EDT-STACK: A Stacking Ensemble-Based Decision Trees Algorithm for Tire Tread Depth Condition Classification
Results in Engineering(2024)
摘要
Tires serve as the critical interface between a vehicle and the road. Their condition significantly impacts road safety, vehicle performance, fuel efficiency, and operational costs. Developing a machine learning model for accurately and efficiently classifying tire conditions using tread depth measurements poses a critical challenge in enhancing automotive safety and maintenance. This research aims to address this challenge by leveraging machine learning techniques to categorize and assess tire conditions based on tread depth, thereby contributing to developing a robust and automated system for tire safety condition monitoring in diverse vehicle fleets. This article presents a novel ensemble method-based machine learning algorithm called EDT-STACK (Stacking Ensembles-Based Decision Tree) that combines several decision tree-based methods using a stacking ensemble technique. The model is trained and tested on a dataset of 500 tires (new and disposed) with different tread depths, The model achieves 99% accuracy in all classes, demonstrating its efficacy in classifying tire conditions from tread depth data. The study also compares the performance of EDT-STACK with other algorithms. Its superior performance outshines other decision tree algorithms, such as XGBoost, AdaBoost, Random Forest, ID3, and Histogram-based Decision Tree, which achieve accuracies of 98%, 78%, 85%, 98%, and 98%, respectively. This study concludes that EDT-STACK offers an innovative solution for tire condition assessment, which can enhance road safety, reduce environmental impacts, and optimize vehicle maintenance.
更多查看译文
关键词
tire,vehicle,ensemble,xgboost,adaboost,id3,HBDT,classification
AI 理解论文
溯源树
样例
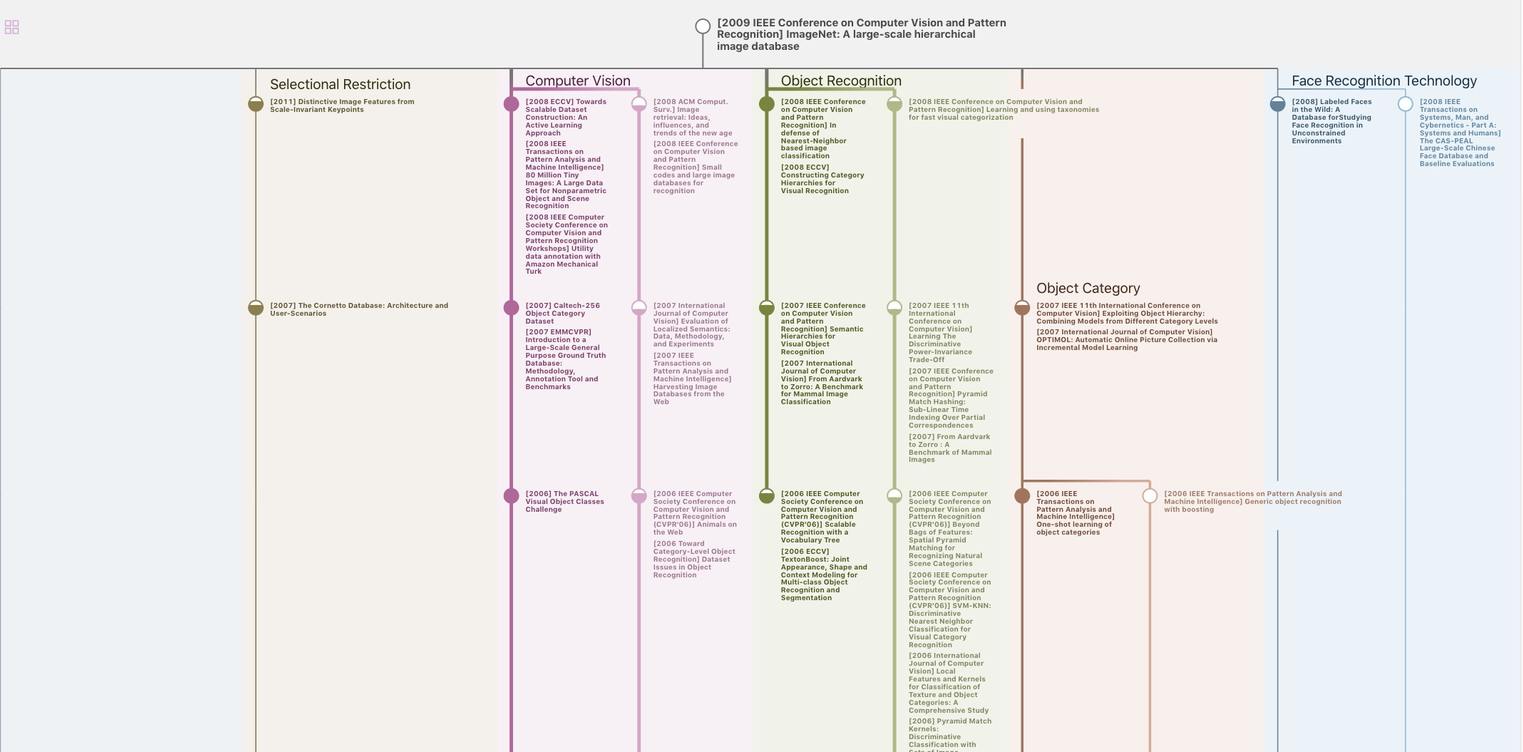
生成溯源树,研究论文发展脉络
Chat Paper
正在生成论文摘要