Deep Active Learning for mmWave Array-Based Multi-Source AoA Tracking
IEEE Transactions on Wireless Communications(2024)
摘要
In this paper, we investigate the problem of tracking the angles of arrival (AoAs) of multiple sources in millimeter wave (mmWave) systems with a limited number of radio frequency (RF) chains. Considering the time-varying nature of the channel, we propose a deep neural network (DNN)-based active learning scheme for adaptive analog beamforming and multi-source AoA tracking. The proposed scheme consists of a DNN-based beamformer and a subspace tracking-based multiple signal classification (MUSIC) estimator. Specifically, the DNN generates the beamformer using soft AoA estimates from the previous time block, and the MUSIC estimator exploits the measured signal by the beamformer to estimate the AoAs in the current time block. The proposed scheme is first applied to the uniform linear array (ULA) scenario, and then extended to the uniform rectangular array (URA) scenario. Particularly, in the URA scenario, to reduce the computational complexity, we modify the reduced-dimension MUSIC (RD-MUSIC) algorithm to a beam-space form. Furthermore, we adopt a partially connected analog beamforming scheme for the large-scale URA scenario to further reduce the hardware costs. We conduct numerical experiments to evaluate the tracking performance of the proposed scheme in the ULA and URA scenarios, and show that the proposed scheme significantly outperforms the existing codebook-based beamformer methods.
更多查看译文
关键词
Multi-source AoA tracking,adaptive beamforming,deep active learning,subspace method,array signal processing
AI 理解论文
溯源树
样例
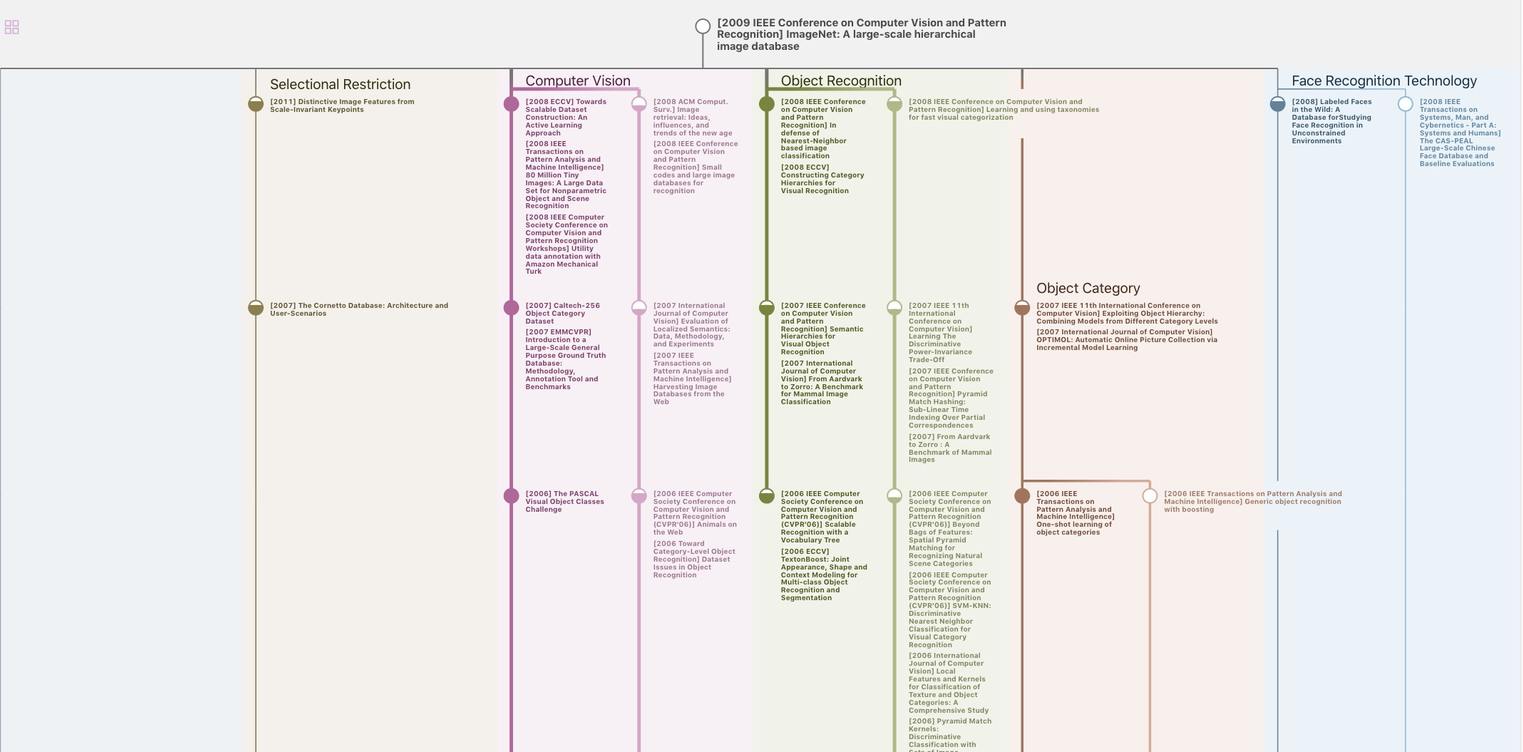
生成溯源树,研究论文发展脉络
Chat Paper
正在生成论文摘要