Leveraging the spatio-temporal coherence of DAS data for detection and classification
crossref(2024)
摘要
Distributed Acoustic Sensing (DAS) enables the recording of seismic wavefields with an unprecedented spatial resolution, of the order of several metres, and with a sampling frequency that is uniform in both time and space. In spite of these unique characteristics, DAS data are commonly processed trace-by-trace, essentially treating the instrument as a set of conventional seismometers. Hence, one can imagine that such processing routines do not achieve their full potential by not considering the spatial dimension of DAS. To address this, we propose detection and classification methods leveraging the spatio-temporal coherence of the data, encoded in the form of covariance matrices. Through the use of Riemannian geometry, we propose a framework for change-point detection in a stream of such covariance matrices, detecting signals based on their coherence rather than their energy. We extend this framework to facilitate clustering and classification, opening the door to unsupervised data exploration. We apply these methods to borehole and offshore DAS data, and we conclude with an outlook on how this framework can be merged with Deep Learning methods.
更多查看译文
AI 理解论文
溯源树
样例
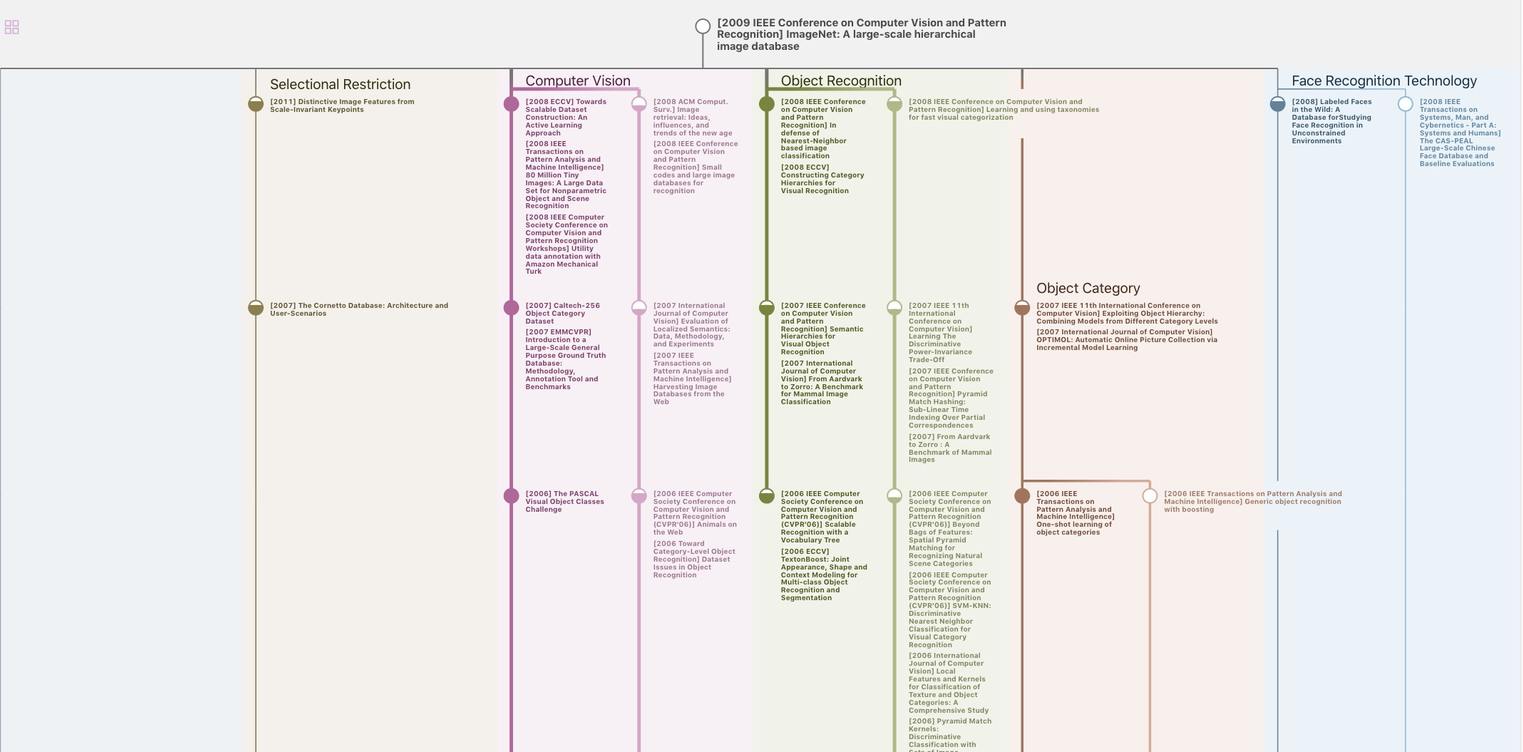
生成溯源树,研究论文发展脉络
Chat Paper
正在生成论文摘要