Evolutionary Computing Empowered Community Detection in Attributed Networks
IEEE COMMUNICATIONS MAGAZINE(2024)
摘要
Discovering communities in attributed networks is an important research topic in complex network analysis. Community detection based on multi-objective evolutionary computing (MOEA) models community detection as a multi-objective optimization problem and searches the optimal solutions by simulating the evolution of a biological population. However, the existing multi-objective evolutionary algorithms for community detection faces two challenges: their encoding schemes are designed based on network topology and neglects the information in node attributes; and they are easy to fall into local optimum. In this article, we propose a community detection algorithm empowered by multi-objective evolutionary computing, named ECEVO-MOEA, which conducts edge closeness encoding and embedding vector optimization alternately. On the one hand, the evolution of a biological population is completed by employing a new edge closeness encoding scheme and multiple attribute-aware objective functions. On the other hand, the update of embedding vectors is used to calculate similarity matrix and communities to improve solution quality, avoiding it from early convergence. Experiments on real networks demonstrate that ECEVO-MOEA achieves higher accuracy than the baseline algorithms.
更多查看译文
关键词
Evolutionary computation,Encoding,Statistics,Social factors,Image edge detection,Search problems,Pareto optimization,Complex networks,Detection algorithms,Community networks
AI 理解论文
溯源树
样例
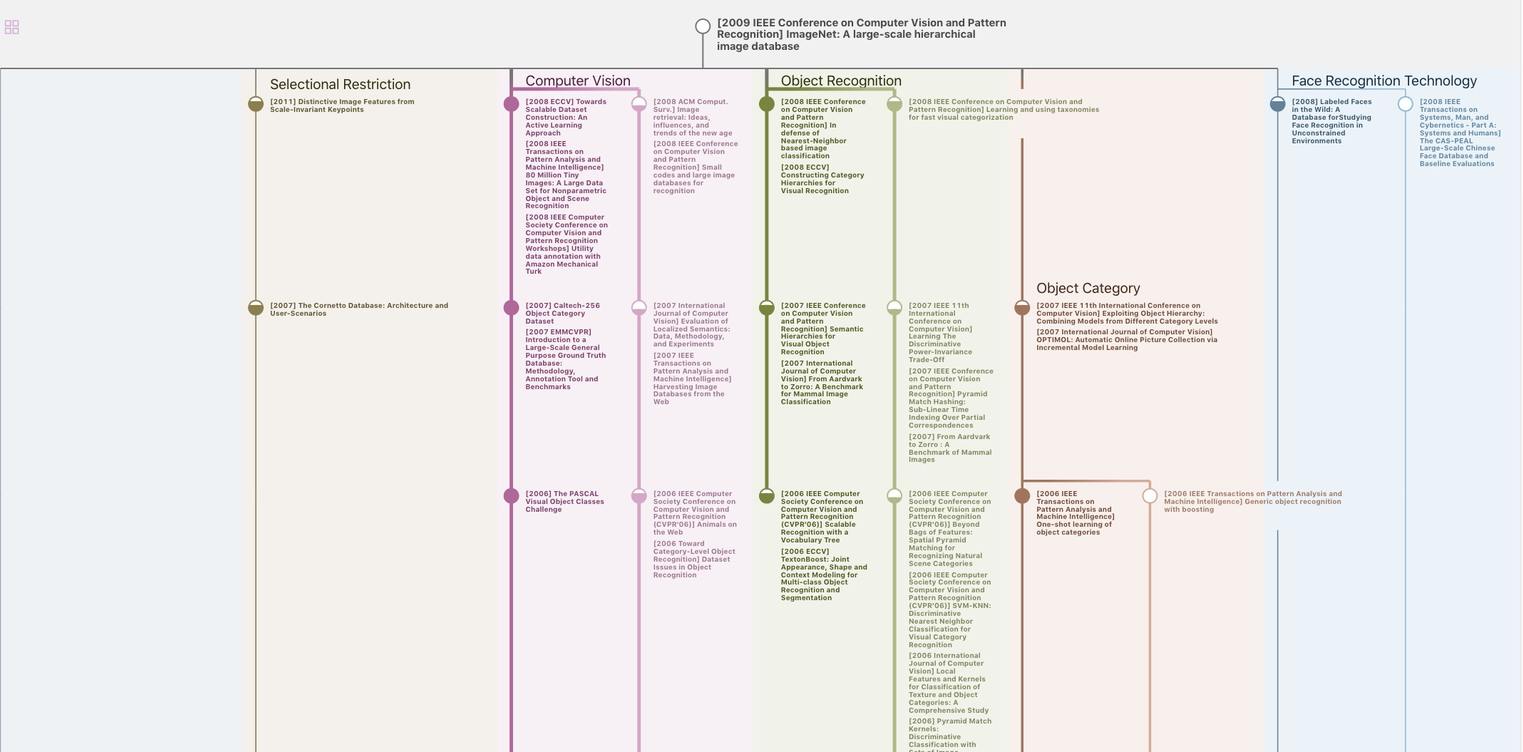
生成溯源树,研究论文发展脉络
Chat Paper
正在生成论文摘要