Provably Unlearnable Examples
arxiv(2024)
摘要
The exploitation of publicly accessible data has led to escalating concerns
regarding data privacy and intellectual property (IP) breaches in the age of
artificial intelligence. As a strategy to safeguard both data privacy and
IP-related domain knowledge, efforts have been undertaken to render shared data
unlearnable for unauthorized models in the wild. Existing methods apply
empirically optimized perturbations to the data in the hope of disrupting the
correlation between the inputs and the corresponding labels such that the data
samples are converted into Unlearnable Examples (UEs). Nevertheless, the
absence of mechanisms that can verify how robust the UEs are against unknown
unauthorized models and train-time techniques engenders several problems.
First, the empirically optimized perturbations may suffer from the problem of
cross-model generalization, which echoes the fact that the unauthorized models
are usually unknown to the defender. Second, UEs can be mitigated by train-time
techniques such as data augmentation and adversarial training. Furthermore, we
find that a simple recovery attack can restore the clean-task performance of
the classifiers trained on UEs by slightly perturbing the learned weights. To
mitigate the aforementioned problems, in this paper, we propose a mechanism for
certifying the so-called (q, η)-Learnability of an unlearnable dataset via
parametric smoothing. A lower certified (q, η)-Learnability indicates a
more robust protection over the dataset. Finally, we try to 1) improve the
tightness of certified (q, η)-Learnability and 2) design Provably
Unlearnable Examples (PUEs) which have reduced (q, η)-Learnability.
According to experimental results, PUEs demonstrate both decreased certified
(q, η)-Learnability and enhanced empirical robustness compared to existing
UEs.
更多查看译文
AI 理解论文
溯源树
样例
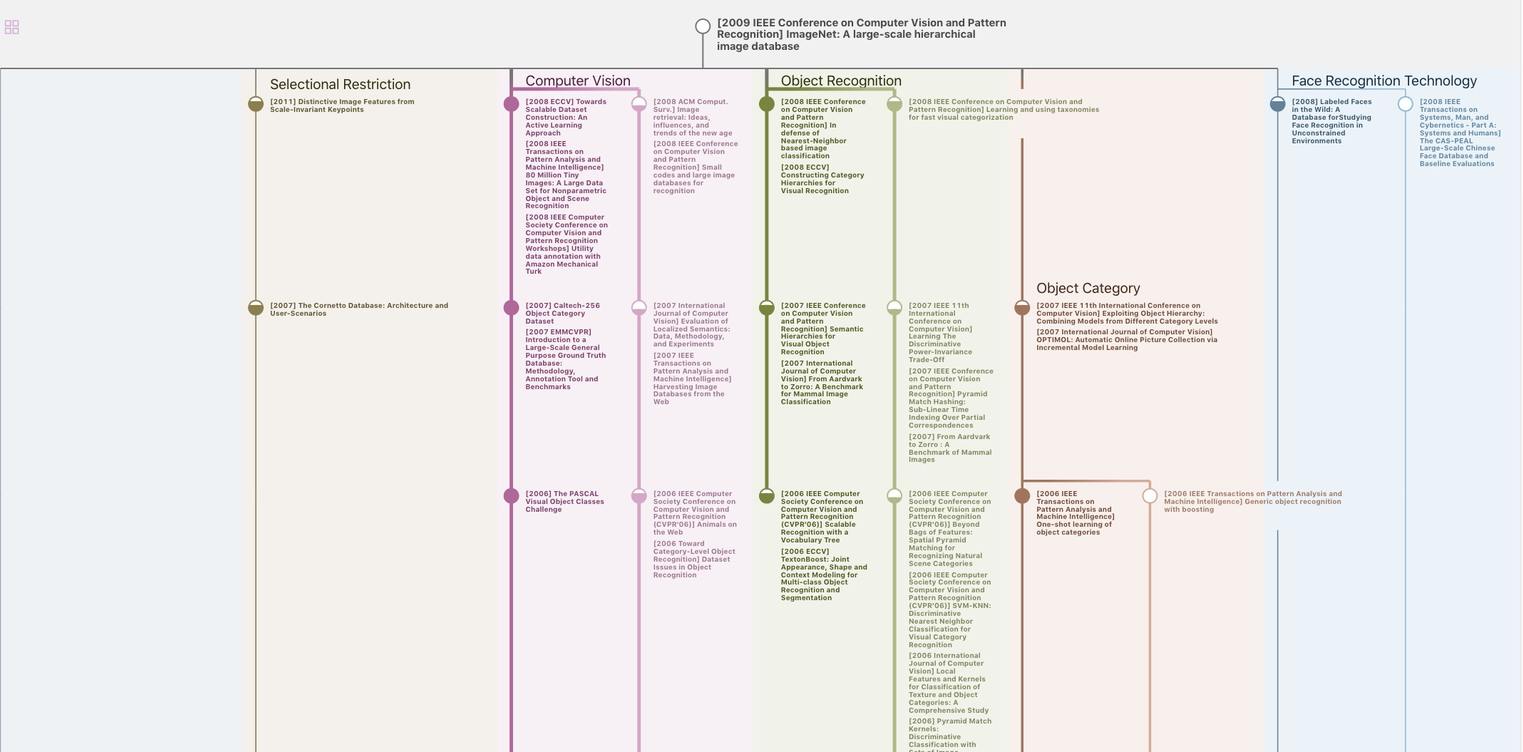
生成溯源树,研究论文发展脉络
Chat Paper
正在生成论文摘要