Sugarcane Leaf Health Grading Using State-of-the-Art Deep Learning Approaches
2024 3rd International Conference for Innovation in Technology (INOCON)(2024)
摘要
The abstract provides a thorough summary of a classification model intended to assess the health characteristics of sugarcane leaves in different classes. The precision values of the model range from 96.93% to 97.40%, which indicates its superior performance metrics and guarantees the precise identification of positive cases inside each class. The recall values show how well the model captures most real positive events while minimizing false negatives, ranging from 96.96% to 97.48%. The precision and recall of an F1-Score are well balanced, as seen by their constant hovering around 97%. A dataset with well-distributed values and percentage of support exhibits a balanced representation across classes. The model, which uses CNNs and SVMs, achieves 87.39% accuracy on 11,133 images. The model successfully classifies sugarcane leaf health with an amazing overall accuracy of 99%, demonstrating its proficiency in this area. The weighted average, micro average, and macro average measures all support the model's consistency and dependability across classes. The class-wise evaluations' unweighted mean, or the macro average, is 97.18%. The model's strong handling of different class sizes is highlighted by the weighted average, which accounts for class imbalances depending on support and is in line with the macro average of 97.19%. The global aggregate provided by the micro average for all classes is in perfect alignment with the class-specific measures, indicating the overall superiority of the model. Together, the findings establish the classification model as a potent and trustworthy instrument with excellent accuracy and precision across a range of health categories for evaluating sugarcane leaf health.
更多查看译文
关键词
Precision,Health,Research,Class
AI 理解论文
溯源树
样例
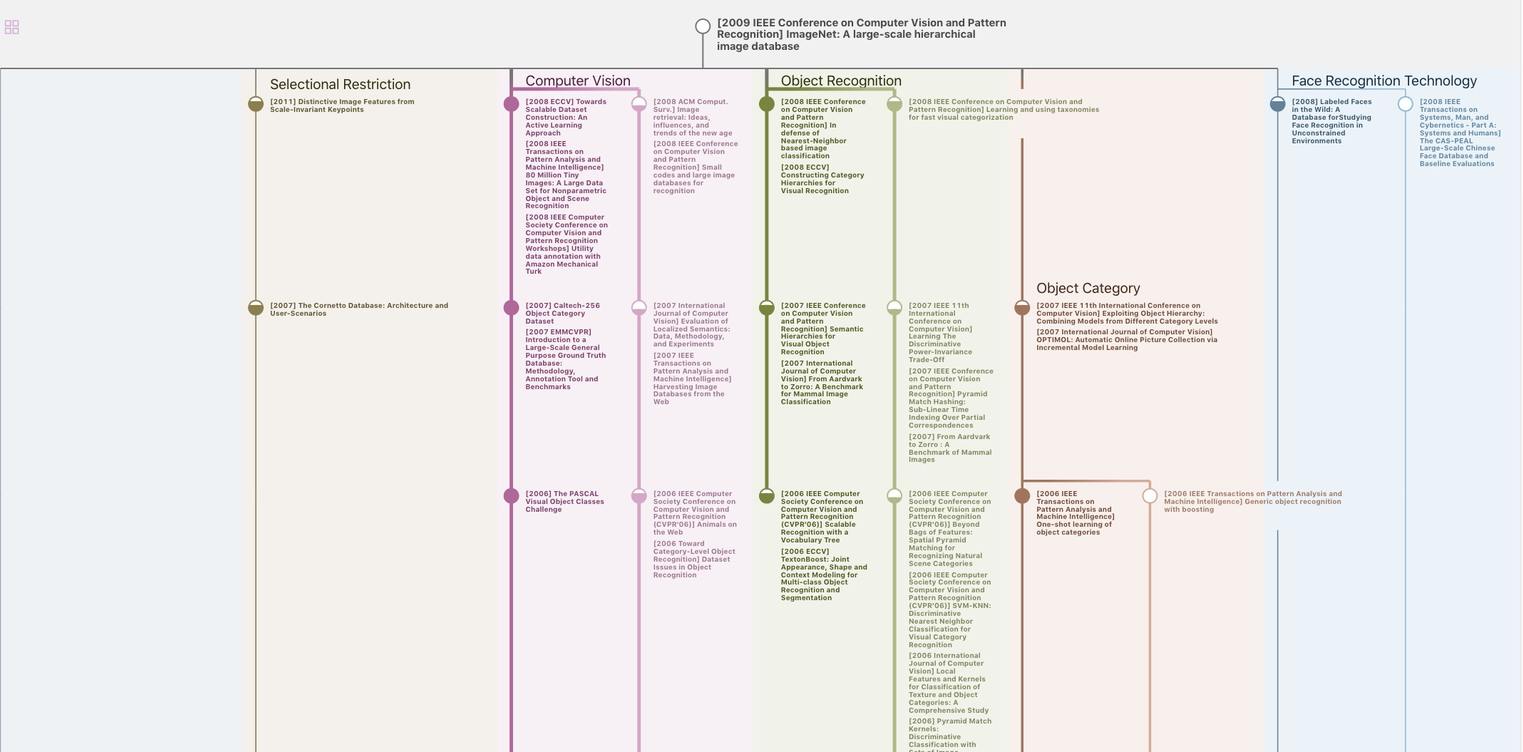
生成溯源树,研究论文发展脉络
Chat Paper
正在生成论文摘要