Structural and Textural-Aware Feature Extraction for Hyperspectral Image Classification
IEEE GEOSCIENCE AND REMOTE SENSING LETTERS(2024)
摘要
Feature extraction is a prevalent technique in hyperspectral remote sensing. Various tasks require this technique as a preprocessing step, including image classification, anomaly detection, image denoising, and so on. Edge-preserving filtering-based methods have been extensively utilized for this purpose. However, these methods do not take the inherent structural and textural information into account, leading to poor performance in classifying hyperspectral images (HSIs). In this letter, a new structural and textural-aware feature extraction method is proposed that preserves the relevant structural information and removes useless textures. First, structural and textural-aware recursive filtering features (STRFs) are extracted along with an exponential form of windowed inherent variance (eWIV). Then, multiscale STRFs are integrated by the principal component analysis (PCA) method to obtain more discriminative features (MSTRF). Finally, the fused features are fed into a pixel-wise classifier to obtain the final results. The main difference between the MSTRF method and other feature extraction methods is that the MSTRF method can make full use of the proposed eWIV map, which can help to properly characterize structure and texture in HSIs. Experimental results on several public datasets indicate that our method leads to state-of-the-art classification performance, especially in the presence of very small training set.
更多查看译文
关键词
Feature extraction,Filtering,Image edge detection,Principal component analysis,Hyperspectral imaging,Electronic mail,Support vector machines,Edge-preserving filtering,hyperspectral image (HSI) classification,structural and textural-aware feature extraction,windowed inherent variance (WIV)
AI 理解论文
溯源树
样例
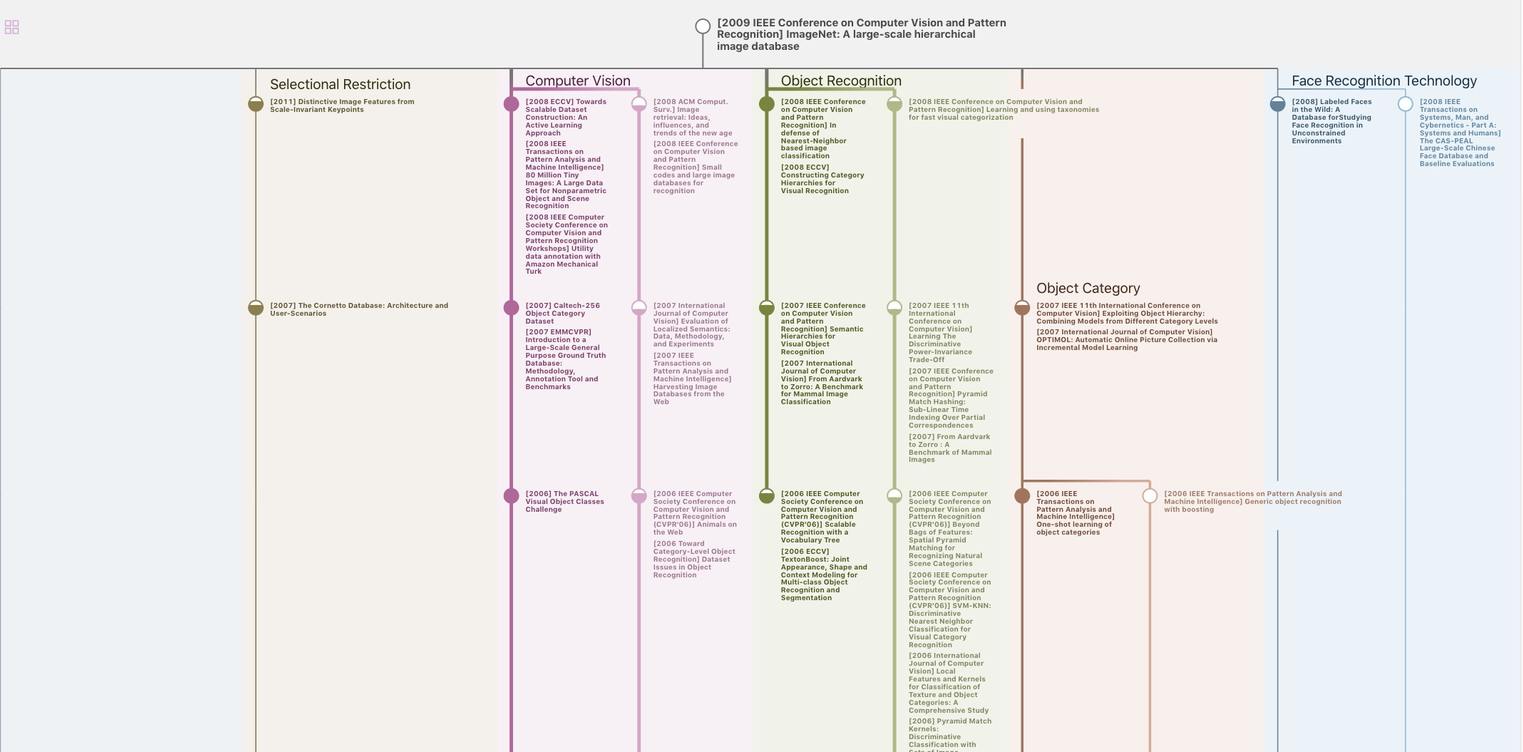
生成溯源树,研究论文发展脉络
Chat Paper
正在生成论文摘要