Learning to compose diversified prompts for image emotion classification
Computational Visual Media(2024)
摘要
Image emotion classification (IEC) aims to extract the abstract emotions evoked in images. Recently, language-supervised methods such as contrastive language-image pretraining (CLIP) have demonstrated superior performance in image understanding. However, the underexplored task of IEC presents three major challenges: a tremendous training objective gap between pretraining and IEC, shared suboptimal prompts, and invariant prompts for all instances. In this study, we propose a general framework that effectively exploits the language-supervised CLIP method for the IEC task. First, a prompt-tuning method that mimics the pretraining objective of CLIP is introduced, to exploit the rich image and text semantics associated with CLIP. Subsequently, instance-specific prompts are automatically composed, conditioning them on the categories and image content of instances, diversifying the prompts, and thus avoiding suboptimal problems. Evaluations on six widely used affective datasets show that the proposed method significantly outperforms state-of-the-art methods (up to 9.29 https://github.com/dsn0w/PT-DPC/for research purposes .
更多查看译文
关键词
image emotion analysis,multimodal learning,pretraining model,prompt tuning
AI 理解论文
溯源树
样例
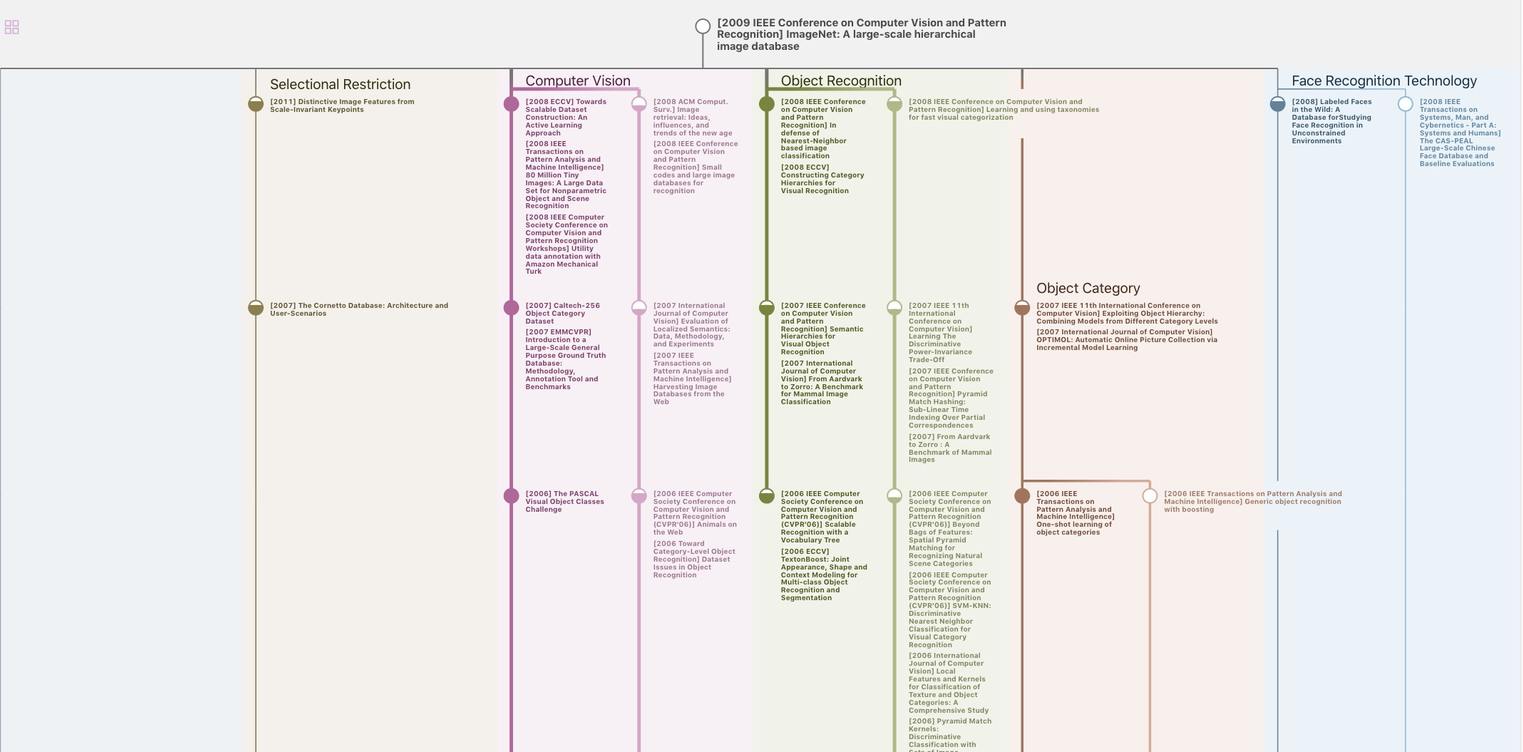
生成溯源树,研究论文发展脉络
Chat Paper
正在生成论文摘要