A Hybrid Monte Carlo and Deep Learning Approach for Fast and Generic Photon Beam Dose Calculation
arxiv(2024)
Abstract
Dosimetry is an essential tool to provide the best and safest radio-therapies
to a patient. In this field, Monte-Carlo simulations are considered to be the
golden standard for predicting accurately the deposited dose in the body. Such
methods are very time-consuming and simpler dose calculation engines, like dose
kernel approaches, were created for cases where a fast estimation is necessary.
In those approaches, dose distribution maps (or dose kernel) are learned for
simple beams geometry, then, a combination of numerous kernels is used to
simulate more complex beams. However, those methods often lack personalization
and oversimplify the human body, especially for the secondary interactions dose
deposition. In this article, we explore the possibility of learning the dose
kernel using convolutional neural networks to improve their accuracy towards
each different human body. We also highlight the limits of such approaches.
MoreTranslated text
AI Read Science
Must-Reading Tree
Example
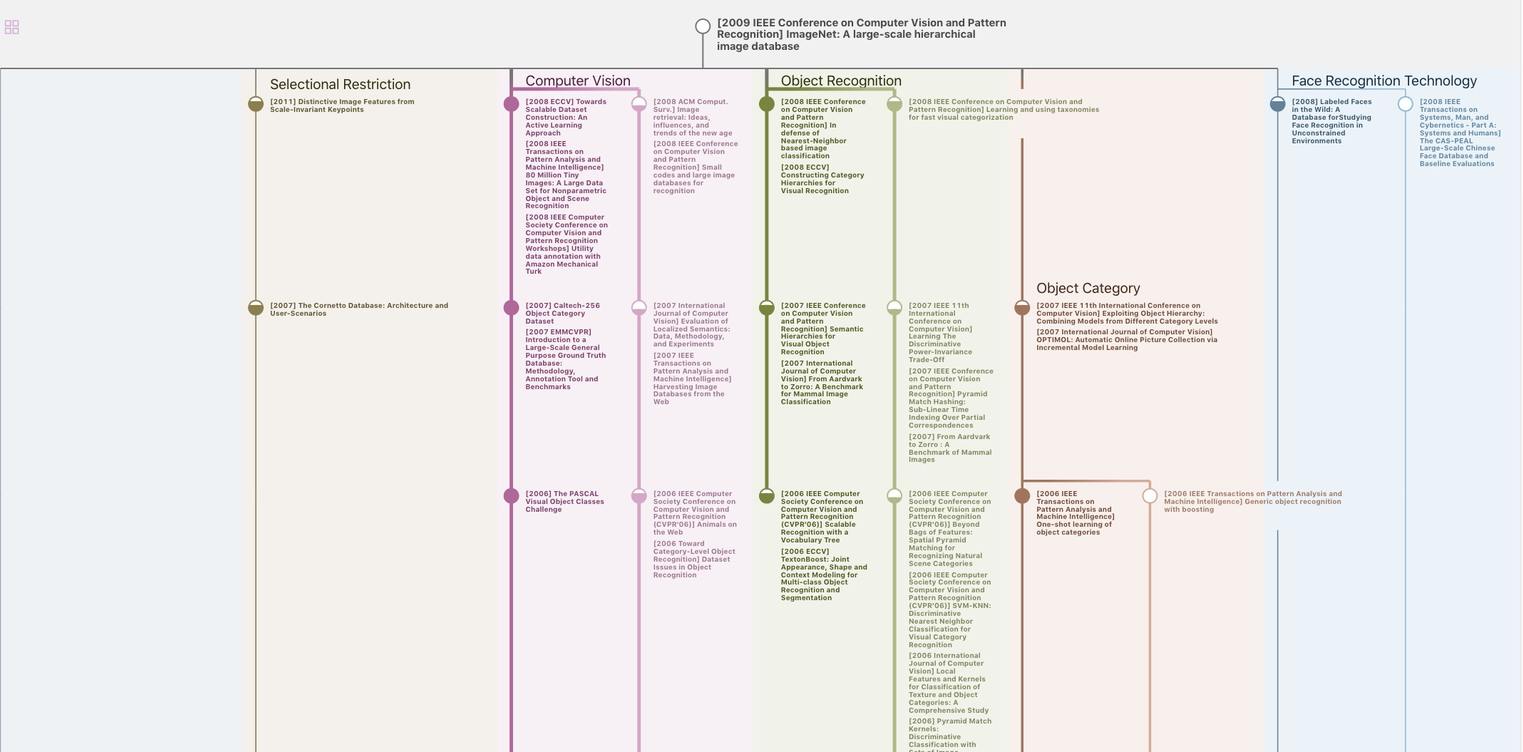
Generate MRT to find the research sequence of this paper
Chat Paper
Summary is being generated by the instructions you defined