Artificial-Intelligence-Driven Shot Reduction in Quantum Measurement
arxiv(2024)
Abstract
Variational Quantum Eigensolver (VQE) provides a powerful solution for
approximating molecular ground state energies by combining quantum circuits and
classical computers. However, estimating probabilistic outcomes on quantum
hardware requires repeated measurements (shots), incurring significant costs as
accuracy increases. Optimizing shot allocation is thus critical for improving
the efficiency of VQE. Current strategies rely heavily on hand-crafted
heuristics requiring extensive expert knowledge. This paper proposes a
reinforcement learning (RL) based approach that automatically learns shot
assignment policies to minimize total measurement shots while achieving
convergence to the minimum of the energy expectation in VQE. The RL agent
assigns measurement shots across VQE optimization iterations based on the
progress of the optimization. This approach reduces VQE's dependence on static
heuristics and human expertise. When the RL-enabled VQE is applied to a small
molecule, a shot reduction policy is learned. The policy demonstrates
transferability across systems and compatibility with other wavefunction
ansatzes. In addition to these specific findings, this work highlights the
potential of RL for automatically discovering efficient and scalable quantum
optimization strategies.
MoreTranslated text
AI Read Science
Must-Reading Tree
Example
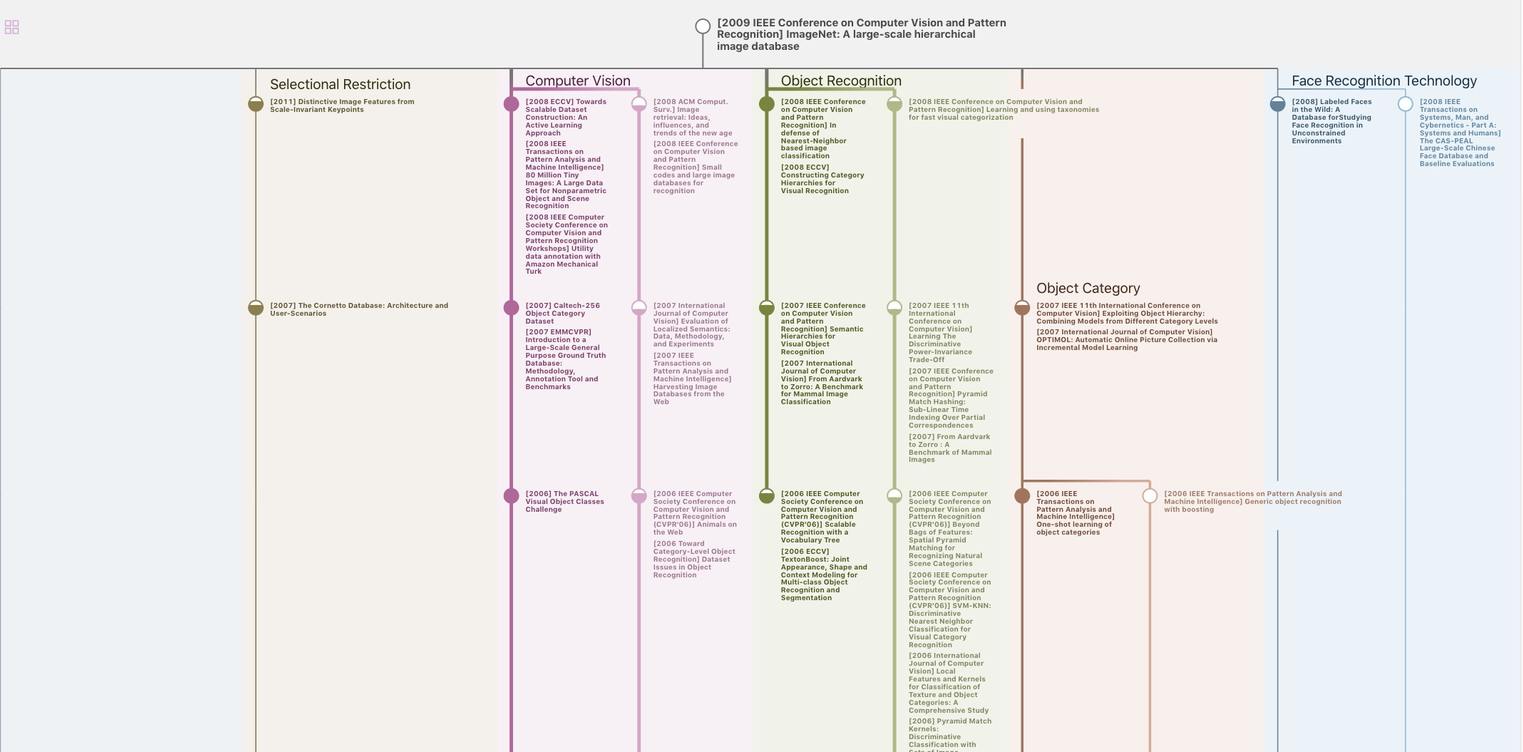
Generate MRT to find the research sequence of this paper
Chat Paper
Summary is being generated by the instructions you defined