Task-related network based on meta-learning for few-shot knowledge graph completion
Applied Intelligence(2024)
Abstract
Knowledge graph (KG) is a powerful tool in many areas, but it is impossible to take in all knowledge during construction for the complexity of relations among natural entities. In practical terms, many relations within KG have few samples to learn from, so few-shot KG completion matters. Existing approaches generally complete KGs by learning either static representations of entities and relations or the dynamic representations of fixed model parameters, while ignoring the importance of dynamic fine-tuning of model parameters for rapidly mining task-related information. In this paper, we propose a novel task-related network based on meta-learning (MTRN) for few-shot knowledge graph completion. Firstly, a task-related neighbor-aware encoder, which can distinguish the contributions of different neighbors, is designed to mine the task-related information of neighbors from the heterogeneous background graph and to enhance task entity representations. Secondly, a self-attention entity-pair encoder is proposed to generate the representations of references by modeling the interaction of head and tail entities. Then MTRN models references, that is entity pairs in the reference set, through a matching network to differentiate the contributions of different entity pairs to task relations and obtain the task-related representations with fine-grained semantics. When performing different knowledge graph completion tasks, MTRN can extract the features of few-shot references in a meta-learning way to dynamically fine-tune model parameters, making MTRN quickly adapt to diverse tasks in few-shot setting. The evaluation on two public datasets of knowledge graph completion shows that MTRN achieves competitive completion performance under different few-shot sizes.
MoreTranslated text
Key words
Dynamic fine-tuning,Knowledge graph completion,Meta-learning,Task-related representation learning
AI Read Science
Must-Reading Tree
Example
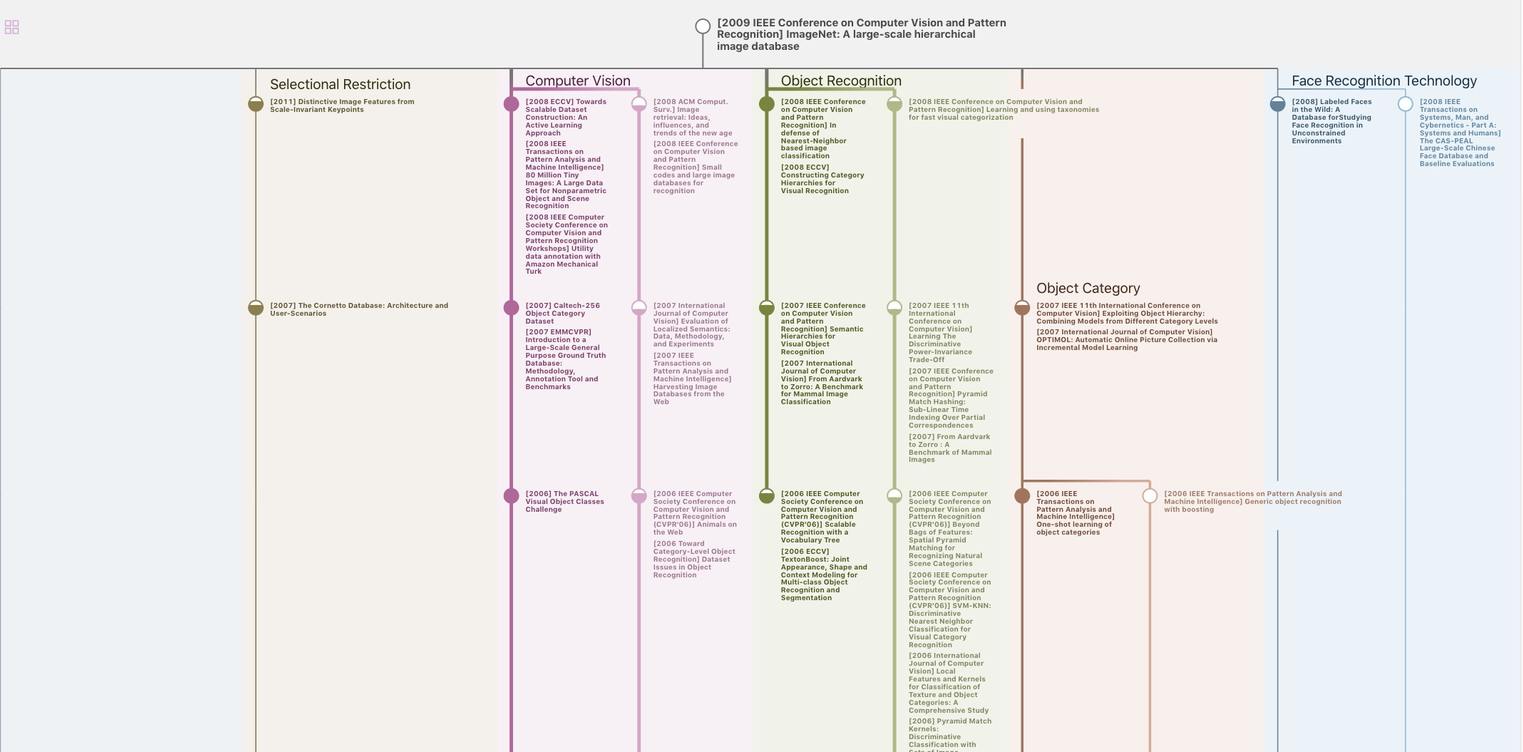
Generate MRT to find the research sequence of this paper
Chat Paper
Summary is being generated by the instructions you defined