Automated Design of Fault Diagnosis CNN Network for Satellite Attitude Control Systems.
IEEE transactions on cybernetics(2024)
摘要
Despite the dominance of unsupervised and self-supervised anomaly detection methods in the current satellite fault diagnosis domain, supervised anomaly detection offers a superior alternative for high-sensitivity detection and lightweight deployment requirements specific to subsystems or components, such as attitude control systems (ACSs). This article addresses the issues of over-design and insufficient accuracy in the CNN network design for satellite ACS fault diagnosis by introducing the modified particle swarm optimization-advanced convolution blocks-based CNN (MPSO-ACBCNN) method. First, we present the ACBCNN, a lightweight, flexible-layer CNN architecture. This architecture leverages advanced convolution blocks (ACBs), which incorporate numerous efficient design elements to enhance feature extraction capabilities within power spectral density (PSD) graphs of various fault samples, and employs classical dense connection methods to prevent the issue of gradient vanishing. Second, we devise the MPSO-ACBCNN algorithm to optimize the ACBCNN fault diagnosis architecture for specified ACS using MPSO. In MPSO-ACBCNN, several optimizations to the canonical PSO are implemented, including the fitness design that balances the tradeoff between total parameter quantity and the training effectiveness, and methods to ensure feasible solutions, etc. Finally, numerical experimental results demonstrate the effectiveness and superiority of MPSO-ACBCNN in fault diagnosis for ACS.
更多查看译文
关键词
Fault diagnosis,network architecture optimization,satellite attitude control systems (ACSs)
AI 理解论文
溯源树
样例
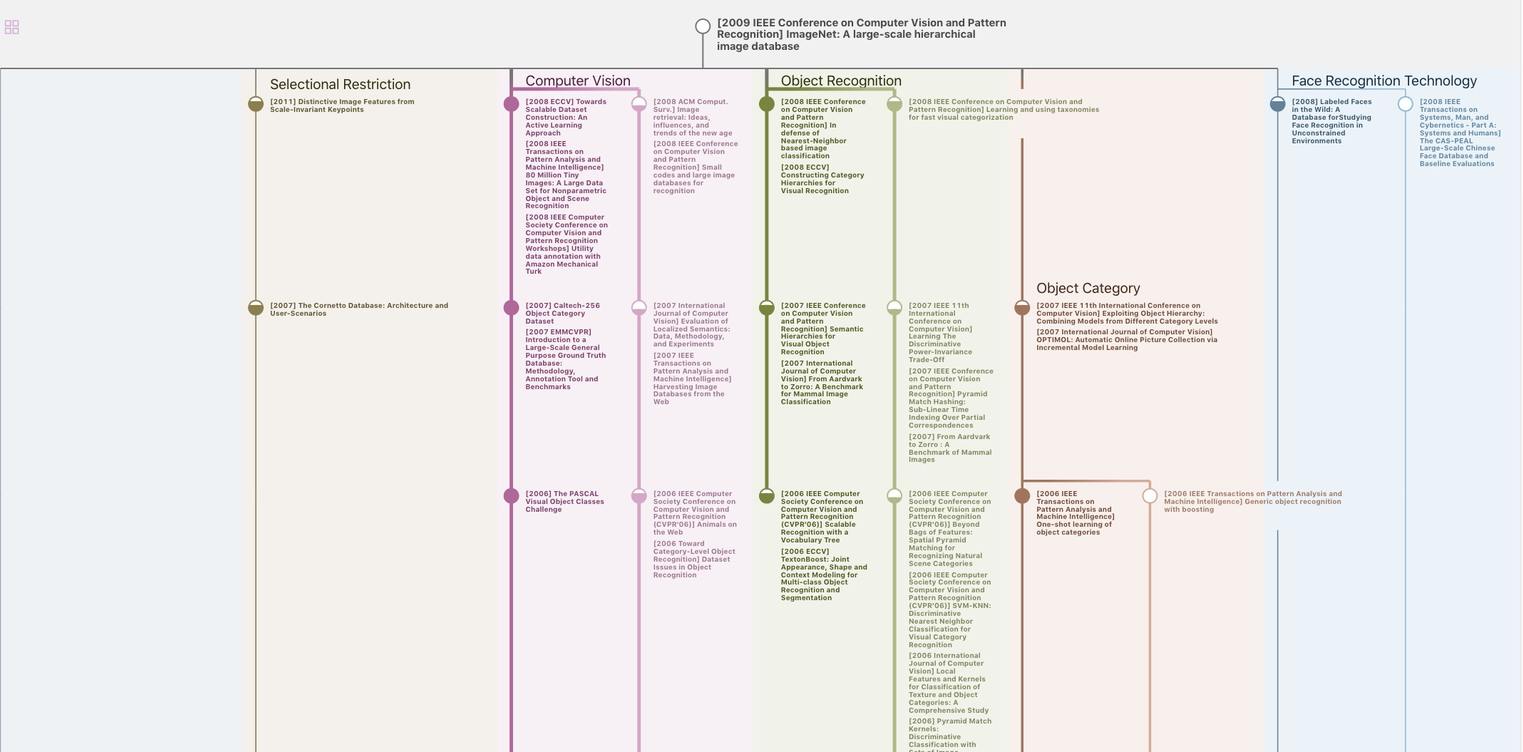
生成溯源树,研究论文发展脉络
Chat Paper
正在生成论文摘要