Differential neural network robust constrained controller using approximate dynamic programming
European Journal of Control(2024)
Abstract
This study focuses on developing a continuous differential neural network (DNN) approximating min–max robust control by applying dynamic neural programming. The suggested controller is applied to a class of nonlinear perturbed systems providing satisfactory dynamics for a given cost function depending on both the trajectories of the perturbed system and the designed constrained control actions. The min–max formulation for dynamic programming offers reliable control for restricted modeling uncertainties and perturbations. The suggested design considers control norm restrictions in the optimization problem. DNN’s approximation of the worst (with respect to the admissible class of perturbations and uncertainties) value function of the Hamilton–Jacobi-Bellman (HJB) equation enables the estimation of the closed-loop formulation of the controller. The robust version of the HJB partial differential equation is studied to create the learning law class for the time-varying weights in the DNN. The controller employs a time-varying Lyapunov-like differential equation and the solution of the corresponding learning laws. A recurrent algorithm based on the Kiefer-Wolfowitz technique can be used by modifying the weights’ initial conditions to fulfill the specified cost function’s end requirements. A numerical example tests the robust control proposed in this study, validating the robust optimal solution based on the DNN approximation for Bellman’s value function.
MoreTranslated text
Key words
Min–max optimal control,System with uncertain model,Differential neural network,Neural network approximation,Lagrange multipliers
AI Read Science
Must-Reading Tree
Example
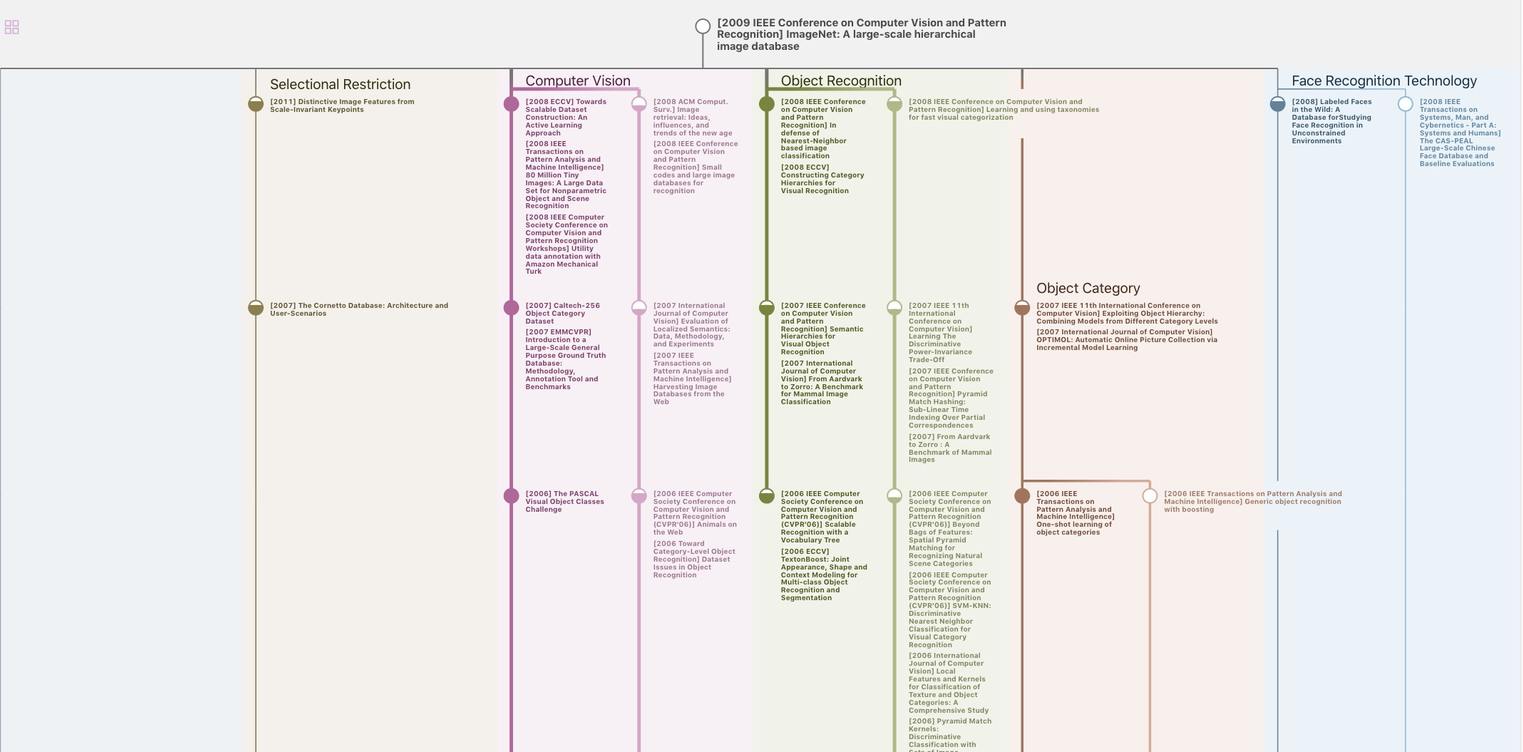
Generate MRT to find the research sequence of this paper
Chat Paper
Summary is being generated by the instructions you defined