Multi-Drug Artificial Neural Network Models for Predicting HIV-1 RTI Resistance
biorxiv(2024)
摘要
Drug resistance is a major barrier to effectively treating HIV/AIDS, necessitating the exploration of novel drugs and an understanding of resistance mechanisms. Genotypic and phenotypic tests, while common, are costly and time-consuming. To address these challenges, machine learning models that effectively generalize available data have been leveraged. This study aims to create multi-drug artificial neural network (MD-ANN) models based on drug-isolate-fold change (DIF) to predict the drug resistance profiles of HIV-1 reverse transcriptase inhibitors (RTIs) using the Stanford HIV Drug Resistance Database. Unlike existing isolate-fold change (IF) models, the DIF-based models can test novel RTIs against a given mutant strain. It has been shown that the DIF-based models have competitive predictive capabilities in single-drug benchmarks compared to IF-based models while taking advantage of molecular learning to test novel RTIs. The DIF-based models can rank ten FDA-approved inhibitor pairs according to their drug resistance scores. We validate our observations with an external test set consisting of drug resistance scores for novel RTIs in the CheMBL database. By combining the Stanford dataset and the external dataset, our DIF model achieves impressive classification and regression results with an accuracy of 0.774, AUC of 0.859, and r of 0.701 on the test set. To demonstrate how our DIF models learn from drug molecules, we construct a null model that only takes into account isolate representations. This null model yields an accuracy of 0.641, AUC of 0.701, and r of 0.470. We highlight the significant contribution of drug information in improving the predictive accuracy and reliability of the DIF model. The DIF models have the potential to facilitate the testing of new inhibitors across various isolates.
### Competing Interest Statement
The authors have declared no competing interest.
更多查看译文
AI 理解论文
溯源树
样例
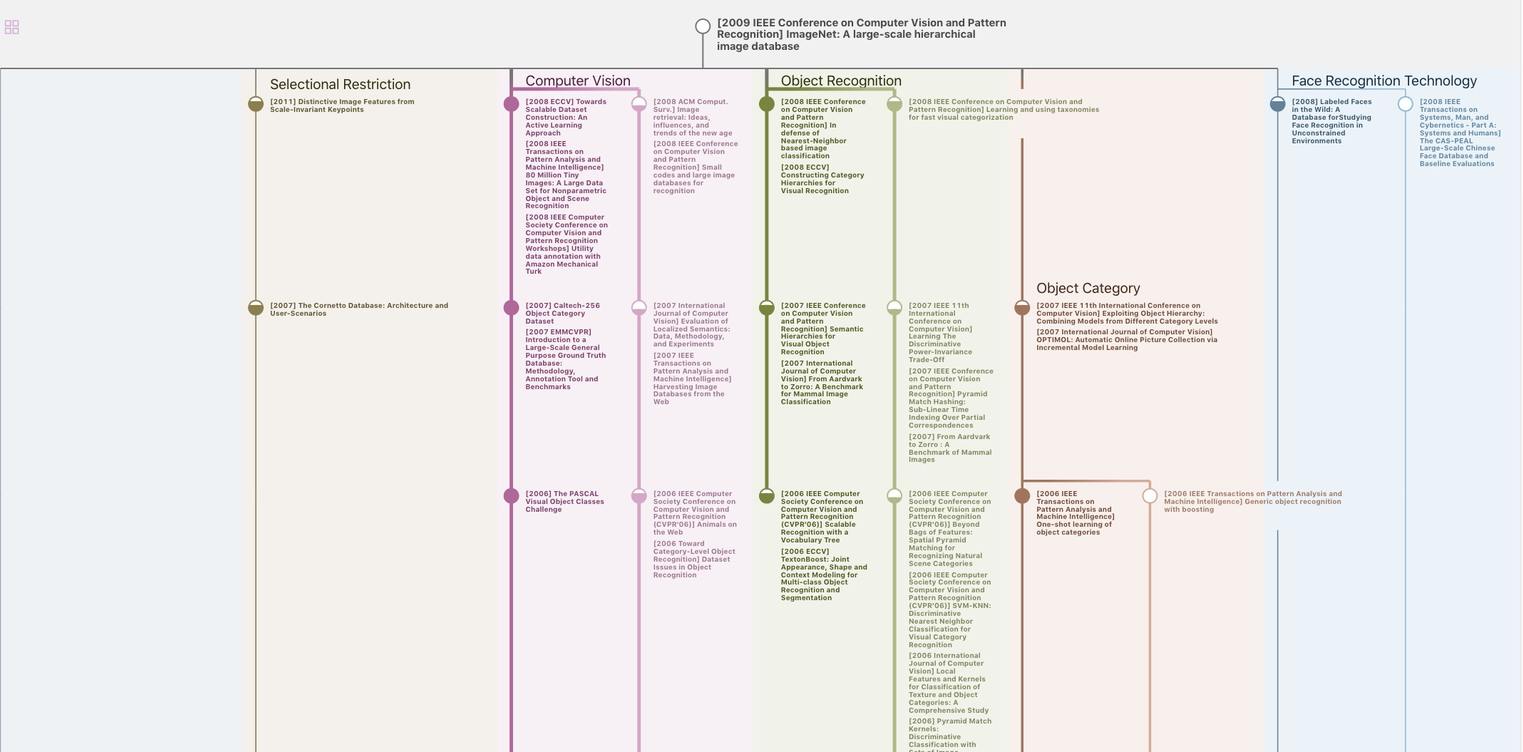
生成溯源树,研究论文发展脉络
Chat Paper
正在生成论文摘要