Neural Network and Random Forest Algorithms as Catalysts in QSAR/QSAAR Modeling: Targeting β-Carbonic Anhydrase for Antituberculosis Drug Design
biorxiv(2024)
Abstract
Mycobacterium tuberculosis beta-carbonic anhydrases ( MtbCAs ) are metalloenzymes responsible for catalyzing the conversion of CO2 to HCO3- by hydration. The pH regulation of Mycobacterium is considered crucial for Mtb survival in acidic environments. Through the inhibition of MtbCAs , we can identify novel targets for antituberculosis medications that operate differently from currently approved treatments. In the present study, we developed a novel cheminformatics pipeline by generating two diverse molecular feature-based machine learning-assisted quantitative structural activity relationship (ML-QSAR) models concerning the individual inhibition mechanisms of MtbCA1 and MtbCA2 . Random forest algorithm-based ML-QSAR prediction models were implemented using a combination of molecular fingerprints and descriptors to investigate the individual chemical spaces of inhibitors for each MtbCA subtype. The final ML-QSAR predictive models were further developed as a web application, MtbCA-Selec-Pred ( ), to allow users to predict the bioactivity of molecules against MtbCA1 and MtbCA2 . Additionally, the molecular signatures of MtbCA1 MtbCA2 dual inhibitors were investigated using a neural network-based machine learning-assisted quantitative structural activity-activity relationship (ML-QSAAR) model. We concluded that molecules with conserved molecular signatures, SubFP1, SubFP179, SubFP287, SubFP143, SubFP100, nHBint8, SHBint8, naasC, and SHssNH, selectively inhibited MtbCA1 . In contrast, the molecules with conserved molecular signatures SubFP275, SubFP28, SubFP1, SubFP183, SubFP184, minHBa, nHeteroRing, and n5Ring selectively inhibited MtbCA2 . The presented models have the potential to serve as tools for identifying crucial molecular characteristics in the rational design of MtbCA inhibitors and might be employed for developing effective drugs against tuberculosis.
### Competing Interest Statement
The authors have declared no competing interest.
MoreTranslated text
AI Read Science
Must-Reading Tree
Example
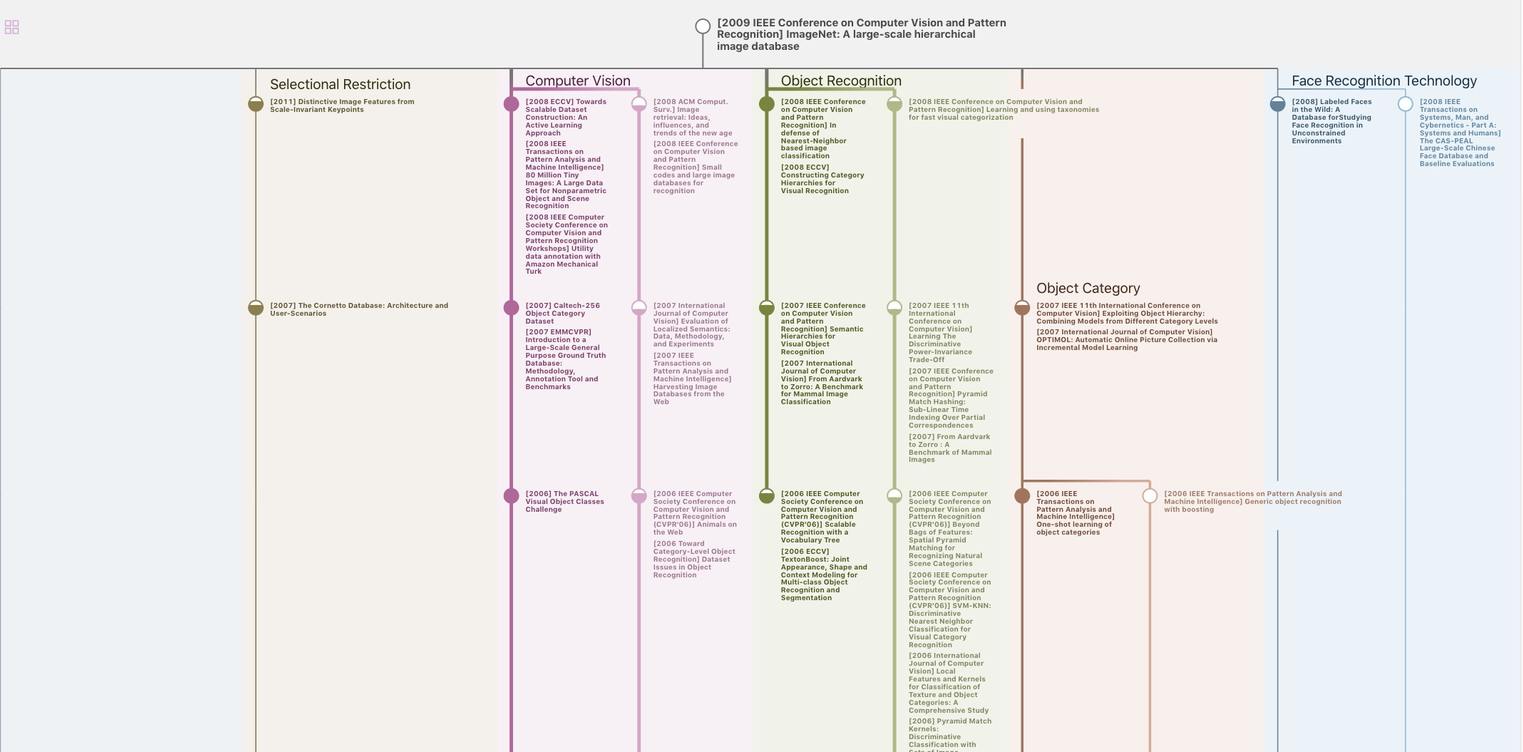
Generate MRT to find the research sequence of this paper
Chat Paper
Summary is being generated by the instructions you defined