Advancing Multimodal Medical Capabilities of Gemini
arxiv(2024)
摘要
Many clinical tasks require an understanding of specialized data, such as
medical images and genomics, which is not typically found in general-purpose
large multimodal models. Building upon Gemini's multimodal models, we develop
several models within the new Med-Gemini family that inherit core capabilities
of Gemini and are optimized for medical use via fine-tuning with 2D and 3D
radiology, histopathology, ophthalmology, dermatology and genomic data.
Med-Gemini-2D sets a new standard for AI-based chest X-ray (CXR) report
generation based on expert evaluation, exceeding previous best results across
two separate datasets by an absolute margin of 1
AI reports on normal cases, and 43
"equivalent or better" than the original radiologists' reports. We demonstrate
the first ever large multimodal model-based report generation for 3D computed
tomography (CT) volumes using Med-Gemini-3D, with 53
clinically acceptable, although additional research is needed to meet expert
radiologist reporting quality. Beyond report generation, Med-Gemini-2D
surpasses the previous best performance in CXR visual question answering (VQA)
and performs well in CXR classification and radiology VQA, exceeding SoTA or
baselines on 17 of 20 tasks. In histopathology, ophthalmology, and dermatology
image classification, Med-Gemini-2D surpasses baselines across 18 out of 20
tasks and approaches task-specific model performance. Beyond imaging,
Med-Gemini-Polygenic outperforms the standard linear polygenic risk score-based
approach for disease risk prediction and generalizes to genetically correlated
diseases for which it has never been trained. Although further development and
evaluation are necessary in the safety-critical medical domain, our results
highlight the potential of Med-Gemini across a wide range of medical tasks.
更多查看译文
AI 理解论文
溯源树
样例
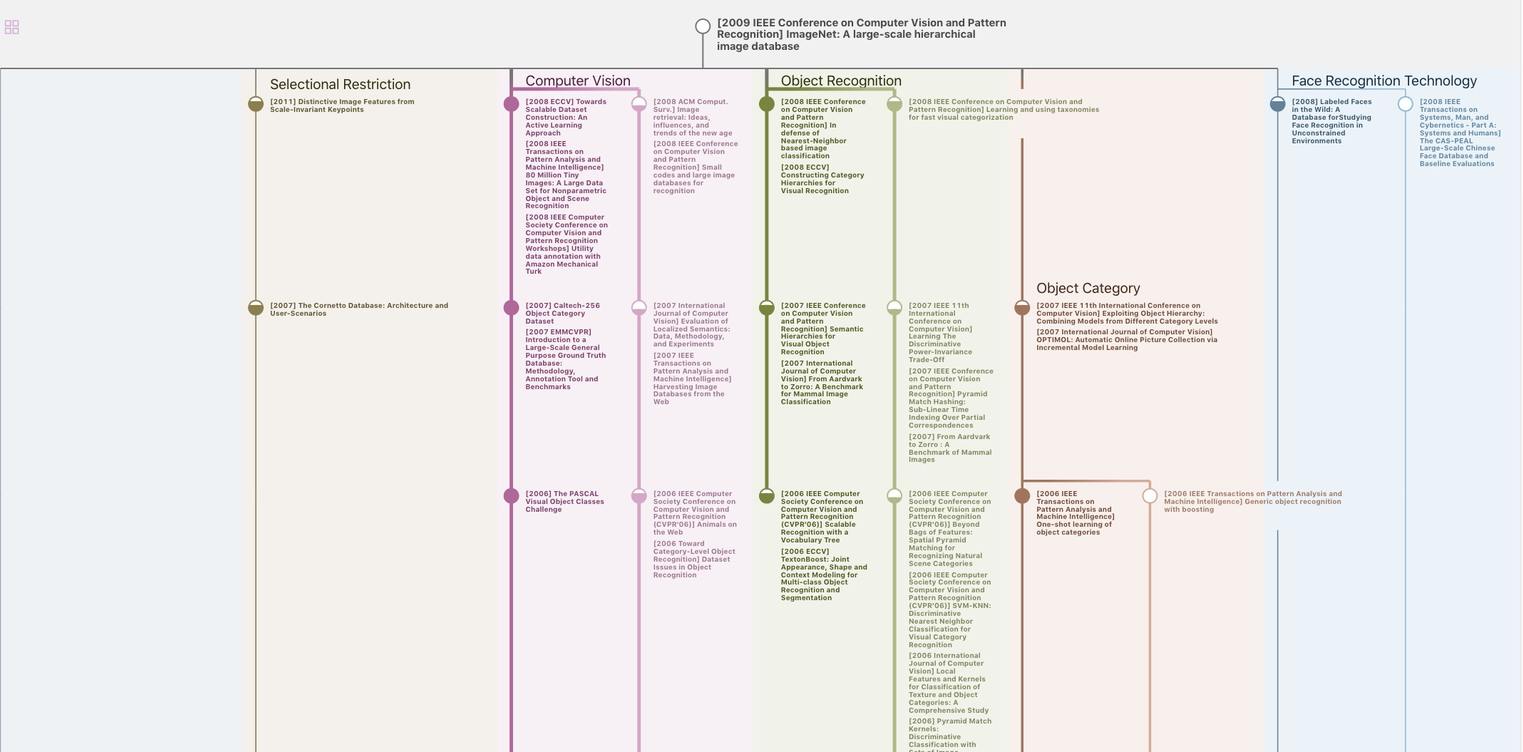
生成溯源树,研究论文发展脉络
Chat Paper
正在生成论文摘要