Operator learning for homogenizing hyperelastic materials, without PDE data
Mechanics Research Communications(2024)
Abstract
In this work, we address operator learning for stochastic homogenization in nonlinear elasticity. A Fourier neural operator is employed to learn the map between the input field describing the material at fine scale and the deformation map. We propose a variationally-consistent loss function that does not involve solution field data. The methodology is tested on materials described either by piecewise constant fields at microscale, or by random fields at mesoscale. High prediction accuracy is obtained for both the solution field and the homogenized response. We show, in particular, that the accuracy achieved with the proposed strategy is comparable to that obtained with the conventional data-driven training method.
MoreTranslated text
Key words
Computational homogenization,Hyperelastic materials,Multiscale analysis,Operator learning
AI Read Science
Must-Reading Tree
Example
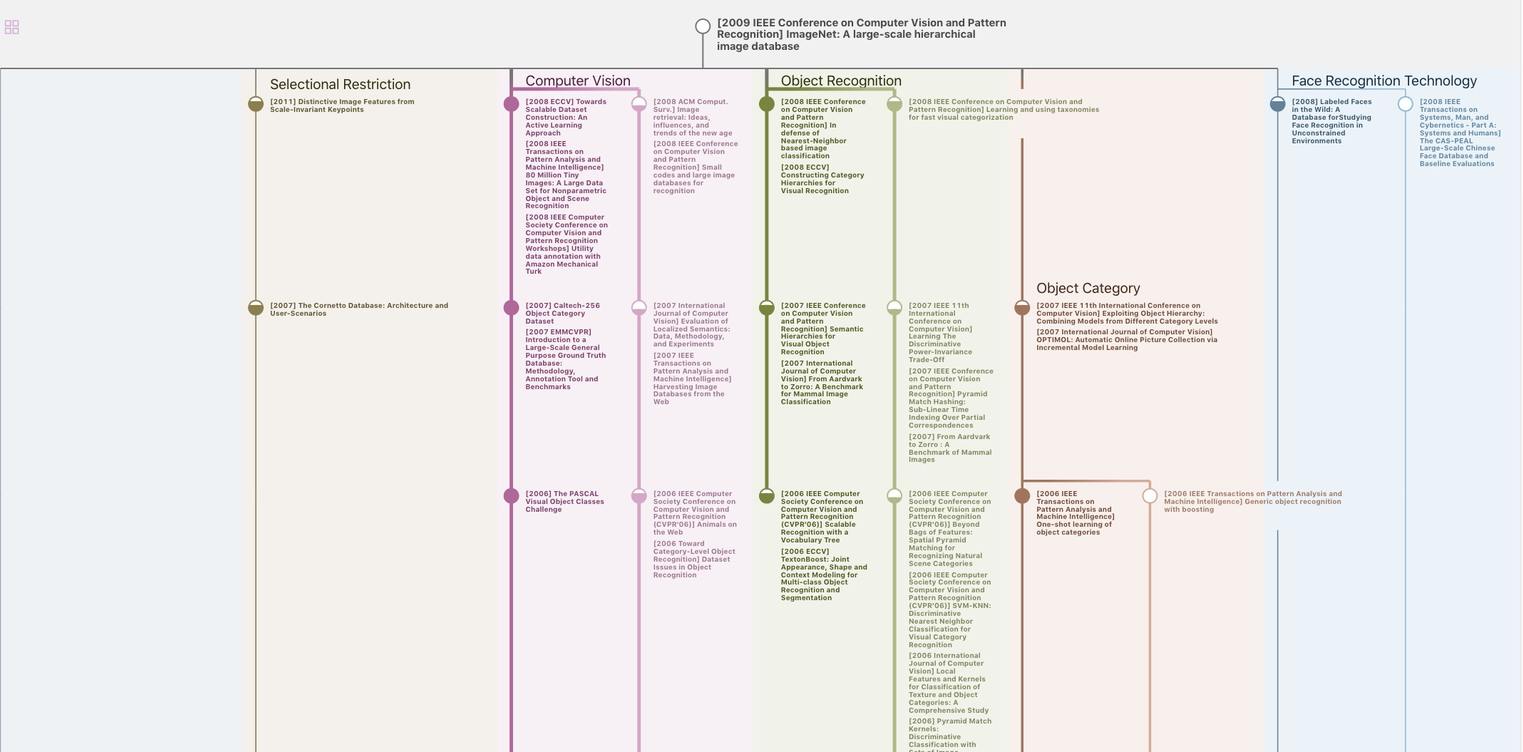
Generate MRT to find the research sequence of this paper
Chat Paper
Summary is being generated by the instructions you defined