Innovative Approaches to Agricultural Sustainability: A Hybrid CNN-SVM Model for Tomato Disease Classification
2024 3rd International Conference for Innovation in Technology (INOCON)(2024)
摘要
A new blend model for accurately classifying tomato illnesses is built and studied in this work. Combining support vector machine (SVM) and convolutional neural network (CNN) methods, the model is made. Some of the more complex tests used in the grading process are accuracy, memory, and F1 scores. These measurements are used to figure out how well the model is working generally. From the data we have, it looks like the model is pretty accurate generally. Its rates run from 94% to 96%. During the whole process, this is always the case. Particularly impressive about the system are its high accuracy in finding positive cases within each disease category and its high memory in gathering a large number of real positive cases. They both show that the model does a great job in both of these areas. The study’s results show that the model can tell the difference between things, which supports the idea that it could be used in agriculture as a safe way to find and treat disease problems early on. Most of the time, the study’s results are good news. If the model’s accuracy, memory, and F1 scores gave correct information that could be used for disease-specific focused treatments, then the model would be more useful in farming settings.
更多查看译文
关键词
Convolutional Neural Network (CNN),Septoria leaf spot (SLS),Support Vector Machine (SVM)
AI 理解论文
溯源树
样例
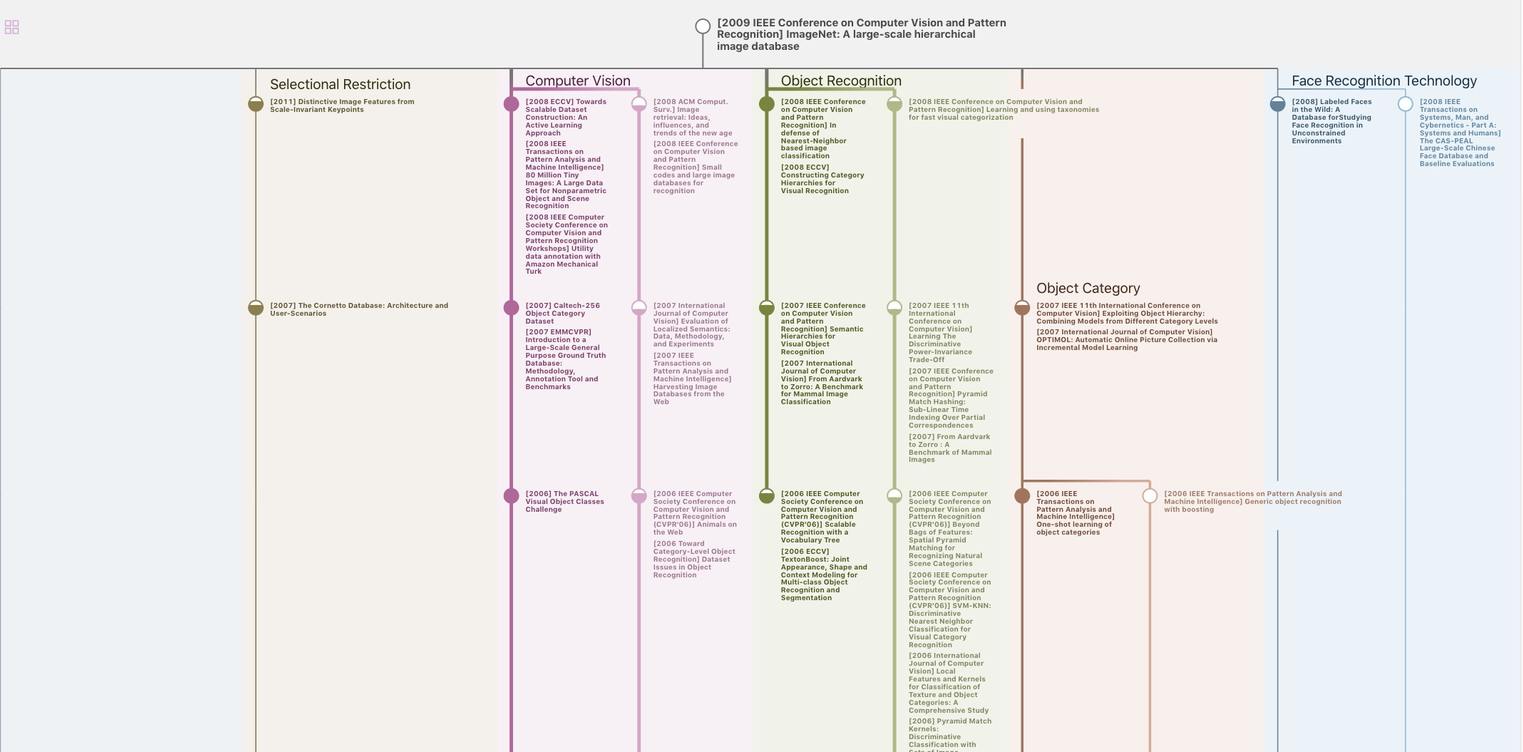
生成溯源树,研究论文发展脉络
Chat Paper
正在生成论文摘要