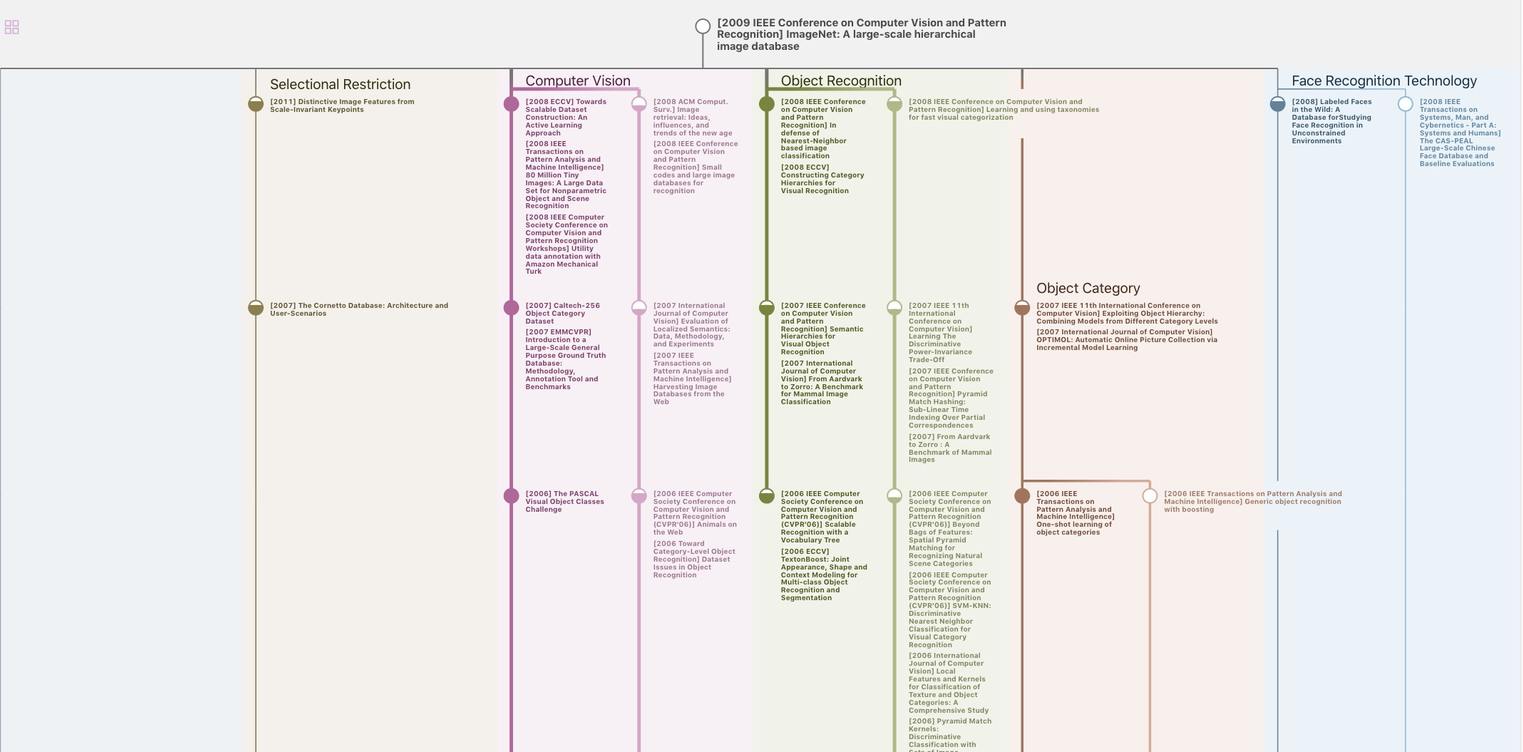
生成溯源树,研究论文发展脉络
Chat Paper
正在生成论文摘要