Intelligent recognition and parameter acquisition of blastholes in rock tunnel based on improved Faster R-CNN
Tunnelling and Underground Space Technology(2024)
摘要
In rock tunnel excavation using the drilling and blasting method, controlling overbreak and underbreak relies heavily on the careful selection and optimization of blasthole parameters, such as number and spacing. To achieve accurate blasting control, it is essential to automatically detect, acquire, and optimize the number and spacing of blastholes on the tunnel face. With the development of image recognition techniques based on convolutional neural networks (CNNs), there are new opportunities for blasthole detection. This study proposes an improved faster region-based CNN (Faster R-CNN) for blasthole detection and automatic acquisition of blasthole number and spacing. The proposed network adopts modular design concepts, including top-down feature fusion, two-stage training, false detection blasthole filtering, and blasthole parameter acquisition. These strategies enable efficient feature extraction, aggregation, filtering, and range finding, thereby significantly enhancing the intelligent acquisition ability of the overall blasthole parameters on the tunnel face. The improved CNNs are validated by an experimental study, and their precision, recall, and F1 score are superior to those of SqueezeNet, VGG-16, and ResNet-50. The distance data from the blasthole image-based detection results can effectively indicate the horizontal and vertical spacings of different types of blastholes on the tunnel face.
更多查看译文
关键词
Tunnel blasting,Blastholes,Faster R-CNN,Object detection,Intelligent parameter acquisition
AI 理解论文
溯源树
样例
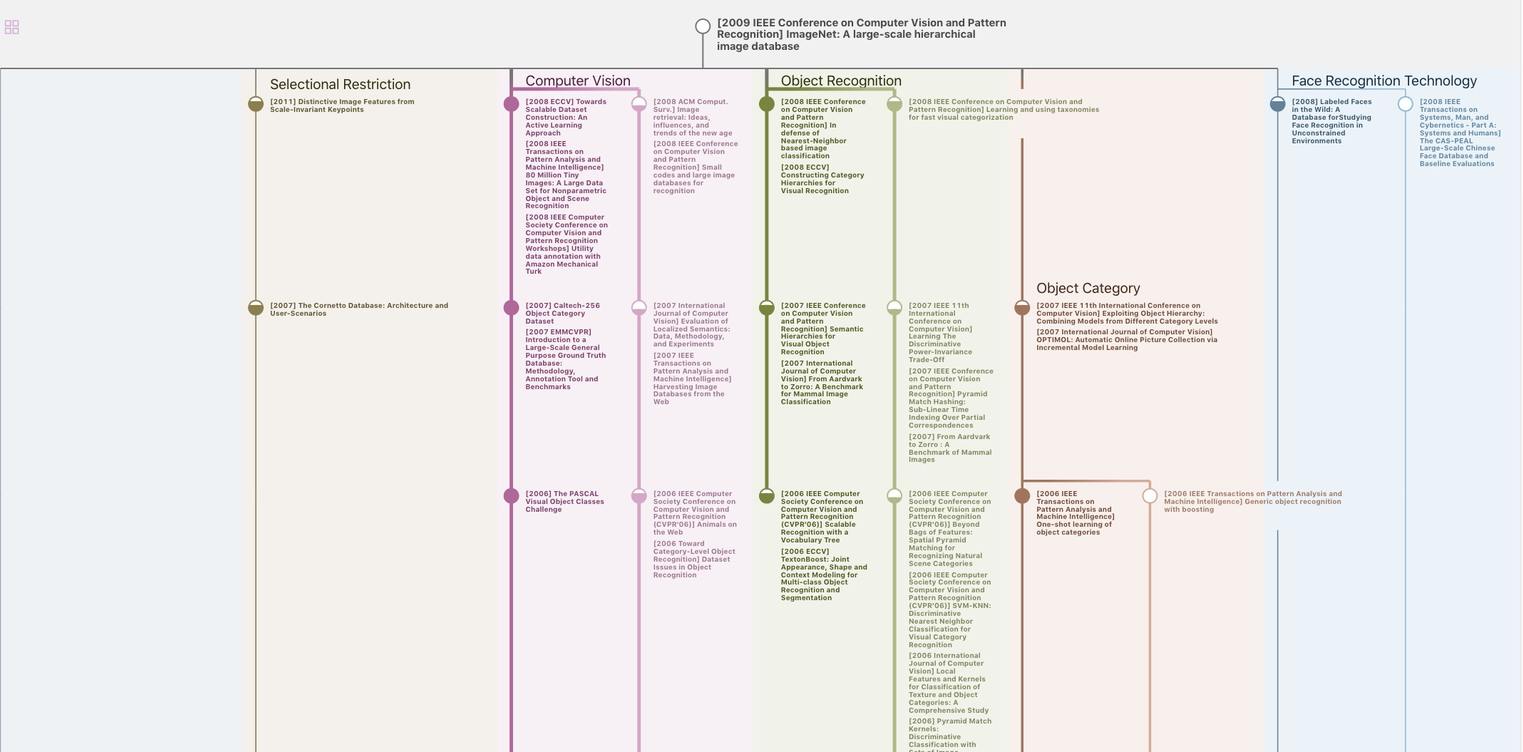
生成溯源树,研究论文发展脉络
Chat Paper
正在生成论文摘要