Exploring Local Interpretability in Dimensionality Reduction: Analysis and Use Cases
Expert Systems with Applications(2024)
关键词
Dimensionality reduction,Interpretability,Local,Model-agnostic
AI 理解论文
溯源树
样例
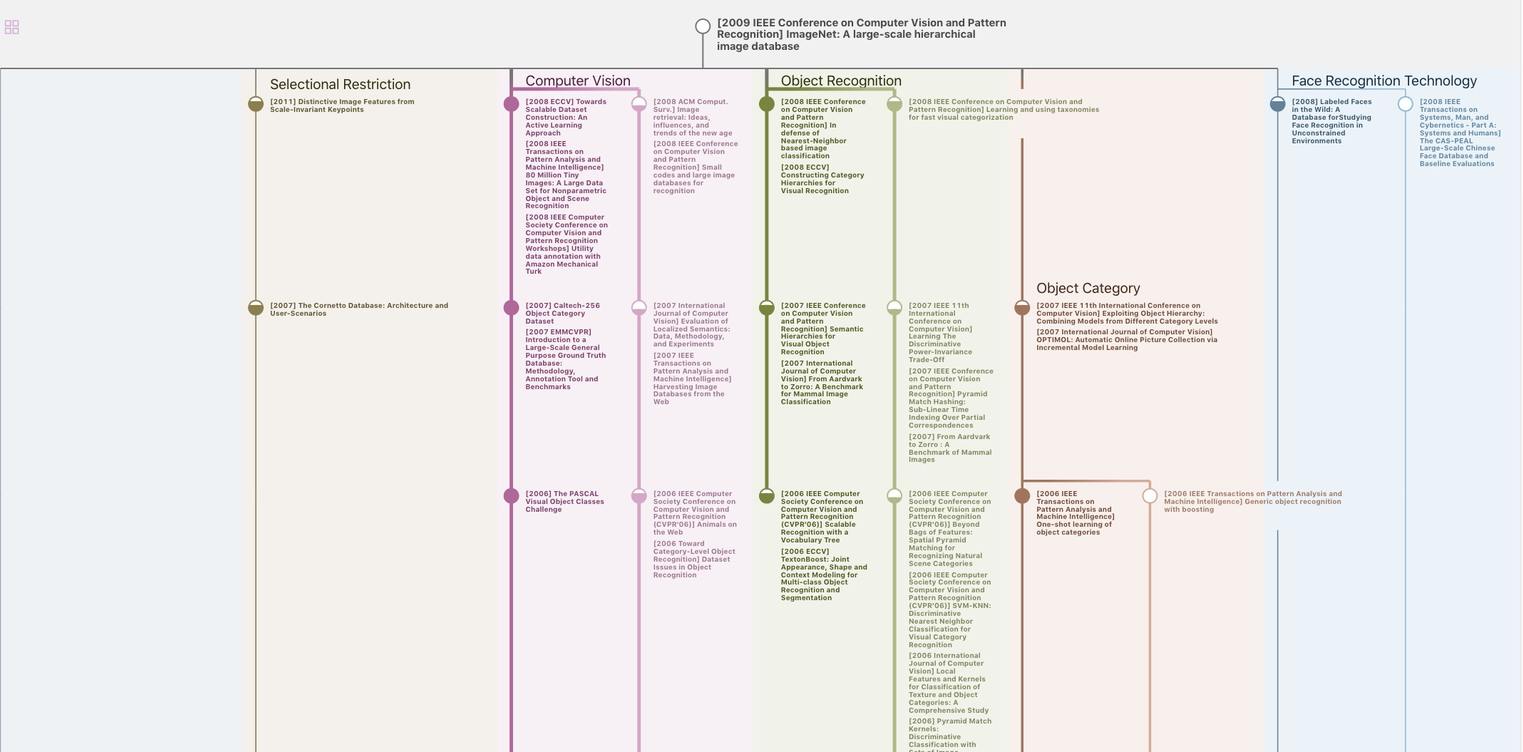
生成溯源树,研究论文发展脉络
Chat Paper
正在生成论文摘要
Expert Systems with Applications(2024)