Adaptive deep homogenization theory for periodic heterogeneous materials
Composite Structures(2024)
摘要
We present an adaptive physics-informed deep homogenization neural network (DHN) approach to formulate a full-field micromechanics model for elastic and thermoelastic periodic arrays with different microstructures. The unit cell solution is approximated by fully connected multilayers via minimizing a loss function formulated in terms of the sum of residuals from the stress equilibrium and heat conduction partial differential equations (PDEs), together with interfacial traction-free or adiabatic boundary conditions. In comparison, periodicity boundary conditions are directly satisfied by introducing a network layer with sinusoidal functions. Fully trainable weights are applied on all collocation points, which are simultaneously trained alongside the network weights. Hence, the network automatically assigns higher weights to the collocation points in the vicinity of the interface (particularly challenging regions of the unit cell solution) in the loss function. This compels the neural networks to enhance their performance at these specific points. The accuracy of adaptive DHN is verified against the finite element and the elasticity solution respectively for elliptical and circular cylindrical pores/fibers. The advantage of the adaptive DHN over the original DHN technique is justified by considering locally irregular porous architecture where pore–pore interaction makes training the network particularly slow and hard to optimize.
更多查看译文
关键词
Physics-informed machine learning,Full-field homogenization,Composites,Periodic arrays,Elasticity
AI 理解论文
溯源树
样例
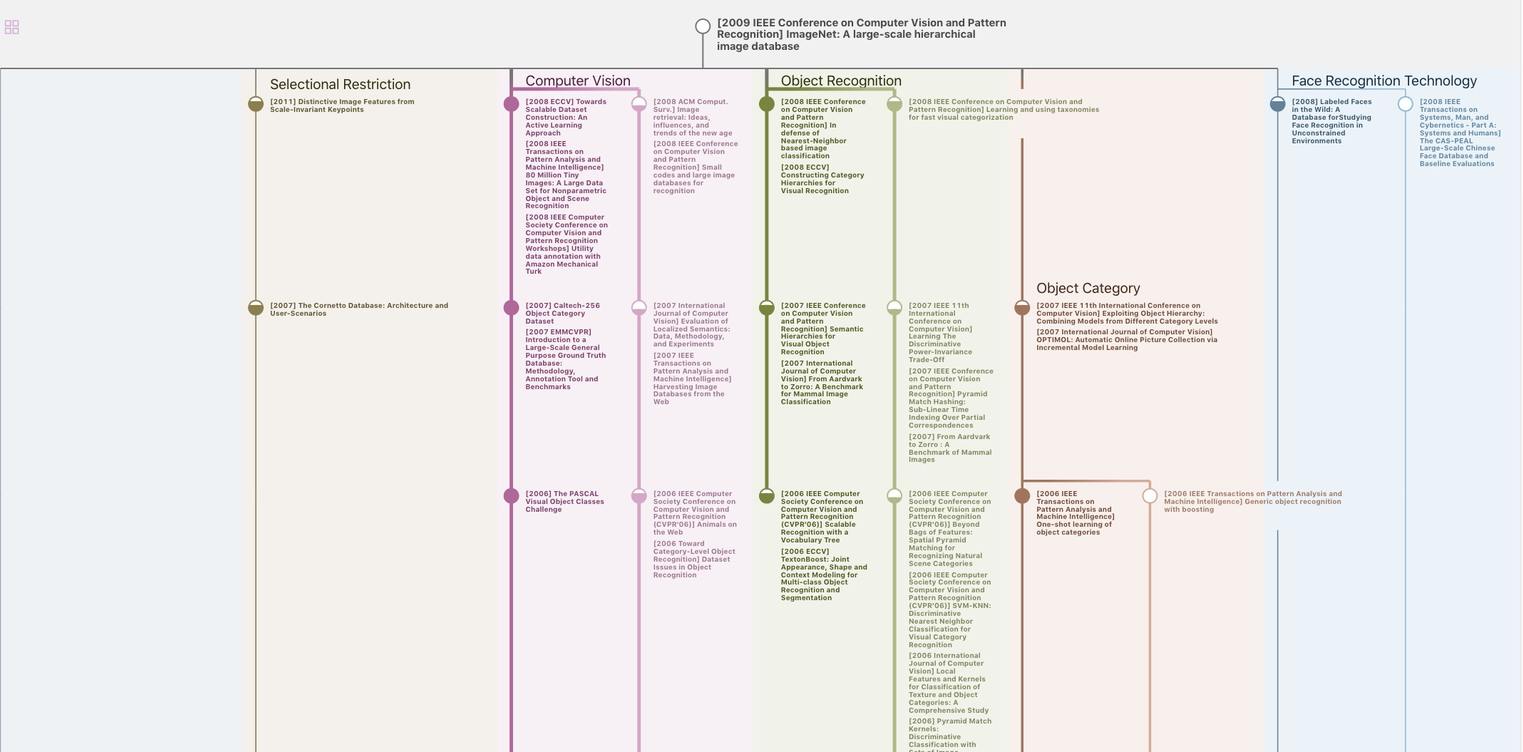
生成溯源树,研究论文发展脉络
Chat Paper
正在生成论文摘要