Neural Network Enhanced Single-Photon Fock State Tomography
arxiv(2024)
摘要
Even though heralded single-photon sources have been generated routinely
through the spontaneous parametric down conversion, vacuum and multiple photon
states are unavoidably involved. With machine-learning, we report the
experimental implementation of single-photon quantum state tomography by
directly estimating target parameters. Compared to the Hanbury Brown and Twiss
(HBT) measurements only with clicked events recorded, our neural network
enhanced quantum state tomography characterizes the photon number distribution
for all possible photon number states from the balanced homodyne detectors. By
using the histogram-based architecture, a direct parameter estimation on the
negativity in Wigner's quasi-probability phase space is demonstrated. Such a
fast, robust, and precise quantum state tomography provides us a crucial
diagnostic toolbox for the applications with single-photon Fock states and
other non-Gaussisan quantum states.
更多查看译文
AI 理解论文
溯源树
样例
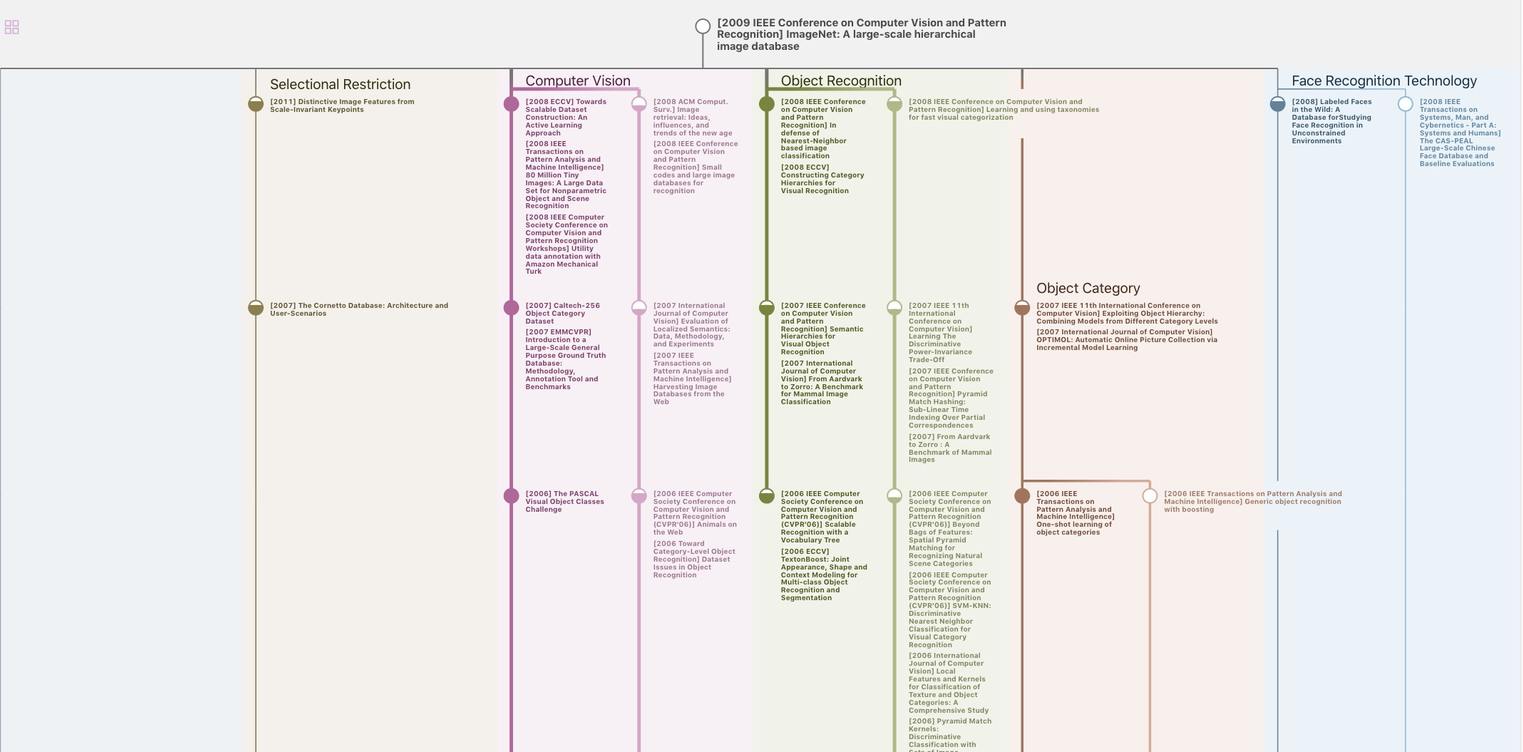
生成溯源树,研究论文发展脉络
Chat Paper
正在生成论文摘要