3D point cloud analysis for surface quality inspection: A steel parts use case
Procedia CIRP(2024)
摘要
A manufacturing process includes inspecting the product to verify it meets its quality standards. Such steps, however, are time-consuming and, depending on the means, prone to errors. If not identified in time, defects occurring at an early step of a manufacturing process may result in significant waste, especially if the product is not easy to re-work. Today, however, the combination of AI with computer vision technologies can enable manufacturers to transform quality inspection by automating the detection of defects. This study discusses the use of products’ 3D shape for inline surface defect detection, facilitating the adoption of proactive control strategies facilitating the reduction of waste. The product's 3D shape, represented by a point cloud is acquired by two fixed laser triangulation sensors orthogonally arranged. The K-means method is adopted for the point cloud data analysis, while Voxel Grid filters are used for downsampling to reduce computational time. The proposed approach has been evaluated in a use case related to the production of steel parts, with the findings supporting that an in-line implementation can facilitate the detection of surface or geometry defects, which, in turn, may facilitate the reduction of waste, by avoiding further processing of the defective product.
更多查看译文
关键词
Machine Learning,Point-cloud,Quality,Sustainable Manufacturing
AI 理解论文
溯源树
样例
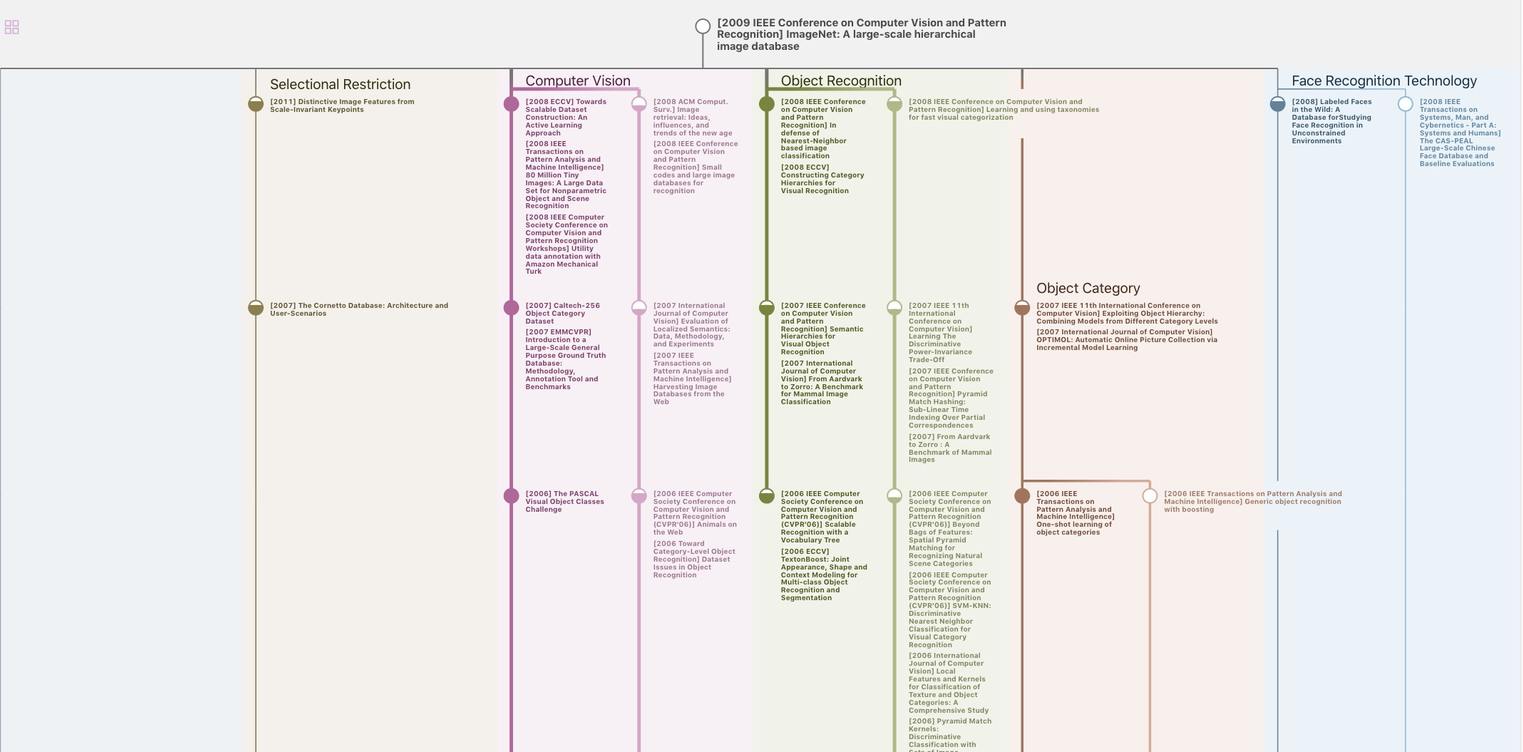
生成溯源树,研究论文发展脉络
Chat Paper
正在生成论文摘要