From data to discovery: AI-guided analysis of disease-relevant molecules in spinal muscular atrophy (SMA)
HUMAN MOLECULAR GENETICS(2024)
摘要
Spinal Muscular Atrophy is caused by partial loss of survival of motoneuron (SMN) protein expression. The numerous interaction partners and mechanisms influenced by SMN loss result in a complex disease. Current treatments restore SMN protein levels to a certain extent, but do not cure all symptoms. The prolonged survival of patients creates an increasing need for a better understanding of SMA. Although many SMN-protein interactions, dysregulated pathways, and organ phenotypes are known, the connections among them remain largely unexplored. Monogenic diseases are ideal examples for the exploration of cause-and-effect relationships to create a network describing the disease-context. Machine learning tools can utilize such knowledge to analyze similarities between disease-relevant molecules and molecules not described in the disease so far. We used an artificial intelligence-based algorithm to predict new genes of interest. The transcriptional regulation of 8 out of 13 molecules selected from the predicted set were successfully validated in an SMA mouse model. This bioinformatic approach, using the given experimental knowledge for relevance predictions, enhances efficient targeted research in SMA and potentially in other disease settings.
更多查看译文
关键词
spinal muscular atrophy,SMA,network biology,artificial intelligence,motoneuron disease
AI 理解论文
溯源树
样例
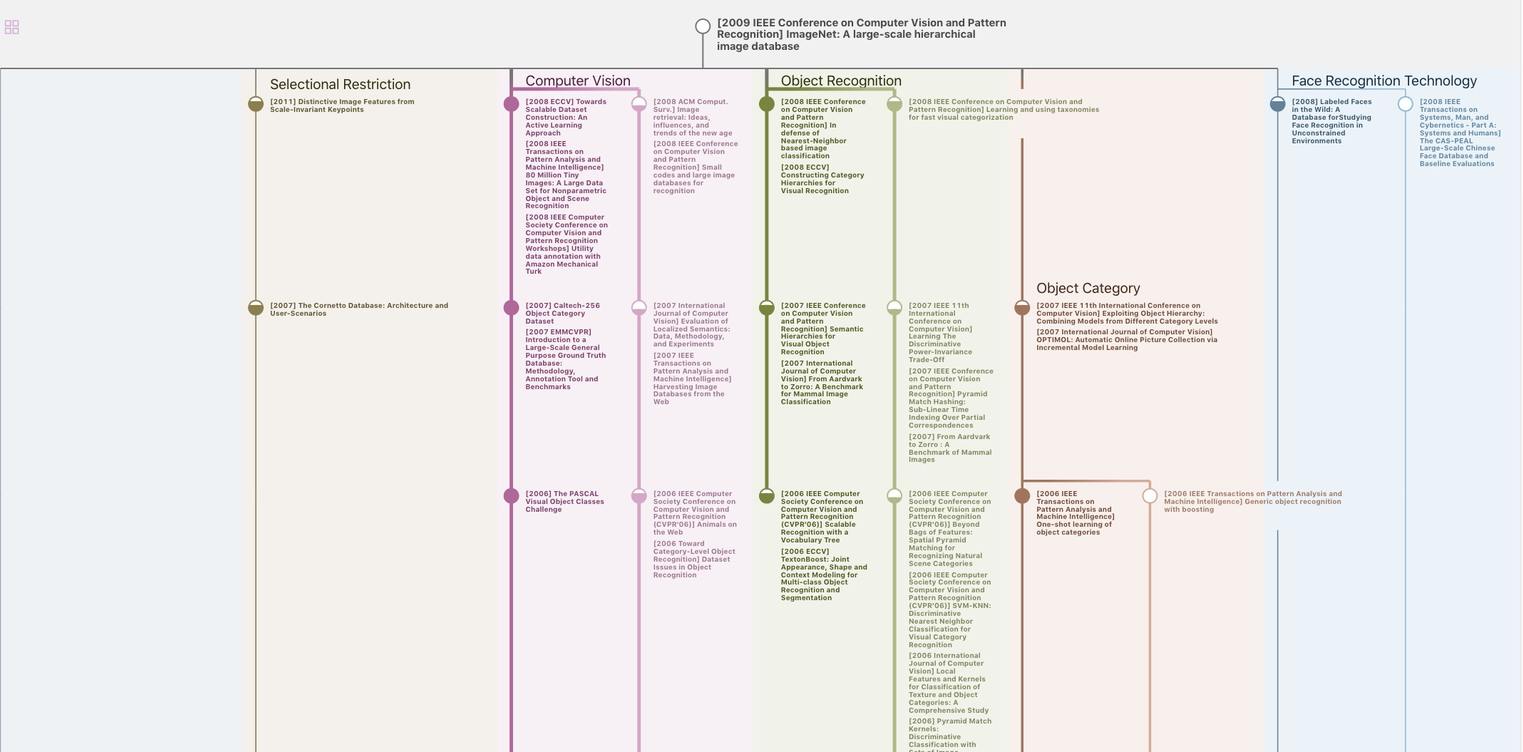
生成溯源树,研究论文发展脉络
Chat Paper
正在生成论文摘要