Inversion of Aerosol Optical Depth: Incorporating Multi-Model Approach
IEEE Transactions on Geoscience and Remote Sensing(2024)
摘要
Atmospheric aerosols originate from diverse sources and exert a notable influence on the radiation budget, atmospheric environment, and human health. However, current aerosol inversion models still have limitations in dealing with multiple types of variables and intricate scenarios, for which a two-stage hybrid model named CNNRF is proposed in this study. Convolution is employed to extract continuous spectral signals. The study takes into account the synergistic impact of spatio-temporal, meteorological, and surface information. Ensemble learning is then applied to adeptly handle diverse independent input variables. In this paper, eight regions were selected globally for modelling and testing based on different scenario types. Additionally, an aerosol hotspot region (India) was chosen for independent experiments. Employing a 10-fold cross-validation (CV) method and cross-comparison with the MCD19A2 and CNN, RF models. The sample-based CV of the CNNRF model demonstrates high and consistent accuracy, with a Pearson correlation coefficient (R) value of 0.958, mean absolute error (MAE) of 0.048, and an within expected error (EE) envelope of 87.15%. For the Indian region, the MAE and EE are 0.05 and 95.8%, respectively. In summary, the proposed hybrid model demonstrates robust generalization capabilities, enabling accurate and stable aerosols estimation on a global scale.
更多查看译文
关键词
Aerosol retrieval,A two-stage hybrid model,Convolution,Ensemble learning,Moderate Resolution Imaging Spectroradiometer (MODIS)
AI 理解论文
溯源树
样例
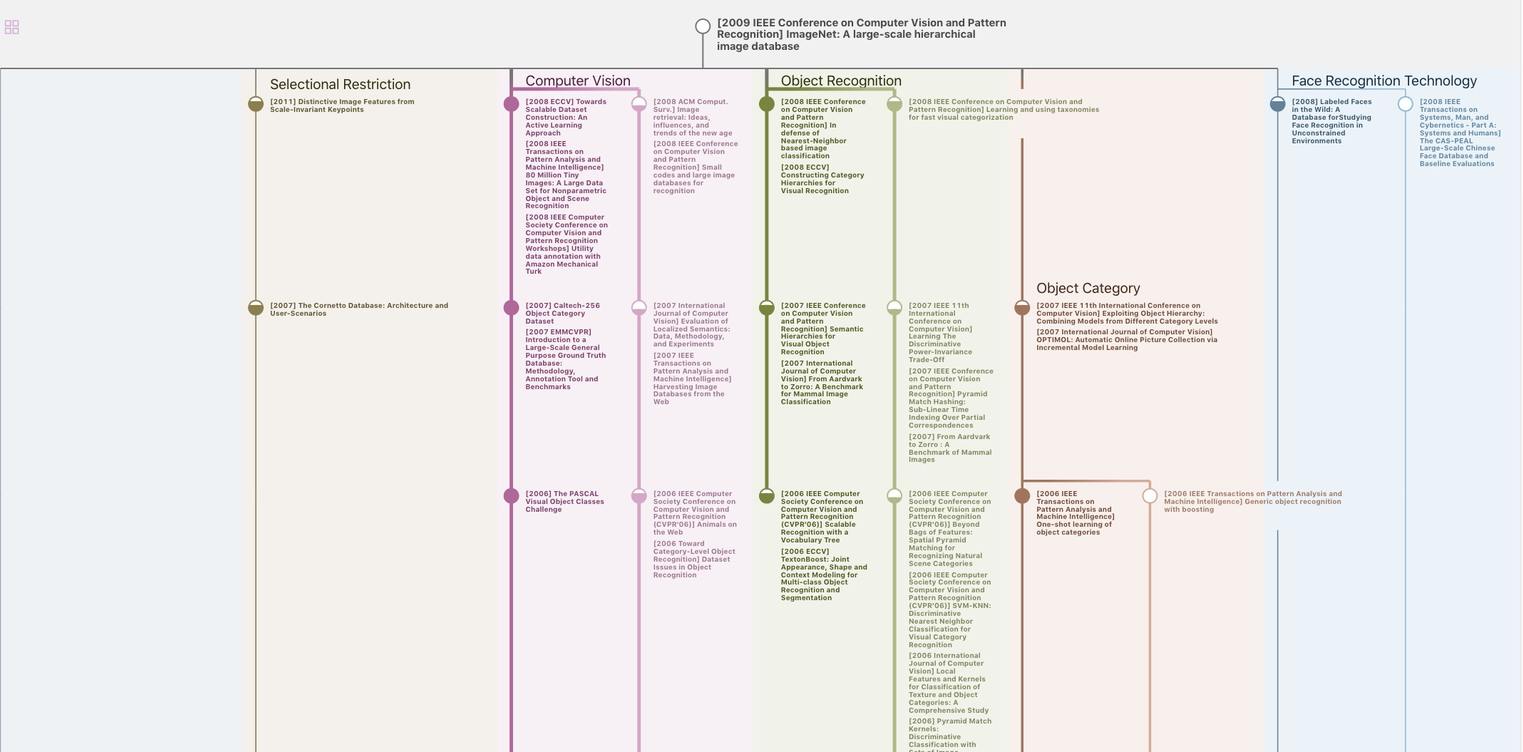
生成溯源树,研究论文发展脉络
Chat Paper
正在生成论文摘要