Learning spatiotemporal relationships with a unified framework for video object segmentation
Applied Intelligence(2024)
摘要
Video object segmentation (VOS) has made significant progress with matching-based methods, but most approaches still show two problems. Firstly, they apply a complicated and redundant two-extractor pipeline to use more reference frames for cues, increasing the models’ parameters and complexity. Secondly, most of these methods neglect the spatial relationships (inside each frame) and do not fully model the temporal relationships (among different frames), i.e., they need adequate modeling of spatial-temporal relationships. In this paper, to address the two problems, we propose a unified transformer-based framework for VOS, a compact and unified single-extractor pipeline with strong spatial and temporal interaction ability. Specifically, to slim the common-used two-extractor pipeline while keeping the model’s effectiveness and flexibility, we design a single dynamic feature extractor with an ingenious dynamic input adapter to encode two significant inputs, i.e., reference sets (historical frames with predicted masks) and query frame (current frame), respectively. Moreover, the relationships among different frames and inside every frame are crucial for this task. We introduce a vision transformer to exploit and model both the temporal and spatial relationships simultaneously. By the cascaded design of the proposed dynamic feature extractor, transformer-based relationship module, and target-enhanced segmentation, our model implements a unified and compact pipeline for VOS. Extensive experiments demonstrate the superiority of our model over state-of-the-art methods on both DAVIS and YouTube-VOS datasets. We also explore potential solutions, such as sequence organizers, to improve the model’s efficiency. On DAVIS17 validation, we achieve ∼ 50 J & F ) drop in segmentation quality. Codes are available at https://github.com/sallymmx/TransVOS.git .
更多查看译文
关键词
Video object segmentation,Transformer,Spatial-temporal,Dynamic feature extractor,Sequence organizers
AI 理解论文
溯源树
样例
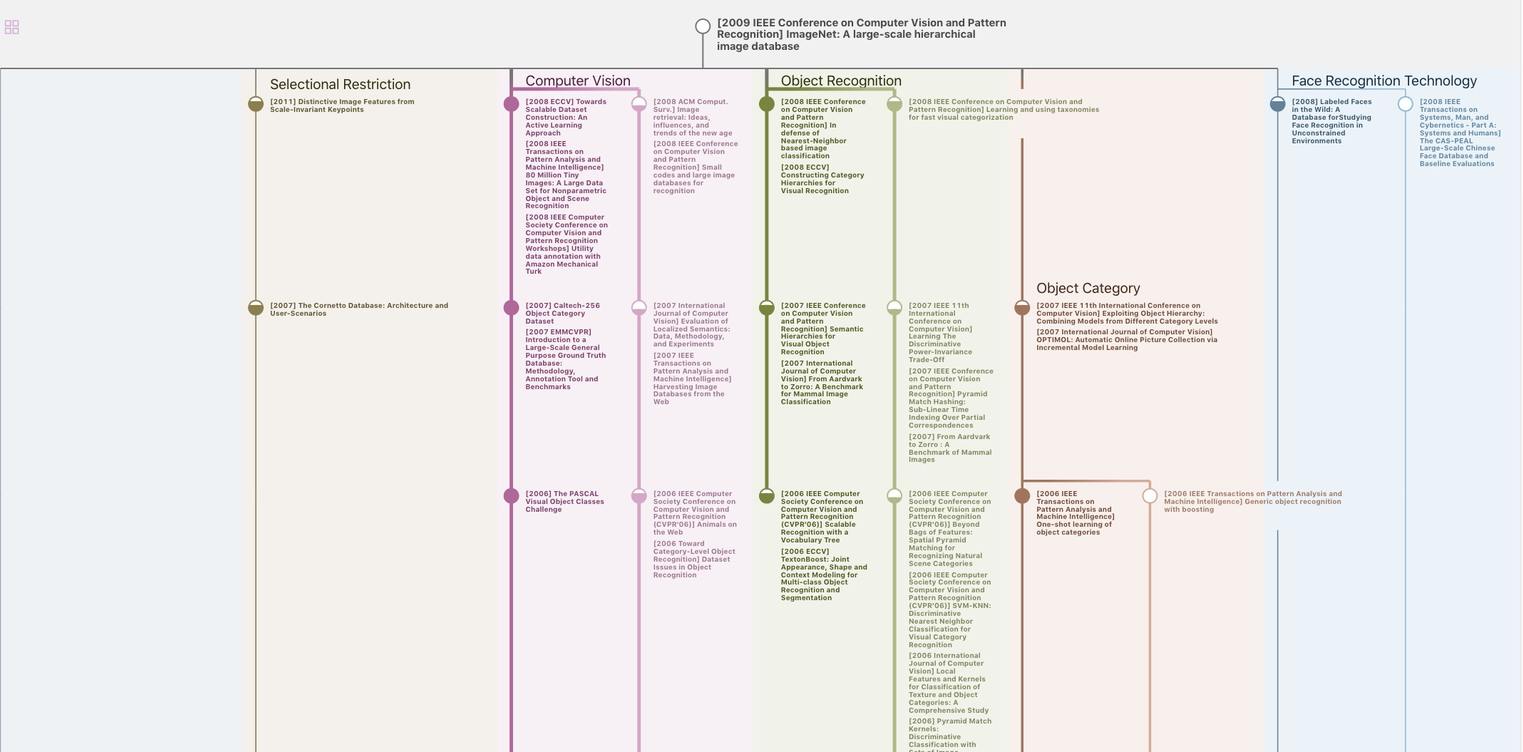
生成溯源树,研究论文发展脉络
Chat Paper
正在生成论文摘要