Filter channel network based on contextual position weight for aspect-based sentiment classification
The Journal of Supercomputing(2024)
Abstract
In recent years, sentiment analysis in the field of natural language processing has garnered increasing attention from researchers. Aspect-level sentiment classification is a fine-grained task aiming to discern the sentiment polarity of specific aspects within sentences. Currently, many methods rely on extracting keyword information from context to judge sentiment polarity, yielding promising results. However, most of these methods overlook the impact of the position of context words on sentiment polarity. To address this issue, we propose a novel aspect-level sentiment analysis method that integrates context position weights and filtering channels (CPW-FC). In our model, an asymmetric position weight function, denoted as α function, is devised to ensure sentiment preservation while mitigating the influence of aspect words not positioned in the sentence’s middle on sentiment polarity judgment. Additionally, we introduce a filtering channel to diminish the influence of contextually irrelevant words on sentiment polarity. Extensive experiments are conducted on four publicly available datasets: Restaurant, Laptop, Twitter, and NAMS. Our model achieves accuracy of 86.33, 81.45, 77.10, and 84.81 https://github.com/ZCMR/alpha .
MoreTranslated text
Key words
Aspect-based sentiment classification,Natural language processing,Position weight,Filter channel
AI Read Science
Must-Reading Tree
Example
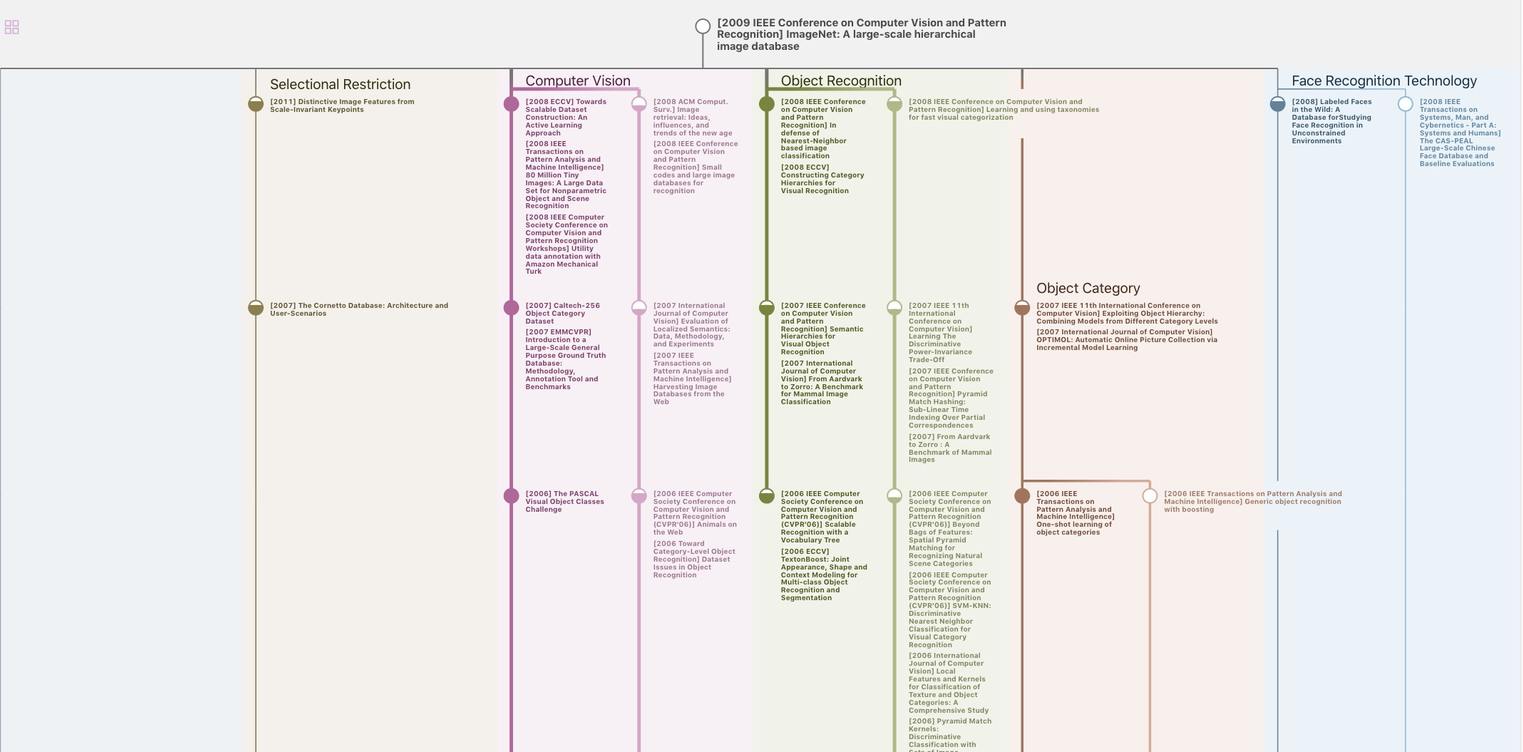
Generate MRT to find the research sequence of this paper
Chat Paper
Summary is being generated by the instructions you defined