DSIS-DPR:Structured Instance Segmentation and Diffusion Prior Refinement for Dental Anatomy Learning
IEEE Transactions on Multimedia(2024)
摘要
Instance segmentation in medical imaging plays a crucial role in clinical diagnostic tasks, and have shown promising performance in practical applications. In this paper, we discuss a more fine-grained instance segmentation task: dental structured instance segmentation based on panoramic radiographs. However, direct segmentation of tooth structures encounters inherent challenges. Traditional instance segmentation networks often fall short in capturing intricate internal features, and exacerbated by the frequent blurring found in medical imaging, which can result in the deficiency of anatomical details. To deal with these problems, we propose a novel framework called DSISDPR, which combines a dental structured instance segmentation (DSIS) network with an enhanced diffusion prior refinement (DPR) method. Specifically, our innovatively designed structureaware network leverages fine-grained feature fusion, acquiring a richer representation of internal anatomical structures. With the integration of adversarial learning, the model is primed to deliver holistic and subtle predictions of tooth structures. Furthermore, taking inspiration from dentists’ inherent ability to utilize prior knowledge, such as understanding dental structures to label invisible anatomical structures, we propose a diffusion inpainting to refine the results of DSIS without additional annotations. Equipped with built-in structure learning, DPR is capable of modifying anomalies within each predicted segmentation, resulting in a more robust and complete structured segmentation result. Meanwhile, we ensure rigorous oversight over the reconstruction of areas affected by abnormalities, ensuring that any introduced adjustments minimally disrupt the wellpredicted structured segmentation results. Extensive experiments have demonstrated that our DSIS-DPR outperforms all existing classical instance segmentation networks. The collected dataset is available:
https://github.com/Zzz512/TSD
.
更多查看译文
关键词
Abnormal Detect,Diffusion Inpaint,Dental Panoramic Imaging,Instance Segmentation,Structured Segmentation
AI 理解论文
溯源树
样例
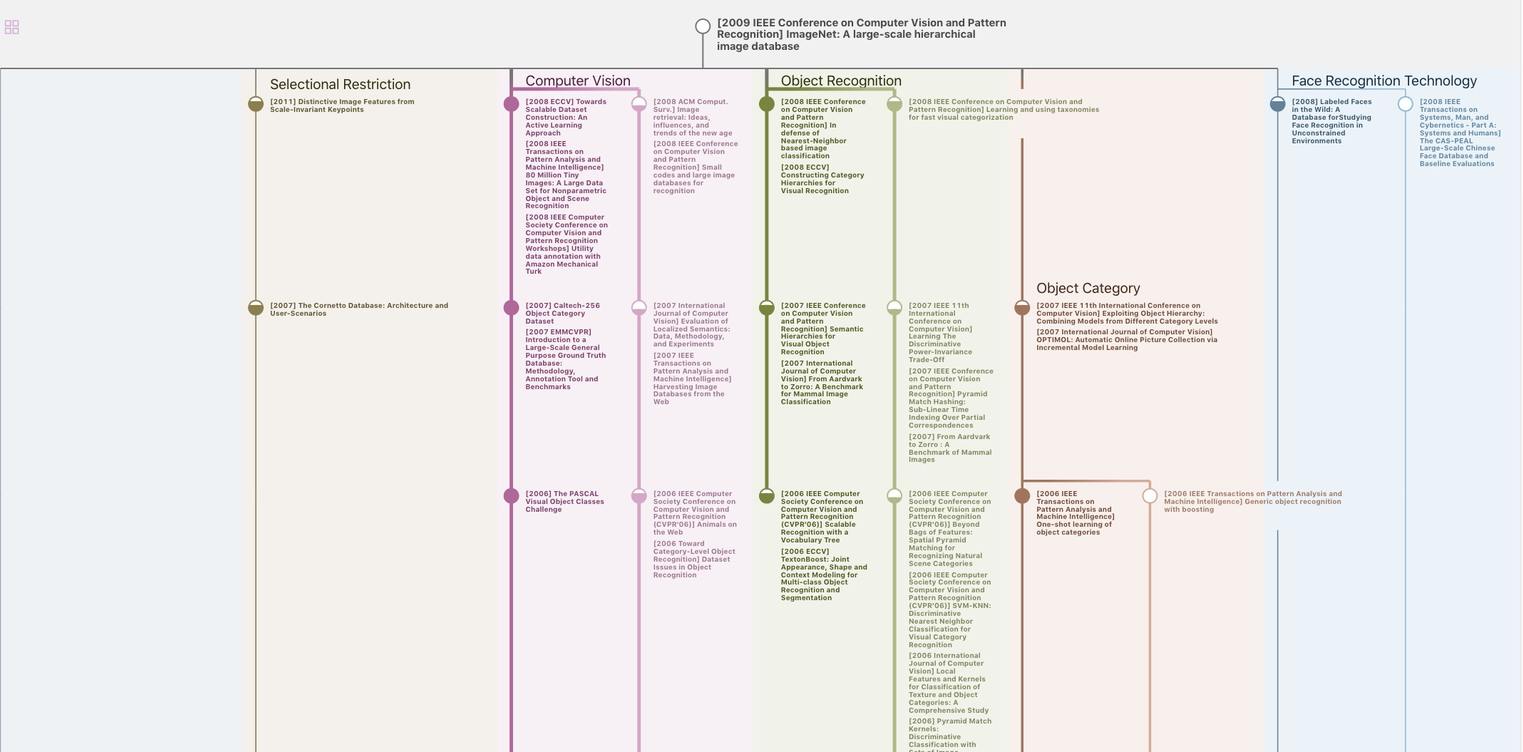
生成溯源树,研究论文发展脉络
Chat Paper
正在生成论文摘要